Robust Geometry-Dependent Attack for 3D Point Clouds.
IEEE Trans. Multim.(2024)
摘要
Deep learning models for point clouds have shown to be vulnerable to adversarial attacks, which have received increasing attention in various safety-critical applications such as autonomous driving, robotics, and surveillance. Since existing 3D attack methods either modify the local points or perform global point-wise perturbations over the point cloud, they fail to capture the dependency between neighboring points for preserving the geometrical context and topological smoothness of the original 3D object. In this paper, we propose a novel Geometry-Dependent Attack (GDA), which aims to generate more robust adversarial point clouds with lower perturbation costs by capturing and preserving the geometry-guided topology information. Specifically, we first analyze the geometric information of each benign point cloud following the graph signal processing and disentangle it into low-frequency (flat) and high-frequency (contour) components. Then, considering the varying characteristics of smoothness and sharpness after disentanglement, we design two collaborative patch-aware and point-aware attacks to perturb these two components separately to misclassify the 3D object. We test the proposed GDA attack using five popular point cloud networks (PointNet, PointNet++, DGCNN, PointTransformer, and PointMLP) on both ModelNet40 and ShapNetPart datasets. Experimental results show that our GDA attack achieves 100% success rates with the lowest perturbation cost. It also demonstrates the increased capability to defeat several existing defense models over other competing attacks.
更多查看译文
关键词
Point cloud processing,disentanglement,geometry-dependent attack
AI 理解论文
溯源树
样例
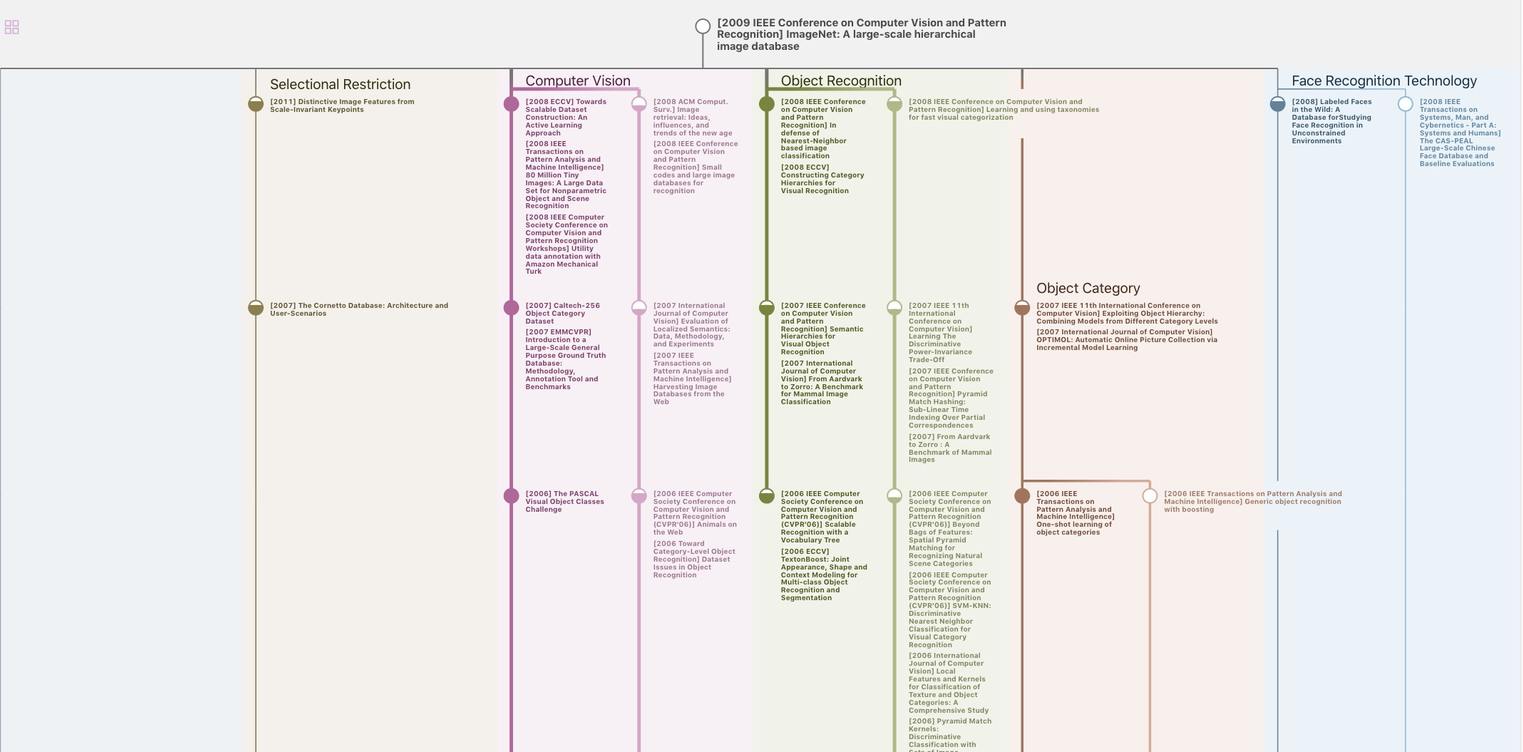
生成溯源树,研究论文发展脉络
Chat Paper
正在生成论文摘要