Covariance between similarly tuned populations in human visual cortex is model-dependent
Journal of Vision(2023)
摘要
Signals from different neural populations tend to covary as a function of tuning similarity. This covariance can be explained by shared stimulus-dependencies, neural factors like common inputs or connectivity, and measurement factors such as shared vasculature in fMRI. Here, we measure population receptive fields (pRF) in human visual cortex using fMRI with standard protocols and find that a significant portion of tuning-dependent covariance between neural populations in V1 and V3 can be explained by failure to subtract the same part of the stimulus-driven signal in both populations. We measured BOLD responses (13 subjects) at 3T to multiple (4-8) identical 4-minute runs of high-contrast drifting-bar stimuli and fit the responses with gaussian pRFs for each voxel. Tuning similarity between well-fit voxels (r-squared > 0.60, eccentricity < 30º) was calculated as normalized pRF overlap and covariance as the correlation between residual time series (measured - model prediction). Similar to previous literature, residual correlations between voxels in V1 and V3 increased with pRF overlap. We fit exponential functions to quantify this relationship; within subjects, all exponents were significantly greater than expected by chance (p < 0.001, permutation test; exponent = 1.4128 ± 0.6356 STD across subjects). This relationship persisted between separate runs of the same stimulus (p < 0.001, permutation tests; exponent = 1.5695 ± 0.6576 STD across subjects), suggesting that residual correlations arise from stimulus-driven responses not fully accounted for by the pRF model. As a parameter-free estimate of the stimulus dependency, we used the average of the responses to half the runs of the same stimulus as a model prediction for the other half. This parameter-free approach reduced correlations as a function of pRF overlap (p < 0.001, paired t-test; exponent = 0.6056 ± 0.2863 STD between subjects). Thus, imperfect encoding models contribute significantly to covariance between similarly tuned voxels.
更多查看译文
关键词
human visual cortex,populations,model-dependent
AI 理解论文
溯源树
样例
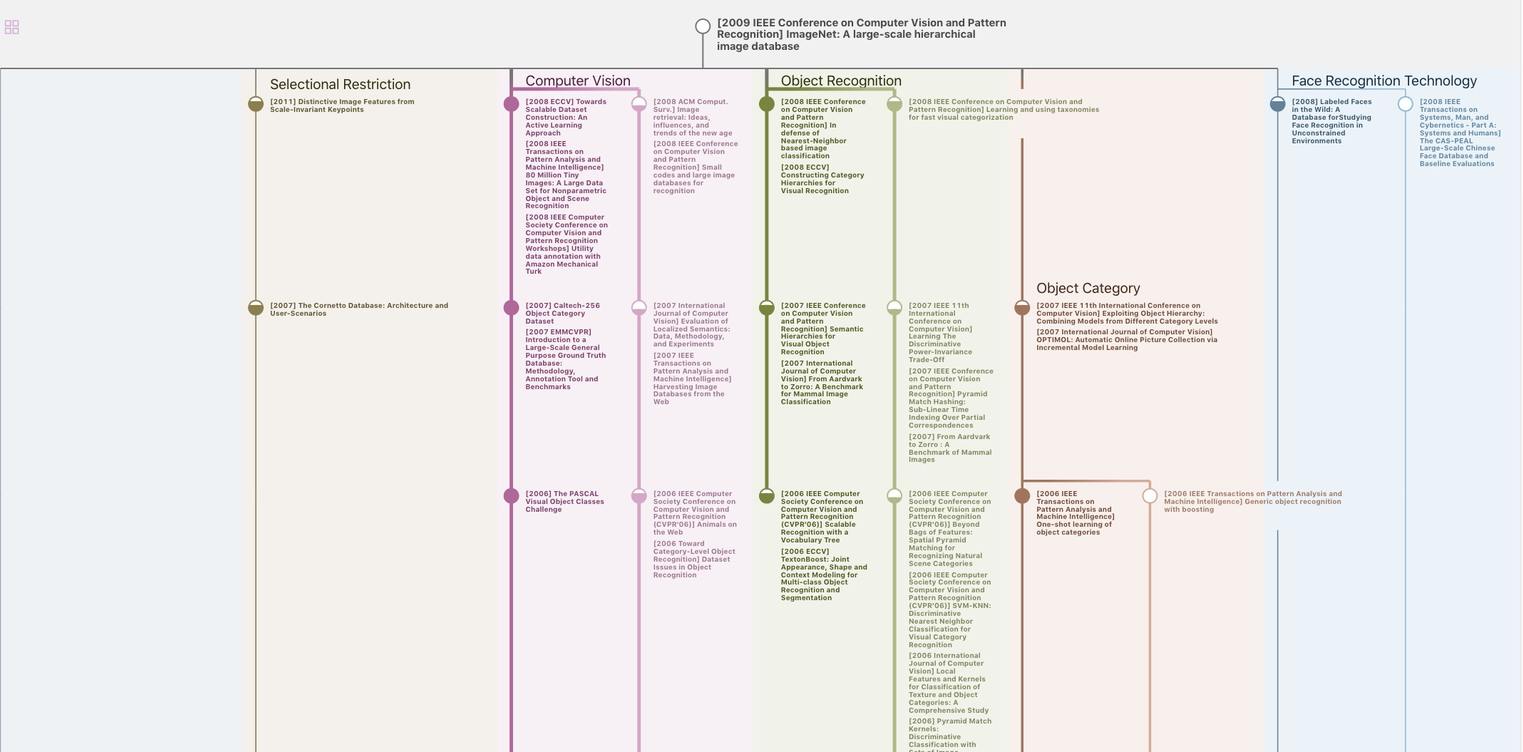
生成溯源树,研究论文发展脉络
Chat Paper
正在生成论文摘要