B-142 Development and Clinical Validation of Artificial Intelligence Assisted Flow Cytometry Diagnosis for Acuteleukemia
Clinical Chemistry(2023)
摘要
Abstract Background Flow cytometry is one of the most commonly used diagnostic tools in hematopathology. Manual flow cytometry data analysis is vulnerable to substantial inter-operator variation and typically takes a technologist 20 min to analyze a case. In the last decade, artificial intelligence (AI) technology has advanced dramatically and become widely used in image processing. Previously, we created an AI-assisted workflow for classifying white blood cell subsets in peripheral blood. The goal of this study is to evaluate the automated flow cytometry analysis system for diagnosing acute leukemia. Methods A total of 117 cases were retrieved from the archived diagnostic cases, consisting of three diagnostic categories: 20 normal cases, 37 acute lymphoblastic leukemia and 60 acute myeloid leukemia. Flow cytometry data manually analyzed by a pathologist is the gold standard. The same flow cytometry data was processed by the flow cytometry analysis software DeepFlow® using proprietary AI algorithm. The final diagnosis and blast cell count of AI and manual analysis are compared by Pearson correlation coefficient. Results The AI-assisted blast cell enumeration showed moderate correlation as compared to manual evaluation (r = 0.8, Fig. 1). Manual adjustment for enumeration of CD34 negative blast population with uncommon immunophenotype is required for 7 cases, including 4 CD34−/CD117+ acute myeloid leukemia and 3 CD34−/TDT+ lymphoblastic leukemia. The AI analysis effectively recognized cell populations and provided correct diagnoses on 94% of cases (110/117). With pathologists’ review, all cases were classified into the correct diagnostic categories. Conclusion Our study demonstrated that AI can accurately recognize the flow cytometry pattern of leukemia and make the proper diagnosis. Additional training for CD34 negative blast gating and other uncommon blast immunophenotypes is being conducted. Ultimately, AI will decrease the demand on manual analysis by technologists and pathologists, standardize the analysis process, and provide accurate diagnosis.
更多查看译文
关键词
acuteleukemia,clinical validation,diagnosis,artificial intelligence
AI 理解论文
溯源树
样例
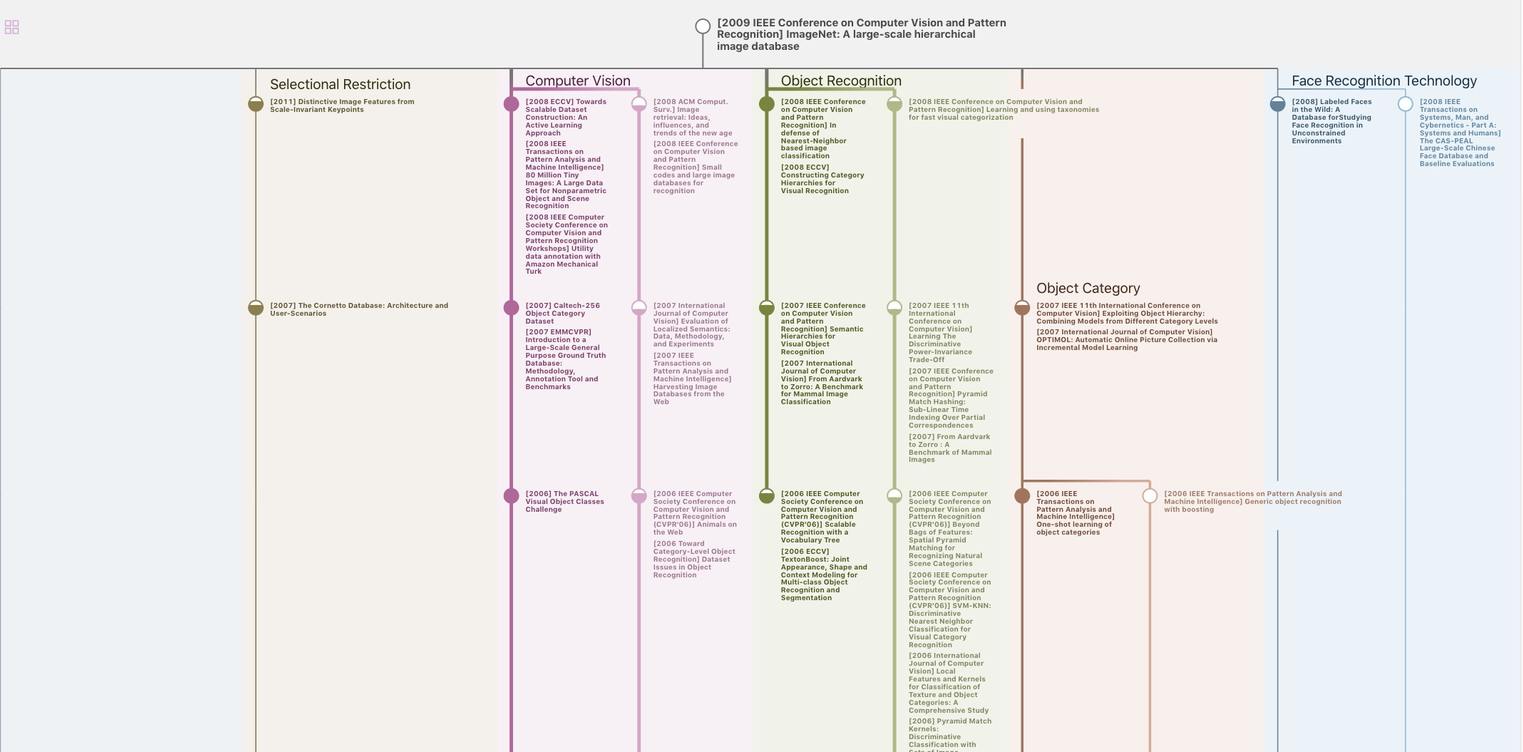
生成溯源树,研究论文发展脉络
Chat Paper
正在生成论文摘要