Ovarian Recurrence risk Assessment using Clinical and serum protein LEvels (ORACLE): A Machine Learning Risk Score to Predict Survival in High Grade Ovarian Cancer
Research Square (Research Square)(2023)
摘要
Abstract In ovarian cancer, there is no current method to accurately predict recurrence after a complete response to chemotherapy. Here, we develop a machine learning risk score using serum proteomics for prediction of early recurrence of ovarian cancer after initial treatment. The developed risk score was validated in an independent cohort who had serum collected prospectively during the remission time period. In the discovery cohort, patients scored as low risk had a median time to recurrence (TTR) that was not reached at 10 years compared to 10.5 months (HR 4.66, p < 0.001) in high risk patients. In the validation cohort, low risk patients had a median TTR which was not reached compared to 4.7 months in high risk patients (HR 4.67, p = 0.009). In advanced stage patients with a CA125 < 10, low risk patients had a median TTR of 68 months compared to 6 months in high risk patients (HR 2.91, p = 0.02). The developed risk score was capable of distinguishing the duration of remission in ovarian cancer patients. This score may help guide maintenance therapy and develop innovative treatments in patients at risk at high risk of recurrence.
更多查看译文
关键词
ovarian cancer,machine learning risk score,risk assessment,serum protein levels
AI 理解论文
溯源树
样例
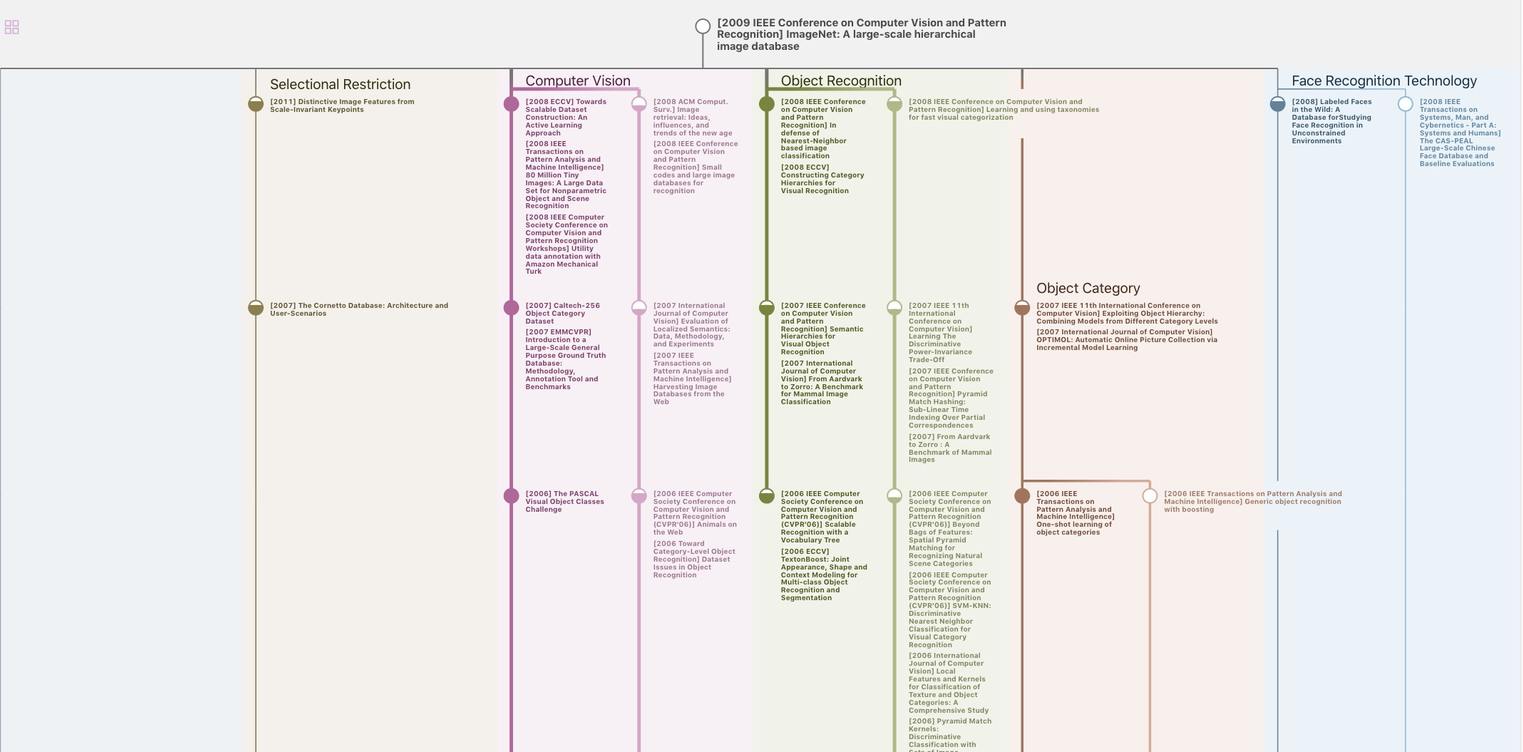
生成溯源树,研究论文发展脉络
Chat Paper
正在生成论文摘要