Latent Gaussian Processes Based Graph Learning for Urban Traffic Prediction.
IEEE Transactions on Vehicular Technology(2024)
摘要
Traffic prediction facilitates various applications in the fields of smart vehicles and vehicular communications, and the key of successfully and accurately forecasting urban traffic state is to model the complex spatiotemporal correlations within urban traffic networks. However, even though great efforts have been devoted to modeling the spatiotemporal correlations, such issue remains challenging in the following two loci, i) capturing dynamic spatial correlations and ii) efficiently and holistically modeling temporal trends. To tackle these challenges, in this article, we propose a novel Adaptive Graph convolution based Autoencoder with Latent Gaussian processes (AGALG). Specifically, a graph fusion module is proposed to fuse learnable graphs for modeling static spatial correlations and data-driven graphs for dynamic spatial correlations. The fused graphs are fed into an adaptive graph convolutional autoencoder whose encoder represents both historical and future data in a latent space where they are holistically modeled by Gaussian processes generated by a latent Gaussian generator. We apply a Gaussian processes regressor to make estimations of latent representations of future data, and the decoder of autoencoder finally generates predictions. Experimental results on several widely used benchmark datasets validate that the proposed model achieves the state-of-the-art performance.
更多查看译文
关键词
Gaussian process,traffic pattern,traffic prediction,vehicular data
AI 理解论文
溯源树
样例
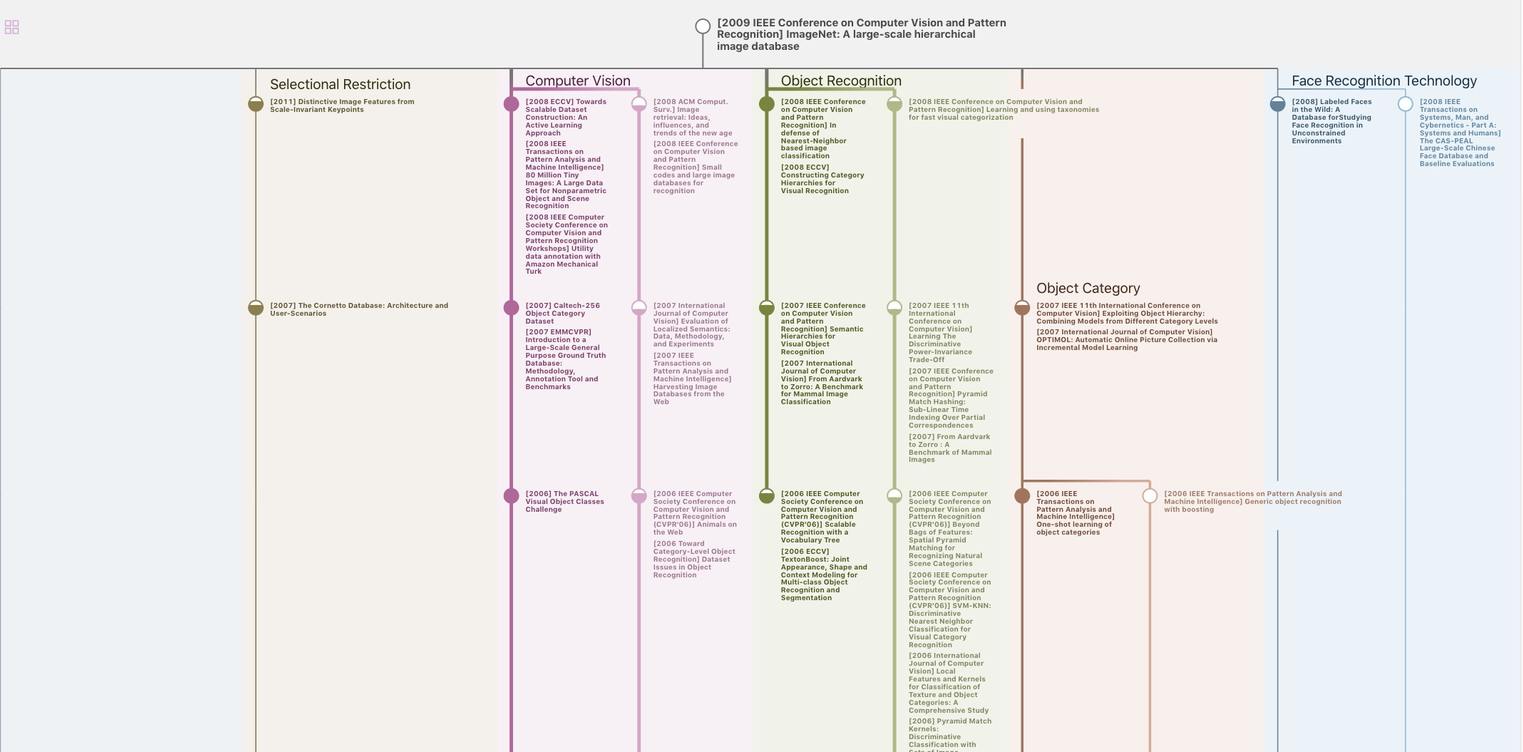
生成溯源树,研究论文发展脉络
Chat Paper
正在生成论文摘要