Spatio-Temporal Graph Analytics on Secondary Affect Data for Improving Trustworthy Emotional AI.
IEEE Trans. Affect. Comput.(2024)
摘要
Ethical affective computing (AC) requires maximizing the benefits to users while minimizing its harm to obtain trust from users. This requires responsible development and deployment to ensure fairness, bias mitigation, privacy preservation, and accountability. To obtain this, we require methodologies that can quantify, visualize, analyze, and mine insights from affect data. Hence, in this article, we propose a spatio-temporal model for representing secondary affect data from network sciences’ perspective. We propose a network science-based model to represent spatio-temporal data, e.g., action units’ sequences, and continuous affect reports. In particular, the proposed model captures the spatial and temporal strength of the relationship among essential variables in the data. The proposed model allows to analyze data as a whole system. We also demonstrated the use case of the model for graph analytics on secondary affect data that can assist to measure and quantify several issues that can be originated from the study setup, data recording devices, and the influences/biases that can originate from the perspective of the affect reporters. We also demonstrated the use cases of the proposed method on ethical trustworthy emotional AI via measuring biases from de-identified data and how it contributes towards ethics, transparency, value alignment, and governance.
更多查看译文
关键词
Spatio-temporal network model,affective graph analytics,data quality inspection,fairness and biases from data collection,recording,and contextual perspectives,trustworthy ethical AC
AI 理解论文
溯源树
样例
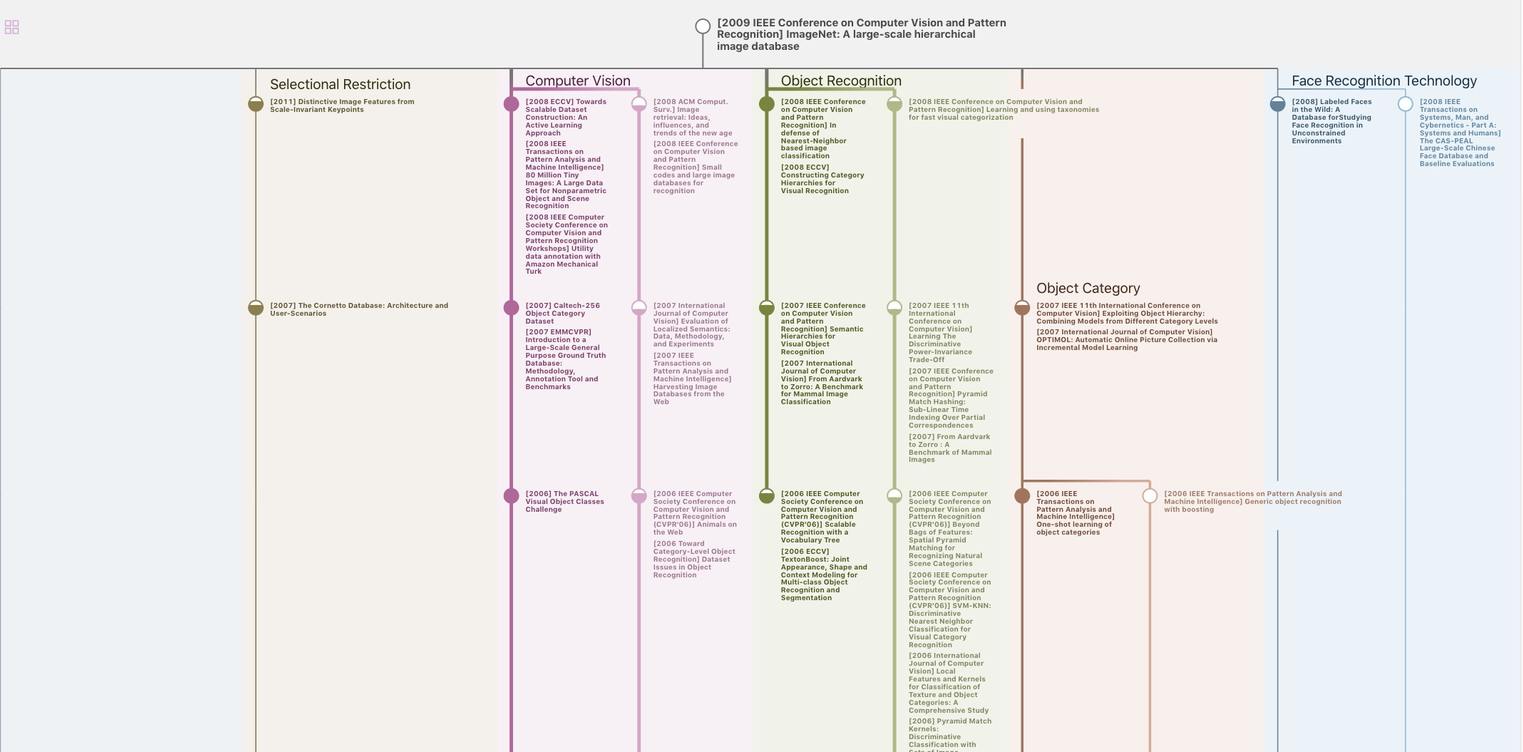
生成溯源树,研究论文发展脉络
Chat Paper
正在生成论文摘要