Plume: A Framework for High Performance Deep RL Network Controllers via Prioritized Trace Sampling
arXiv (Cornell University)(2023)
摘要
Deep Reinforcement Learning (DRL) has shown promise in various networking environments. However, these environments present several fundamental challenges for standard DRL techniques. They are difficult to explore and exhibit high levels of noise and uncertainty. Although these challenges complicate the training process, we find that in practice we can substantially mitigate their effects and even achieve state-of-the-art real-world performance by addressing a factor that has been previously overlooked: the skewed input trace distribution in DRL training datasets. We introduce a generalized framework, Plume, to automatically identify and balance the skew using a three-stage process. First, we identify the critical features that determine the behavior of the traces. Second, we classify the traces into clusters. Finally, we prioritize the salient clusters to improve the overall performance of the controller. Plume seamlessly works across DRL algorithms, without requiring any changes to the DRL workflow. We evaluated Plume on three networking environments, including Adaptive Bitrate Streaming, Congestion Control, and Load Balancing. Plume offers superior performance in both simulation and real-world settings, across different controllers and DRL algorithms. For example, our novel ABR controller, Gelato trained with Plume consistently outperforms prior state-of-the-art controllers on the live streaming platform Puffer for over a year. It is the first controller on the platform to deliver statistically significant improvements in both video quality and stalling, decreasing stalls by as much as 75%.
更多查看译文
关键词
trace selection,network
AI 理解论文
溯源树
样例
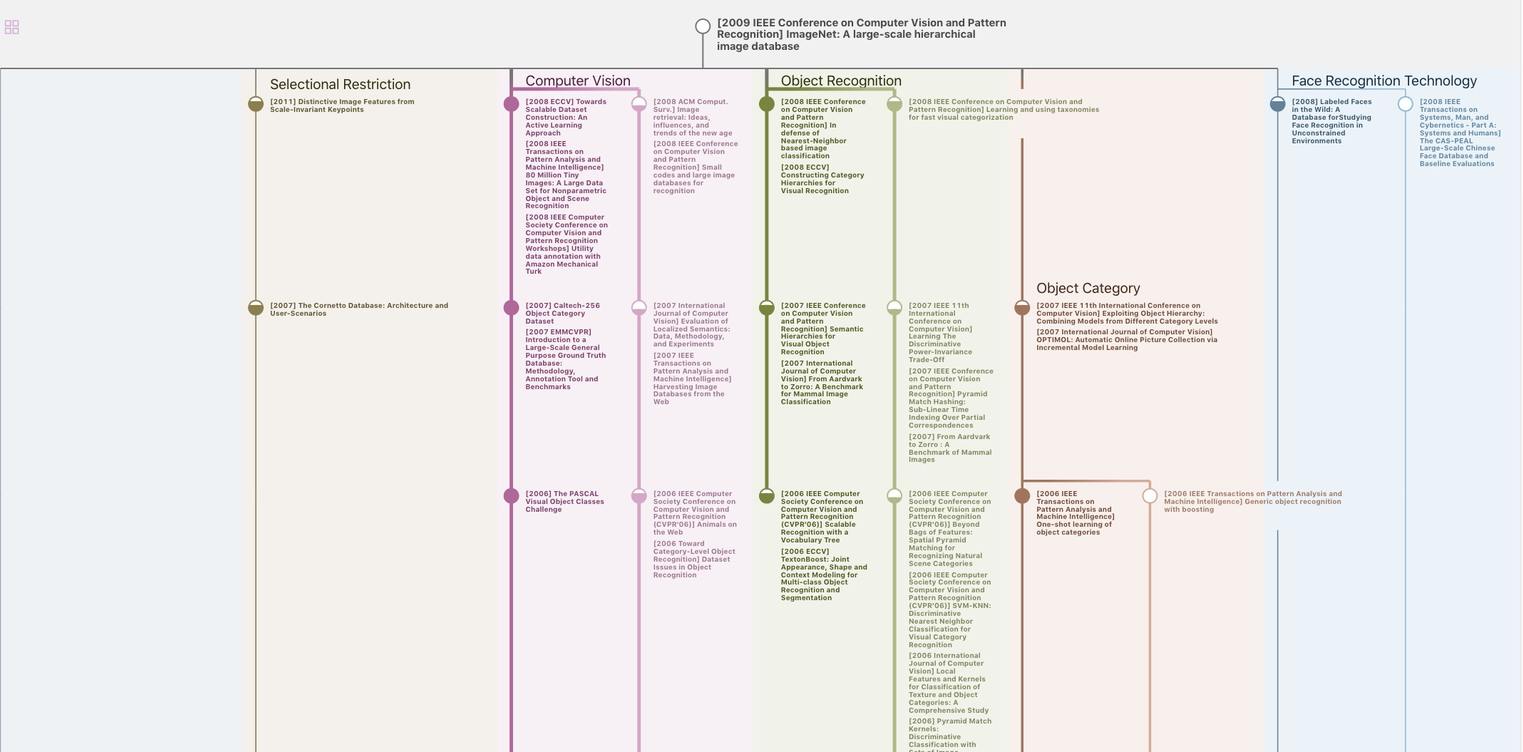
生成溯源树,研究论文发展脉络
Chat Paper
正在生成论文摘要