Incremental Object Detection with Image-level Labels
IEEE transactions on artificial intelligence(2023)
摘要
Incremental object detection (IOD) aims to achieve simultaneous prediction of old and new samples on localization and classification when new concepts are provided. It is a challenging task due to the need for a joint interpretation of semantic and spatial information. While existing work accomplishes the detector generalization with the help of annotated sample classes and bounding boxes, this paper presents one astonishing finding on the unnecessity of new bounding boxes, which will significantly reduce the annotation cost and condition constraints in IOD. To enable the incremental detection process with image-level labels, we propose a multi-branch decoupling scheme in which the representation, classification, and regression branches are customized to accommodate new concepts with class labels with different semantic levels. The regression branch is firstly frozen to ensure generalizable localization while maintaining the stability of representation optimization. Then the representation branch is gradient-inverted to estimate the distribution drift from the old object-level set to the new image-level one, improving the effectiveness of feature updates in the absence of homogeneous semantic and spatial supervision. Finally, the classification branch is calibrated to balance the contribution of different images to the gradient, in which the frequency ratios of old instances and new images are calculated as a post-processing factor for the logits margin. Extensive experiments on two standard incremental detection benchmarks demonstrate the above-par recognition and localization performance while reducing the burden on new class annotation, outperforming state-of-the-art methods by 2% and 3%, respectively.
更多查看译文
关键词
Incremental Learning,Object Detection,Deep Neural Networks
AI 理解论文
溯源树
样例
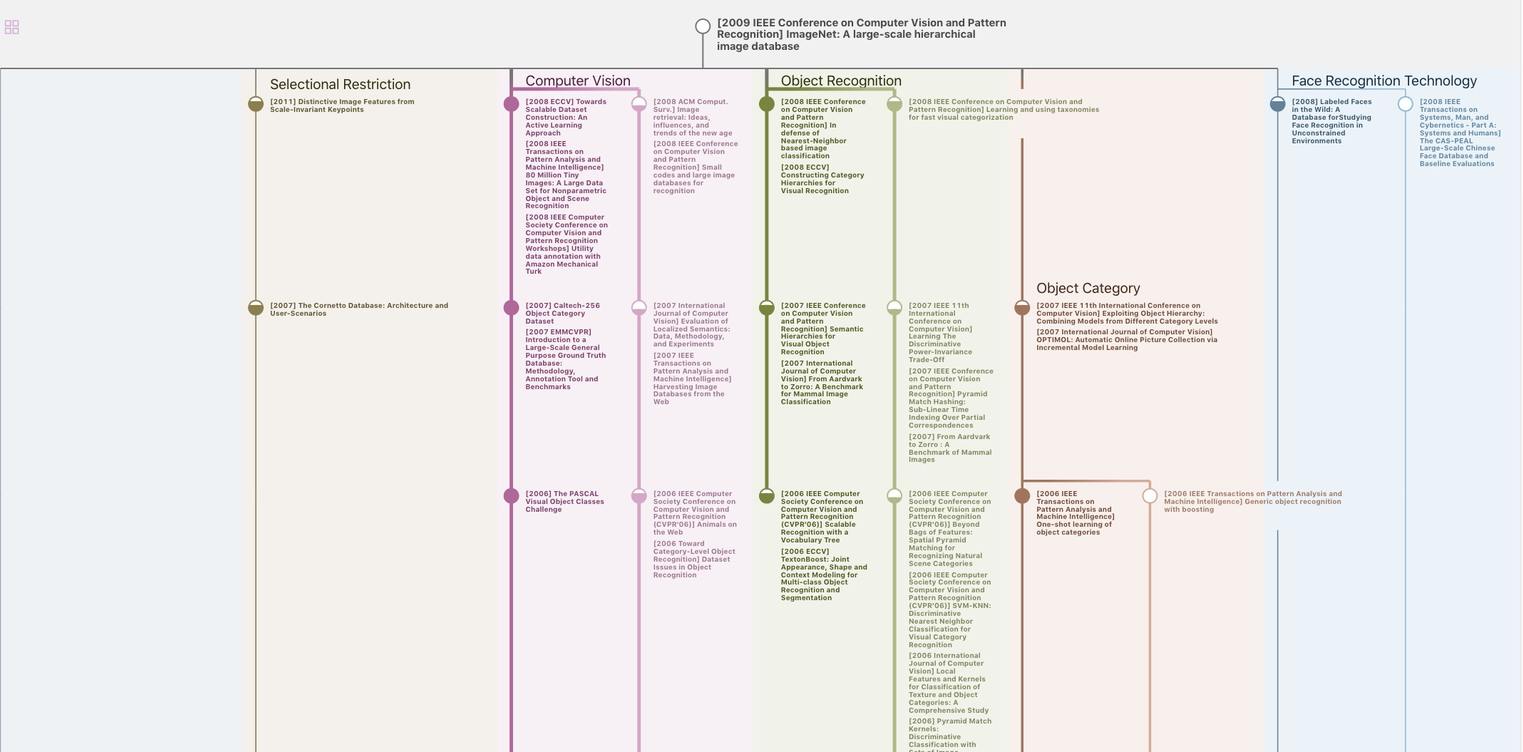
生成溯源树,研究论文发展脉络
Chat Paper
正在生成论文摘要