CoCA: Fusing position embedding with Collinear Constrained Attention for fine-tuning free context window extending
arXiv (Cornell University)(2023)
摘要
Self-attention and position embedding are two key modules in Transformer based LLMs. The potential relationship among them are far from well studied, especially for context window extending. In this paper, we introduce collinear constrained relationship to fuse RoPE and self-attention, and name it as Collinear Constrained Attention (CoCA). We've analyzed the computational and spatial complexity of CoCA and have determined that it adds only minimal additional overhead compared to the original Transformer-based models. We provide an efficient implementation of CoCA, and make it drop-in replacement for any existing position embedding and attention modules in Transformer based models. Experiments show that CoCA performs extraordinary well on context window extending. For instance, a CoCA based GPT model trained with 512 context length can extend the context window up to 8K without perplexity diverging. This indicates more than 16x context window extending without any fine-tuning. Our code is released here: https://github.com/codefuse-ai/Collinear-Constrained-Attention
更多查看译文
关键词
transformers,attention,headache
AI 理解论文
溯源树
样例
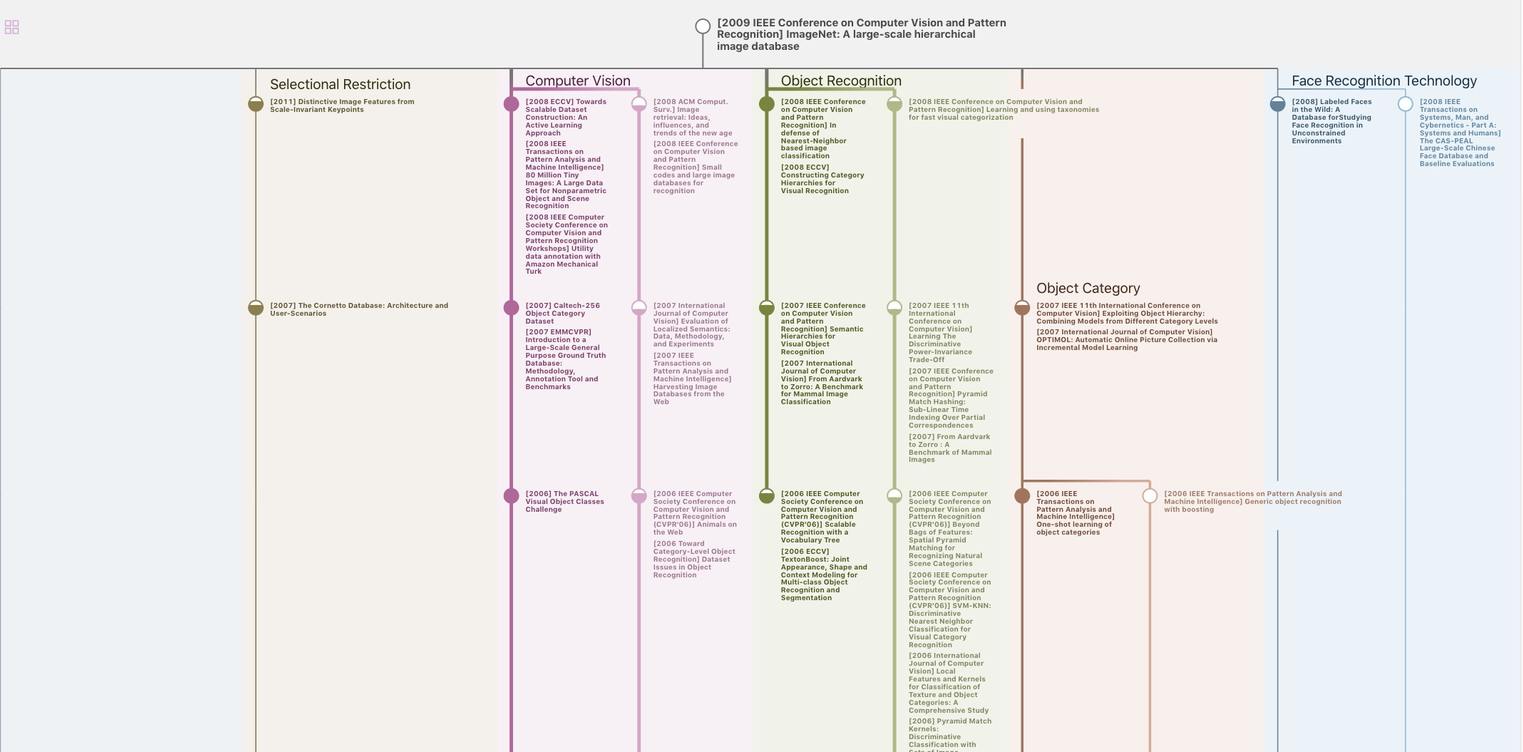
生成溯源树,研究论文发展脉络
Chat Paper
正在生成论文摘要