Pb2704: predicting hematological malignancies using complete blood cell counts from primary care
HemaSphere(2023)
摘要
Topic: 36. Ethics and health economics Background: Hematological malignancies are rare and primary care physicians often only encounter a few cases throughout their career. As optimal referral to secondary care often relies on a combination of pattern recognition and fulfilling referral criteria, it may be challenging for non-hematologists to stay updated and able to recognize hematological cancers in early stages. Aims: To create an algorithm capable of screening complete blood cell counts for impending hematological malignancy using machine learning. Methods: We included adult patients with no history of hematological malignancy, and at least one complete blood cell count (CBC) in the Copenhagen Primary Care Laboratory (CopLab) Database encompassing all laboratory results from the primary care sector of the greater Copenhagen area (20 % of the Danish population) from 2000-2016. Hematological malignancies were identified through the Danish Cancer Register. The CBC furthest from but within 6, 12 and 24 months of a cancer event were included and matched to up to 5 non-cancer events (death, emigration or nothing), for a person with the same sex and within 4 years of age of the cancer patient. Up to 5 years of CBC history was included. The data was split 60:40 into training and validation sets based on a unique personal identifier (CPR-number). The model was trained using SuperLearner; fitting and pre-validating a library of 4 methods, with or without the 5-year CBC history. The optimal ensemble was then estimated using LASSO. Receiver Operating Characteristic (ROC) curves were estimated to assess the algorithms’ discriminatory value. Decision curves were then estimated to assess the net benefit (true positive fraction – false positive fraction x (p/(1-p)) as a function of the threshold probability p. Results: 663,184 patients were included. Areas under the ROC curves (AUC) for malignancy within 6, 12 and 24 months were 0.84, 0.81 and 0.74, respectively. If the 5-year CBC history was included, the AUCs increased to 0.85 (6 mo.), 0.81 (12 mo.) and 0.75 (24 mo.), respectively. The decision curve analysis indicated a positive net benefit of the algorithm over a wide range of threshold probabilities, and clearly indicates superiority to the WHO criteria for referral. Summary/Conclusion: Risk prediction of any hematologic malignancy within 6 months can be done with high accuracy based on current CBC with no marked benefits of including a 5-year CBC history. Accuracy declines slightly when looking at 1 year and 3 years. Risk prediction models are well-calibrated in this selected subsample, appearing to out-perform the current WHO referral criteria in this setting. Keywords: Prediction, Machine learning
更多查看译文
关键词
hematological malignancies,complete blood cell counts,pb2704
AI 理解论文
溯源树
样例
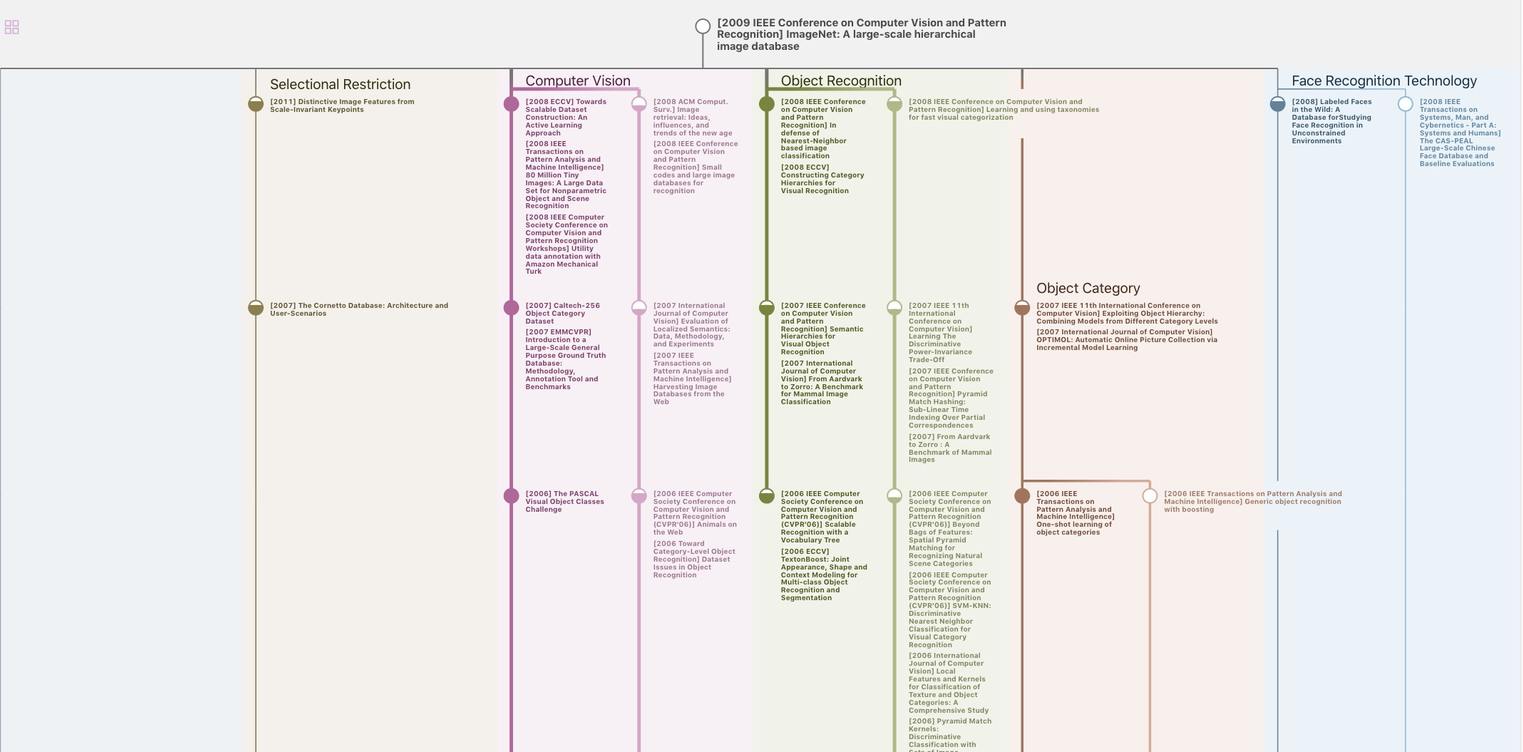
生成溯源树,研究论文发展脉络
Chat Paper
正在生成论文摘要