Optimal Sample Complexity of Reinforcement Learning for Mixing Discounted Markov Decision Processes
arXiv (Cornell University)(2023)
摘要
We consider the optimal sample complexity theory of tabular reinforcement learning (RL) for maximizing the infinite horizon discounted reward in a Markov decision process (MDP). Optimal worst-case complexity results have been developed for tabular RL problems in this setting, leading to a sample complexity dependence on $\gamma$ and $\epsilon$ of the form $\tilde \Theta((1-\gamma)^{-3}\epsilon^{-2})$, where $\gamma$ denotes the discount factor and $\epsilon$ is the solution error tolerance. However, in many applications of interest, the optimal policy (or all policies) induces mixing. We establish that in such settings, the optimal sample complexity dependence is $\tilde \Theta(t_{\text{mix}}(1-\gamma)^{-2}\epsilon^{-2})$, where $t_{\text{mix}}$ is the total variation mixing time. Our analysis is grounded in regeneration-type ideas, which we believe are of independent interest, as they can be used to study RL problems for general state space MDPs.
更多查看译文
关键词
reinforcement learning,complexity
AI 理解论文
溯源树
样例
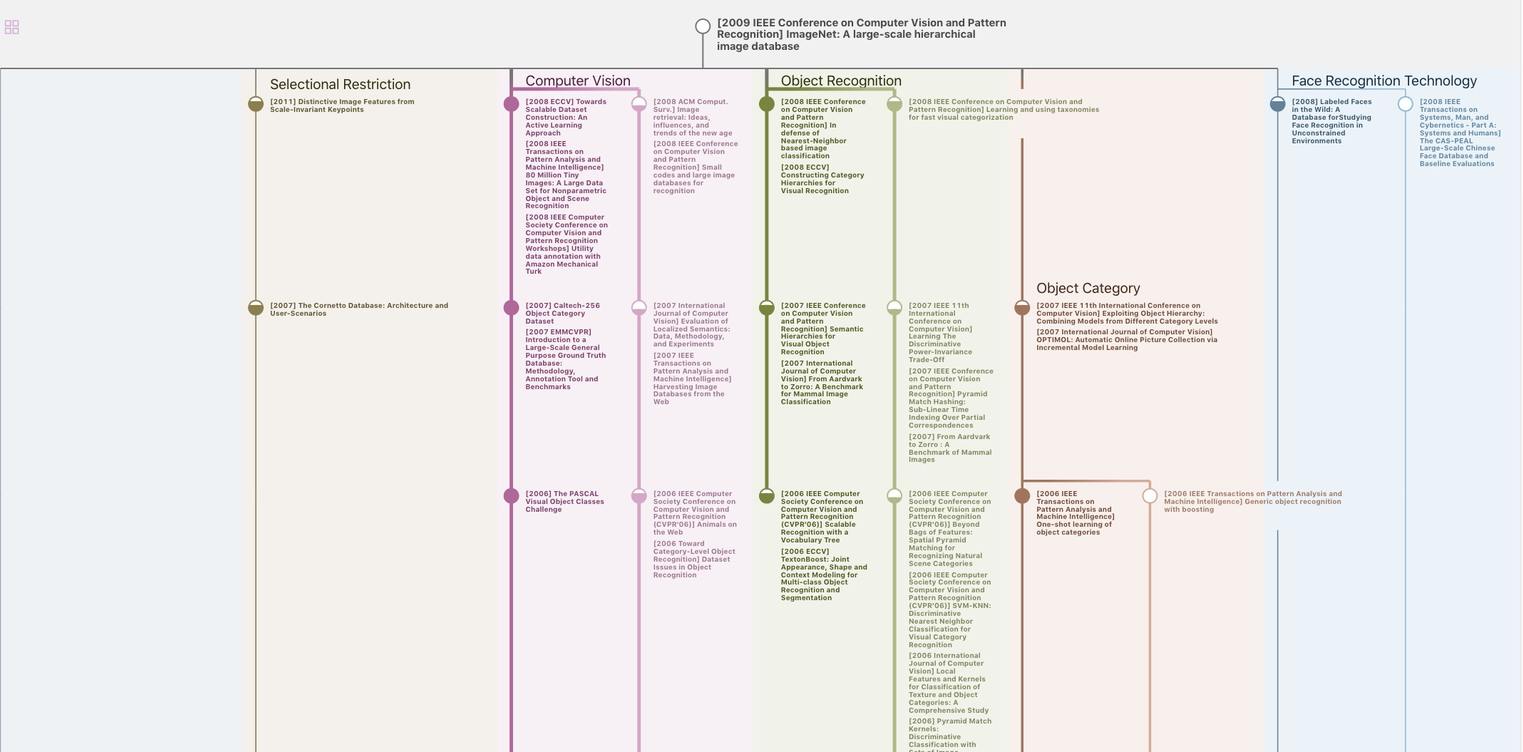
生成溯源树,研究论文发展脉络
Chat Paper
正在生成论文摘要