Data-driven Method for Fault Diagnosis in Braking System of Urban Rail Vehicle Based on Reduced Kernel Principal Component Analysis
Research Square (Research Square)(2023)
摘要
Condition monitoring and fault diagnosis in braking system of urban rail vehicles have important practical value, and can directly improve the stable operation of equipment and passenger comfort. In this study, a data integration system was established to consistently acquire accurate online information, and then the operating conditions were extracted from a massive historical dataset. Kernel principal component analysis (KPCA) is widely used for fault detection and diagnosis, however, the approach is limited by the high computational cost and memory requirements of large-scale training datasets. Herein, the reduced KPCA (RKPCA) approach is developed. The proposed method reduced redundant data in the training dataset by 32.84%. Furthermore, fault detection performance of RKPCA is superior to those of principal component analysis and KPCA.
更多查看译文
关键词
fault diagnosis,braking system,urban rail vehicle,data-driven
AI 理解论文
溯源树
样例
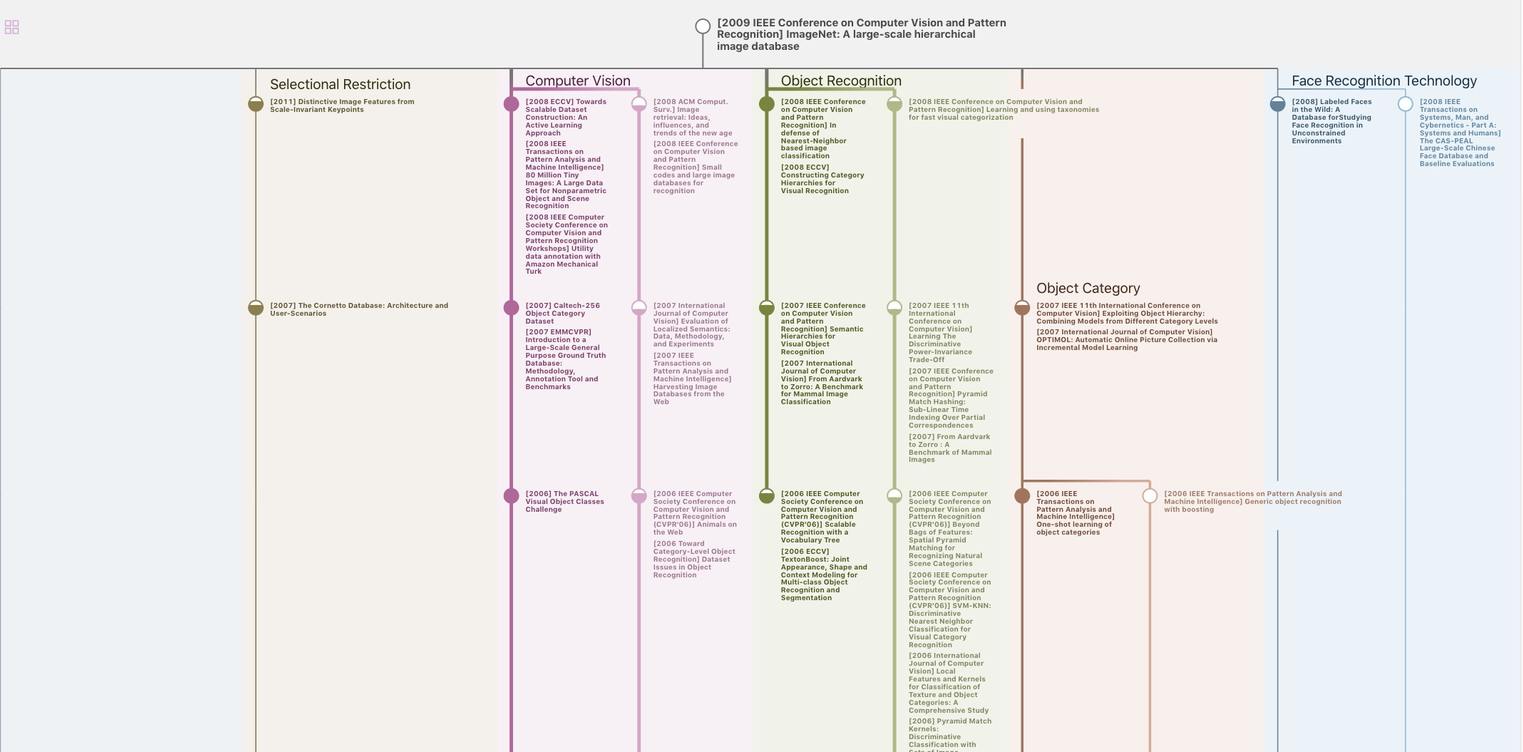
生成溯源树,研究论文发展脉络
Chat Paper
正在生成论文摘要