Dual-Path Transformer for 3D Human Pose Estimation
IEEE Transactions on Circuits and Systems for Video Technology(2023)
摘要
Video-based 3D human pose estimation has achieved great progress, however, it is still difficult to learn precise 2D-3D projection under some hard cases. Multi-level human knowledge and motion information serve as two key elements in the field to conquer the challenges caused by various factors, where the former encodes various human structure information spatially and the latter captures the motion change temporally. Inspired by this, we propose a DualFormer (dual-path transformer) network which encodes multiple human contexts and motion detail to perform the spatial-temporal modeling. Firstly, motion information which depicts the movement change of human body is embedded to provide explicit motion prior for the transformer module. Secondly, a dual-path transformer framework is proposed to model long-range dependencies of both joint sequence and limb sequence. Parallel context embedding is performed initially and a cross transformer block is then appended to promote the interaction of the dual paths which improves the feature robustness greatly. Specifically, predictions of multiple levels can be acquired simultaneously. Lastly, we employ the weighted distillation technique to accelerate the convergence of the dual-path framework. We conduct extensive experiments on three different benchmarks, i.e., Human 3.6M, MPI-INF-3DHP and HumanEva-I. We mainly compute the MPJPE, P-MPJPE, PCK and AUC to evaluate the effectiveness of proposed approach and our work achieves competitive results compared with state-of-the-art approaches. Specifically, the MPJPE is reduced to 42.8mm which is 1.5mm lower than PoseFormer on Human3.6M, which proves the efficacy of the proposed approach.
更多查看译文
关键词
3D human pose estimation,transformer,motion,distillation
AI 理解论文
溯源树
样例
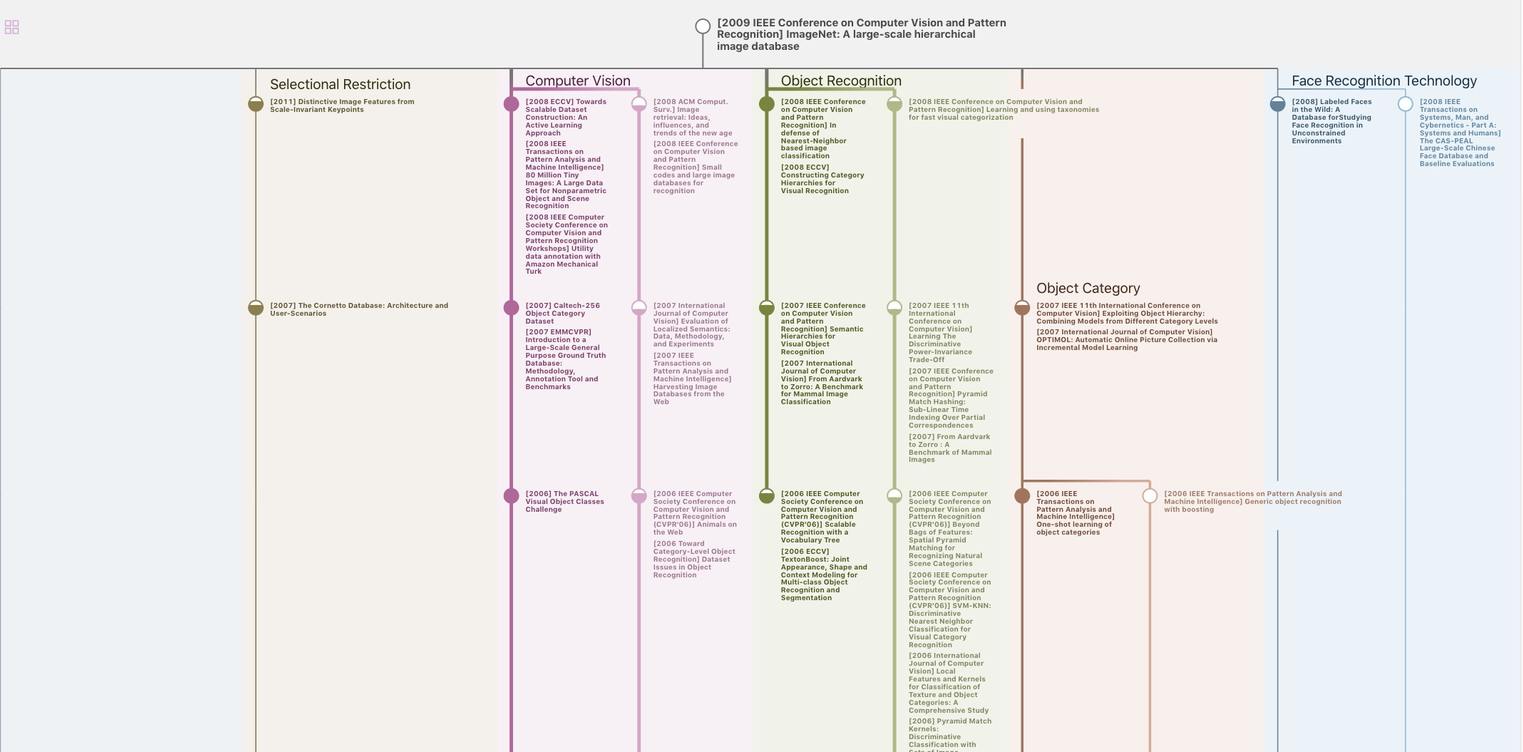
生成溯源树,研究论文发展脉络
Chat Paper
正在生成论文摘要