Efficient Classification by Removing Bayesian Confusing Samples
IEEE TRANSACTIONS ON KNOWLEDGE AND DATA ENGINEERING(2024)
摘要
Improving the generalization performance of classifiers from data pre-processing perspective has recently received considerable attention in the machine learning community. Although many methods have been proposed in the past decades, most of them lack theoretical foundations and cannot guarantee better generalization performance of classifiers on processed datasets. To overcome this flaw, in this paper, we propose a method, which is supported by Bayesian decision theory and percolation theory, to improve generalization performance by removing Bayesian confusing samples (abbr. BCS). Specifically, for a training set, we define the samples that misclassified by the Bayesian optimal classifier as BCS and prove that a classifier trained on the training set after removing BCS can obtain better generalization performance. To find out BCS, we indicate that BCS can be identified according to the size of global homogeneous cluster, a set of samples with the same labels, based on percolation theory. Based on these analysis, we propose a method to construct global homogeneous clusters and remove BCS from the training set. Extensive experiments show that the proposed method is effective for a number of classical and state-of-the-art classifiers.
更多查看译文
关键词
Bayes methods,Training,Task analysis,Data models,Boosting,Support vector machines,Lattices,Generalization performance,classification,data pre-processing,percolation theory
AI 理解论文
溯源树
样例
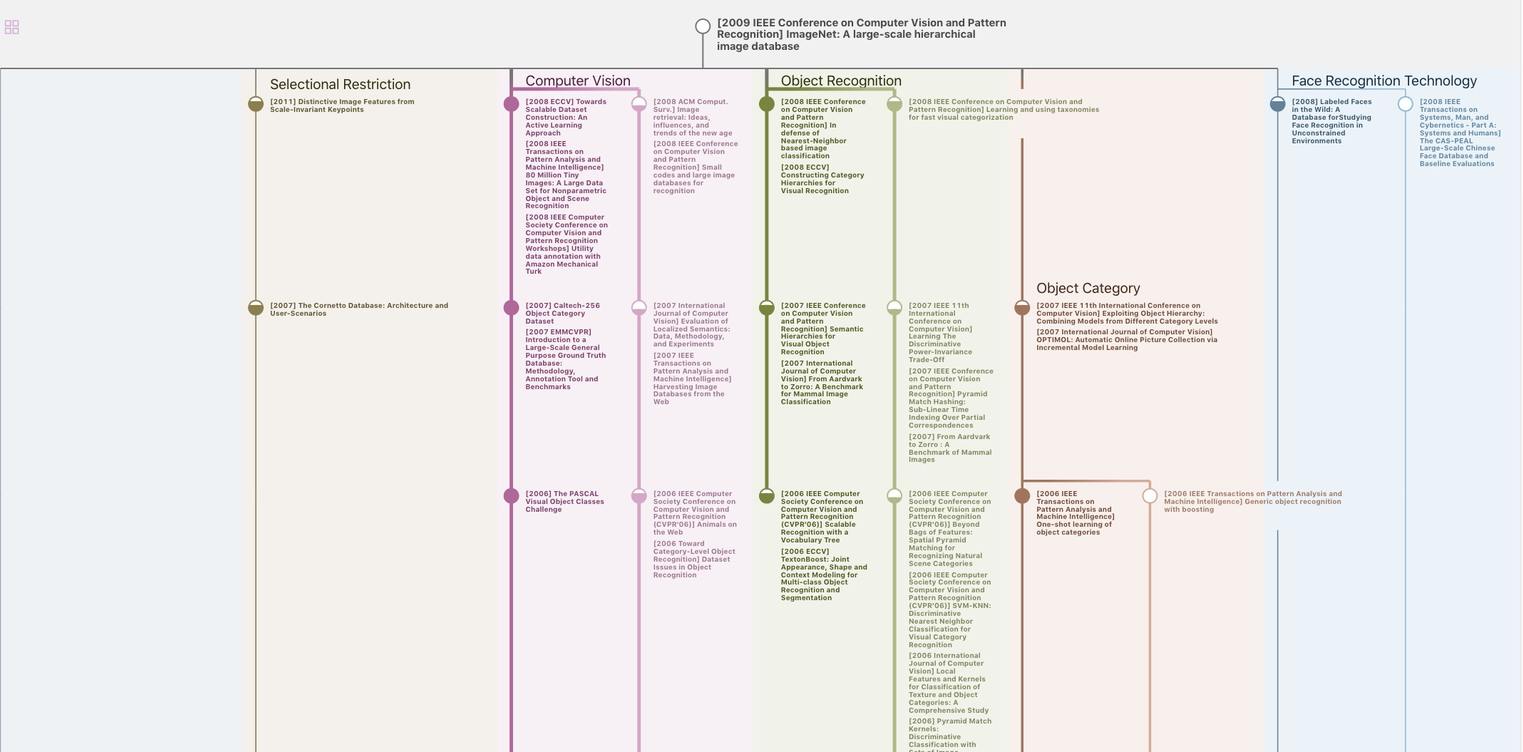
生成溯源树,研究论文发展脉络
Chat Paper
正在生成论文摘要