Depth Maps-Based 3D Convolutional Neural Network and 3D Skeleton Information with Time Sequence for HAR
Algorithms for intelligent systems(2023)
摘要
The traditional methodology is to design a handcrafted feature descriptor to represent the object. In the last decade, with the development of computer software and hardware technology, the deep learning method has been applied in various research and application domains, such as artificial intelligence and computer vision in the real world. Unlike the handcrafted methods to design the feature descriptor for human action recognition, the deep learning-based approaches directly extract feature maps after the neural network processing. Compared with the RGB video, depth sequences are more advantageous in lighting changes and information collection. In this paper, we study depth maps based on deep learning and corresponding skeleton joint information for human activity recognition. The 3D convolutional neural network (3DCNN) directly learns spatial–temporal features from depth maps sequence. Geometric information where skeleton joint point distance, angle information and time sequences of skeleton point changes in different frames are extracted to merge deep learning features map. The merged features descriptor was put into SVM for activity classification. The MSR-Action3D benchmark dataset 1/3 was divided into testing data, training is the rest. The experimental result is compared with the superior performance on the MSR-Action3D benchmark dataset. Our proposed technique proves that it has very promising classification accuracy...
更多查看译文
关键词
3d skeleton information,3d convolutional neural network,depth,maps-based
AI 理解论文
溯源树
样例
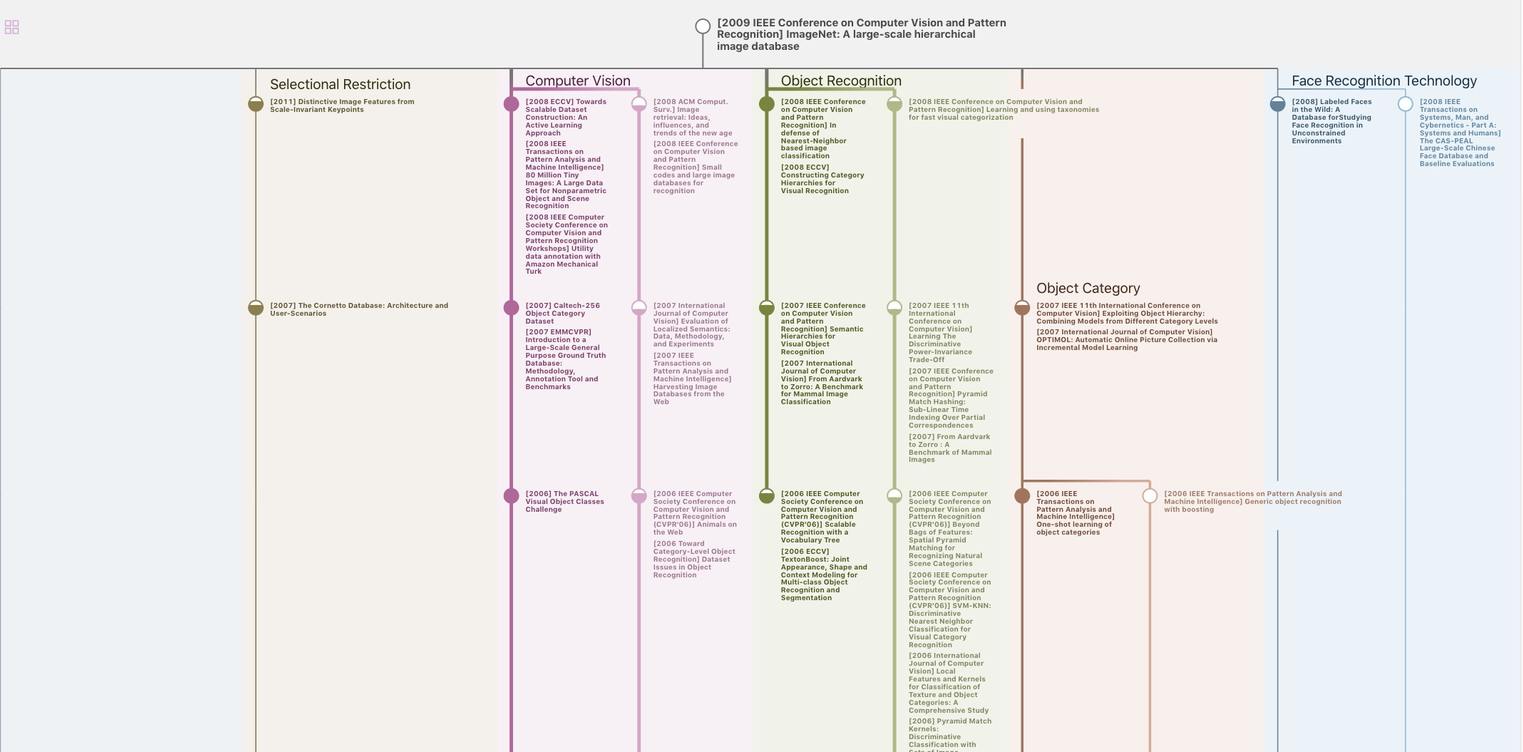
生成溯源树,研究论文发展脉络
Chat Paper
正在生成论文摘要