Integrating Policy Summaries with Reward Decomposition for Explaining Reinforcement Learning Agents
Lecture Notes in Computer Science(2023)
摘要
Explainable reinforcement learning methods can roughly be divided into local explanations that analyze specific decisions of the agents and global explanations that convey the general strategy of the agents. In this work, we study a novel combination of local and global explanations for reinforcement learning agents. Specifically, we combine reward decomposition, a local explanation method that exposes which components of the reward function influenced a specific decision, and HIGHLIGHTS, a global explanation method that shows a summary of the agent’s behavior in decisive states. Results from two user studies show significant benefits for both methods. We found that the local reward decomposition was more useful for identifying the agents’ priorities. However, when there was only a minor difference between the agents’ preferences, the global information provided by HIGHLIGHTS additionally improved participants’ understanding.
更多查看译文
关键词
reward decomposition,policy summaries,reinforcement learning
AI 理解论文
溯源树
样例
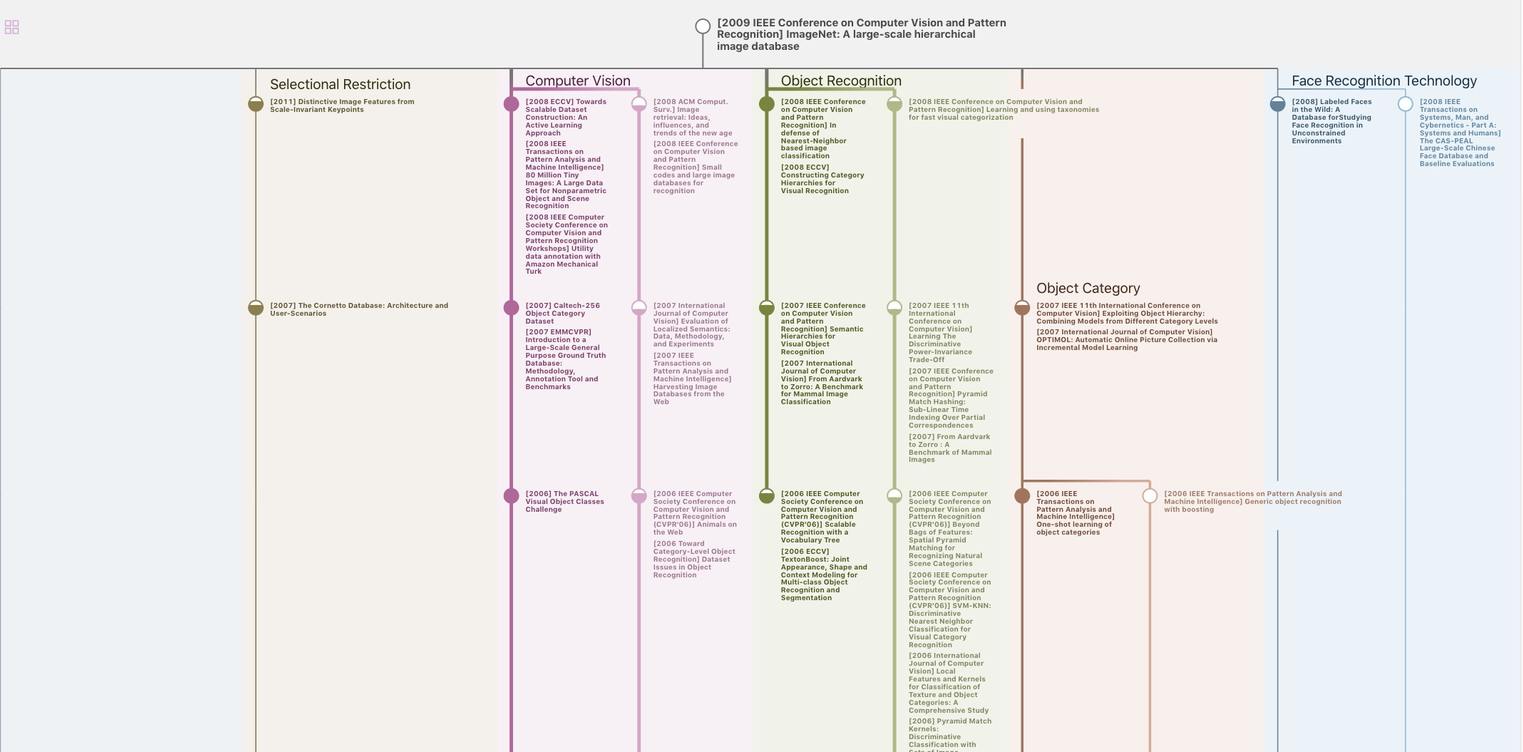
生成溯源树,研究论文发展脉络
Chat Paper
正在生成论文摘要