S121: multiparameter prediction of myeloid neoplasia risk
HemaSphere(2023)
摘要
Background: The myeloid neoplasms (MN) encompass the myeloproliferative neoplasms (MPN), myelodysplastic syndromes (MDS), chronic myelomonocytic leukemia (CMML) and acute myeloid leukemia (AML), and collectively affect approximately 10 per 100,000 individuals per year and the majority of MN patients still succumb to their disease. Recently, it became clear that MN usually develop from clonal hematopoiesis (CH), their shared pre-clinical ancestor and it has been shown that individuals en route to developing AML can be identified years in advance from the mutational characteristics of their CH clones, sparking substantial interest in early detection/ prevention of AML. However, less is known about the predictability of other MN subtypes or the relevance of non-genetic variables to prediction. Our recent analysis of 200,453 United Kingdom Biobank (UKB) participants showed that certain CH driver mutations are associated with a greater risk of progression to MN than others and that some mutations were associated with more significant changes in blood cell parameters. Aims: The recent release of whole exome sequencing (WES) from 454,340 UK biobank (UKB) participants offers an opportunity to use the linked genetic and phenotypic data to develop an improved approach for predicting MN risk in the general population. To do this, we set out to describe the genomic landscape, blood cell and biochemical parameters of the 2045 individuals who developed MN during the 15-year follow-up period and compare these to controls. Using these differences, we then plan to build a regression model to predict the risk of progression to different MN subtypes. Methods: To identify carriers of CH in the UKB, we analyzed WES data from all 454,340 participants using Mutect2 and Samtools focusing on 38 genes known to be recurrently mutated in CH and MN and applied filters to remove sequencing artefacts and germline mutations. We then divided the cohort into training and validation sets with roughly equal numbers of pre-AML, pre-MDS, pre-MPN and control samples. Starting with a core model based solely on age, sex, mutations in genes previously found to be predictive of progression to MN and Variant Allele Fraction (VAF), we used forward stepwise Cox regression on the training set to iteratively add blood/biochemistry parameters to build three distinct multi-parameter models for AML, MDS and MPN prediction. The performances of the three final models were evaluated on the validation set. Results: Each of our three Cox models performed well for predicting future MPN (area under curve (AUC) = 0.83), MDS (AUC = 0.81) and AML (AUC = 0.71) at any time point in the 15-year follow up period. Testing at 1, 2 and 5 years prior to MN diagnosis separately, we found that for AML predictive accuracy was much higher at 0-1 years, compared to 1-5 or >5 years before diagnosis, whereas for MDS and MPN, the model performed similarly well in predicting the development of these neoplasms at >5, 1-5 and 0-1 years before diagnosis. Finally, to aid clinical hematologists managing patients with high risk CH, we built “MN-predict”, a user-friendly web-based application that can predict the risk of MN using selected genetic and blood test parameters. MN-predict enables individualized predictions of the risk of developing different types of MN over time and also aggregates these predictions to infer the probability of MN-free survival. Summary/Conclusion: Our study provides proof-of-principle that individuals that develop any MN subtype can be identified years in advance and provides a framework for disease-specific prognostication that will be of substantial use to researchers and physicians investigating early detection/prevention of these lethal cancers.Keywords: Survival prediction, Myeloid leukemia, Myeloid malignancies
更多查看译文
关键词
multiparameter prediction,risk
AI 理解论文
溯源树
样例
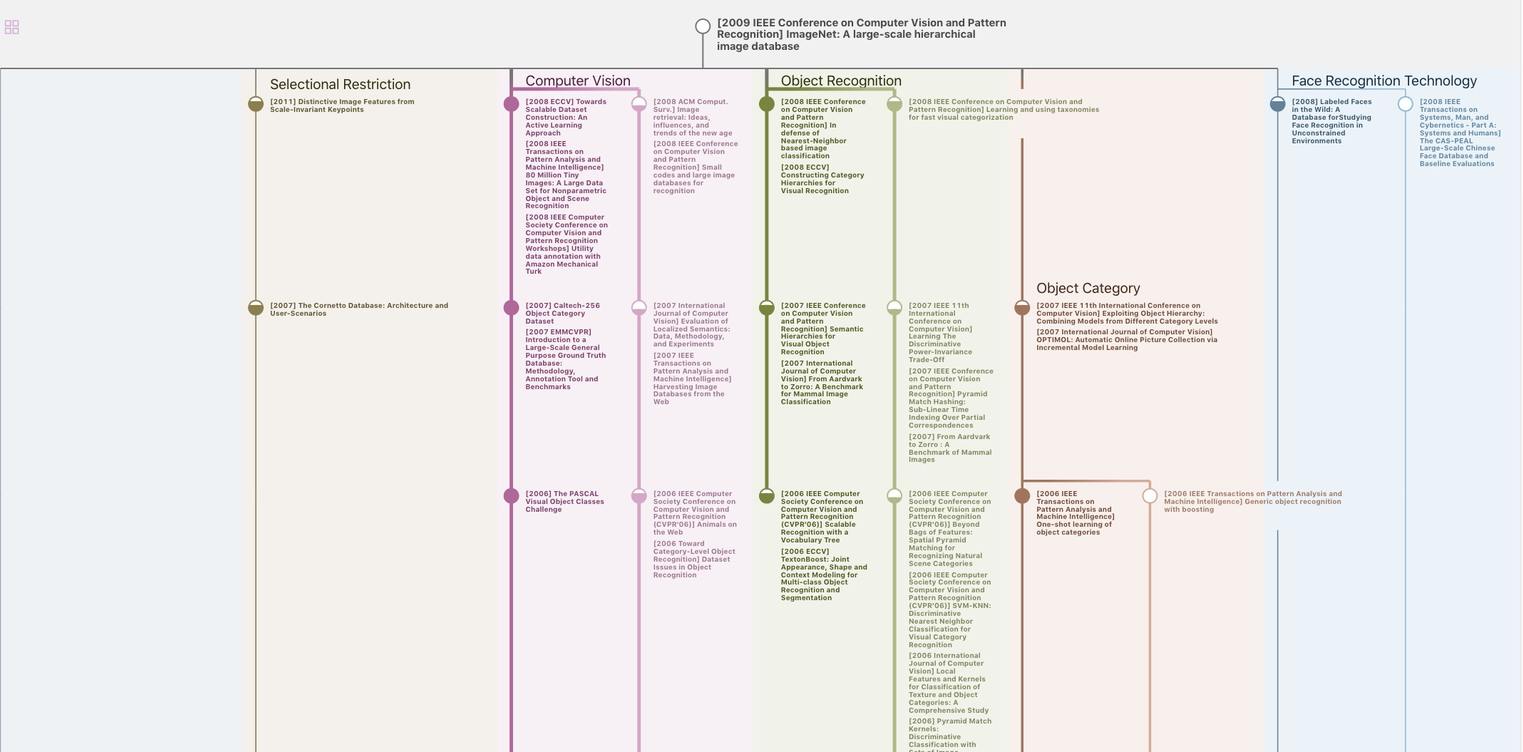
生成溯源树,研究论文发展脉络
Chat Paper
正在生成论文摘要