Development Of Automated Cardiac Arrhythmia Detection Methods Using Single Channel ECG Signal
arXiv (Cornell University)(2023)
摘要
Arrhythmia, an abnormal cardiac rhythm, is one of the most common types of cardiac disease. Automatic detection and classification of arrhythmia can be significant in reducing deaths due to cardiac diseases. This work proposes a multi-class arrhythmia detection algorithm using single channel electrocardiogram (ECG) signal. In this work, heart rate variability (HRV) along with morphological features and wavelet coefficient features are utilized for detection of 9 classes of arrhythmia. Statistical, entropy and energy-based features are extracted and applied to machine learning based random forest classifiers. Data used in both works is taken from 4 broad databases (CPSC and CPSC extra, PTB-XL, G12EC and Chapman-Shaoxing and Ningbo Database) made available by Physionet. With HRV and time domain morphological features, an average accuracy of 85.11%, sensitivity of 85.11%, precision of 85.07% and F1 score of 85.00% is obtained whereas with HRV and wavelet coefficient features, the performance obtained is 90.91% accuracy, 90.91% sensitivity, 90.96% precision and 90.87% F1 score. The detailed analysis of simulation results affirms that the presented scheme effectively detects broad categories of arrhythmia from single-channel ECG records. In the last part of the work, the proposed classification schemes are implemented on hardware using Raspberry Pi for real time ECG signal classification.
更多查看译文
关键词
single channel ecg signal
AI 理解论文
溯源树
样例
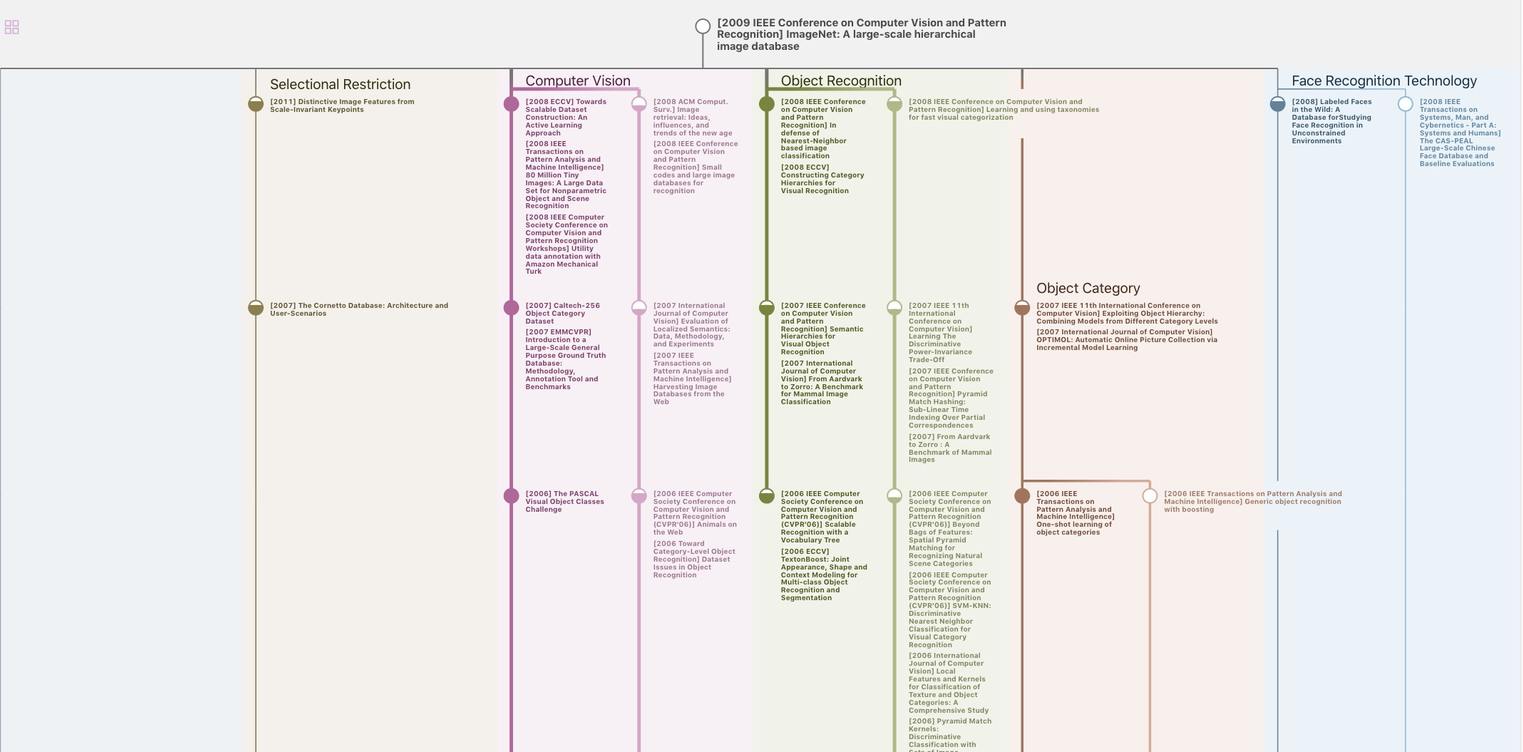
生成溯源树,研究论文发展脉络
Chat Paper
正在生成论文摘要