Hybrid Model Predictive Control for Unmanned Ground Vehicles.
IEEE Trans. Intell. Veh.(2024)
摘要
This paper presents a hybrid approach to enhance the path planning for unmanned ground vehicles (UGVs) by combining stochastic dynamic programming (SDP) based hybrid model predictive control (HMPC) with Dijkstra-based pseudo priority queues (PPQ). The proposed approach employs a platform-specific model that takes into account skid-slip effects, a global cost-to-go (CTG) function, and dynamic obstacles. To achieve efficient path planning on large maps, the proposed approach employs a Dijkstra algorithm and PPQ to generate the CTG function. Moreover, the HMPC process incorporates the latest CTG information, the vehicle model, and the perceived environment, encompassing both static and dynamic obstacles. Extensive simulations have been conducted to comprehensively evaluate the performance of the proposed technique. Through a comparative analysis against existing path planners, the results demonstrate the effectiveness and superiority of the hybrid approach.
更多查看译文
关键词
Cost-to-go function,Model predictive control,Motion planning,Stochastic dynamic programming,Unmanned ground vehicle
AI 理解论文
溯源树
样例
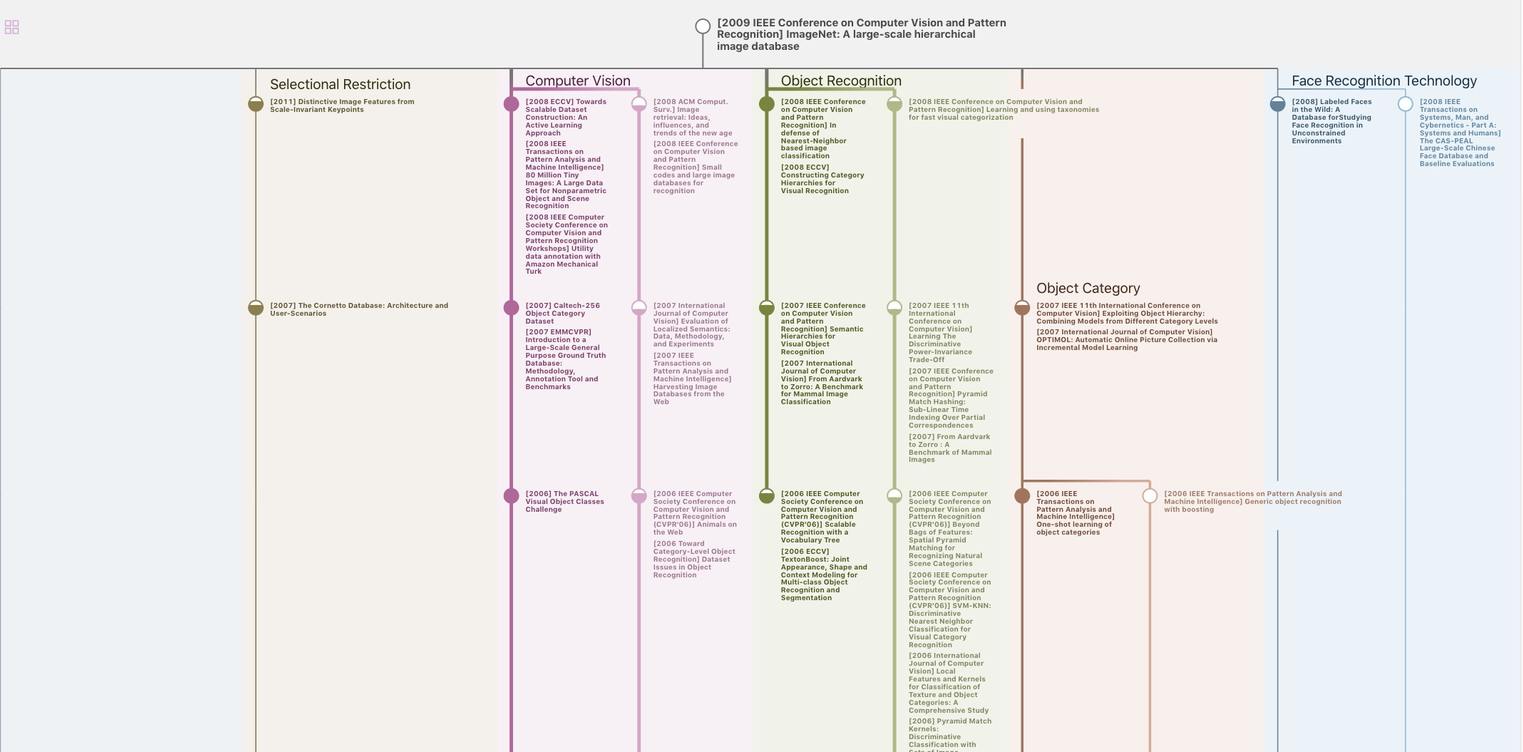
生成溯源树,研究论文发展脉络
Chat Paper
正在生成论文摘要