Causal inference predicts the effect of motion uncertainty in motion integration/segregation tasks.
Journal of Vision(2023)
摘要
Motion integration and segregation are fundamental operations underlying motion perception in ecological vision. Previously, Shivkumar et al. (2022) proposed a Bayesian causal inference model that predicts the transition from integration to segregation in perceptual estimates of motion and, critically, its dependency on sensory uncertainty. However, this dependency on uncertainty has not been empirically tested. To test it, we presented a patch of moving dots (the target) surrounded by two concentric rings of moving dots, and we varied motion coherence to manipulate uncertainty (33, 50, and 100% coherence). Our design disentangles perceiving retinal motion from motion integration percepts, which are indistinguishable in conventional two-ring stimuli. Human observers (n=7) reported the perceived direction of the target patch using a dial. Relative to the target, the motion directions of the inner and outer rings were always clockwise and counterclockwise, respectively. To probe the transition from integration to segregation, we varied the relative direction between the target and the inner ring. For small relative directions, motion integration predicts a clockwise perceptual bias; for large relative directions, segregation predicts a counterclockwise bias. As motion uncertainty increases, our causal inference model predicts that the transition from integration to segregation should occur at larger relative directions between the target and inner ring. Indeed, we found individually significant coherence effects for 5/7 observers at p < 0.001. Specifically, the transition point from integration to segregation changed, on average, from a relative direction of 23 deg at 100% coherence to 49 deg at 33% coherence. The two observers without significant effects showed a similar trend, but had much greater variability in their motion reports. Our results support the predictions of a normative causal inference model of motion perception in complex scenes, where information about objects and their hierarchical relationships are often uncertain.
更多查看译文
关键词
motion uncertainty,motion integration/segregation,integration/segregation tasks
AI 理解论文
溯源树
样例
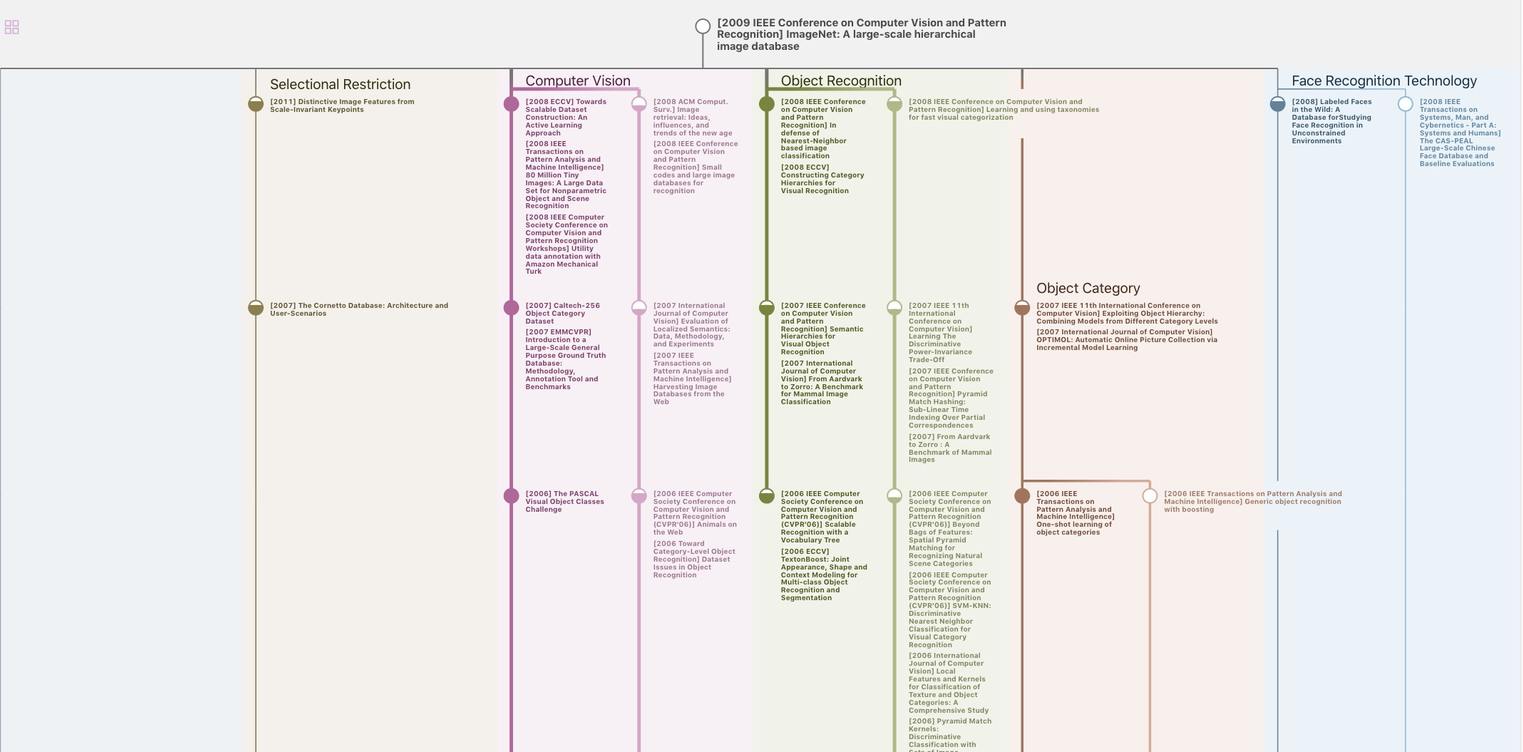
生成溯源树,研究论文发展脉络
Chat Paper
正在生成论文摘要