ReCLIP: Refine Contrastive Language Image Pre-Training with Source Free Domain Adaptation
arXiv (Cornell University)(2023)
摘要
Large-scale Pre-Training Vision-Language Model such as CLIP has demonstrated outstanding performance in zero-shot classification, e.g. achieving 76.3% top-1 accuracy on ImageNet without seeing any example, which leads to potential benefits to many tasks that have no labeled data. However, while applying CLIP to a downstream target domain, the presence of visual and text domain gaps and cross-modality misalignment can greatly impact the model performance. To address such challenges, we propose ReCLIP, the first source-free domain adaptation method for vision-language models, which does not require any source data or target labeled data. ReCLIP first learns a projection space to mitigate the misaligned visual-text embeddings and learns pseudo labels, and then deploys cross-modality self-training with the pseudo labels, to update visual and text encoders, refine labels and reduce domain gaps and misalignments iteratively. With extensive experiments, we demonstrate ReCLIP reduces the average error rate of CLIP from 30.17% to 25.06% on 22 image classification benchmarks.
更多查看译文
关键词
adaptation,refine,language,pre-training
AI 理解论文
溯源树
样例
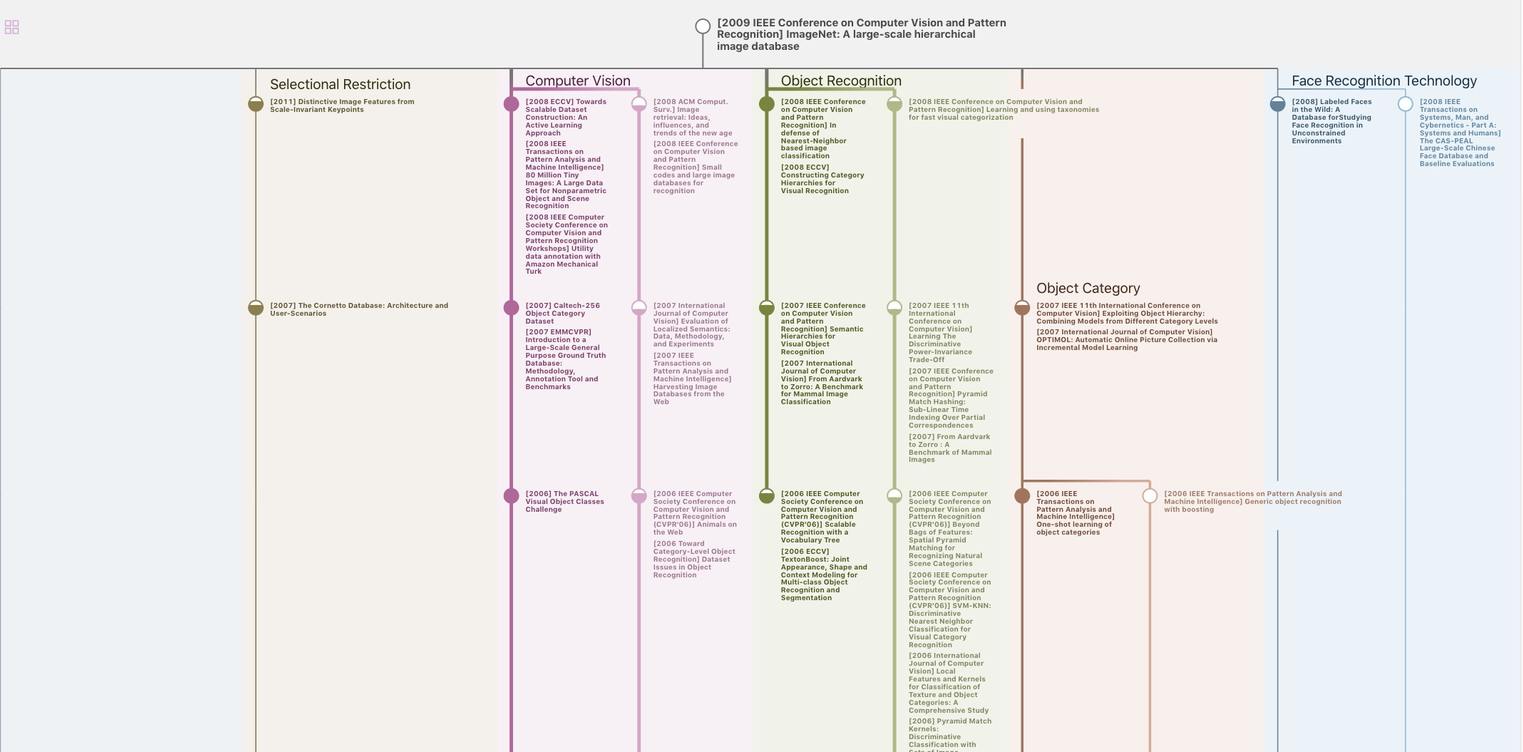
生成溯源树,研究论文发展脉络
Chat Paper
正在生成论文摘要