Scalable quasi-static self-modeling for physical legged locomotion
Research Square (Research Square)(2023)
摘要
Abstract Self-modeling refers to an agent's ability to learn a predictive model of its own behavior. A continuously adapted self-model can serve as an internal simulator, enabling the agent to plan and assess various potential behaviors internally, reducing the need for expensive physical experimentation. Self-models are especially important in legged locomotion, where manual modeling is difficult, reinforcement learning is slow, and physical experimentation is risky. Here, we propose a Quasi-static Self-Modeling framework that focuses on learning a predictive model only of high-level quasi-static dynamics, rather than a continuous model. Experimental results on a 12-degree-of-freedom-legged robot demonstrate improvements over model-free and traditional model-based continuous approaches. Using 80 diverse robot morphologies, we confirm a correlation of R 2 =0.94 between the improvements rendered by our method and the DoF of the robot, suggesting that as future robots increase in complexity, this approach will become more valuable.
更多查看译文
关键词
physical legged locomotion,quasi-static,self-modeling
AI 理解论文
溯源树
样例
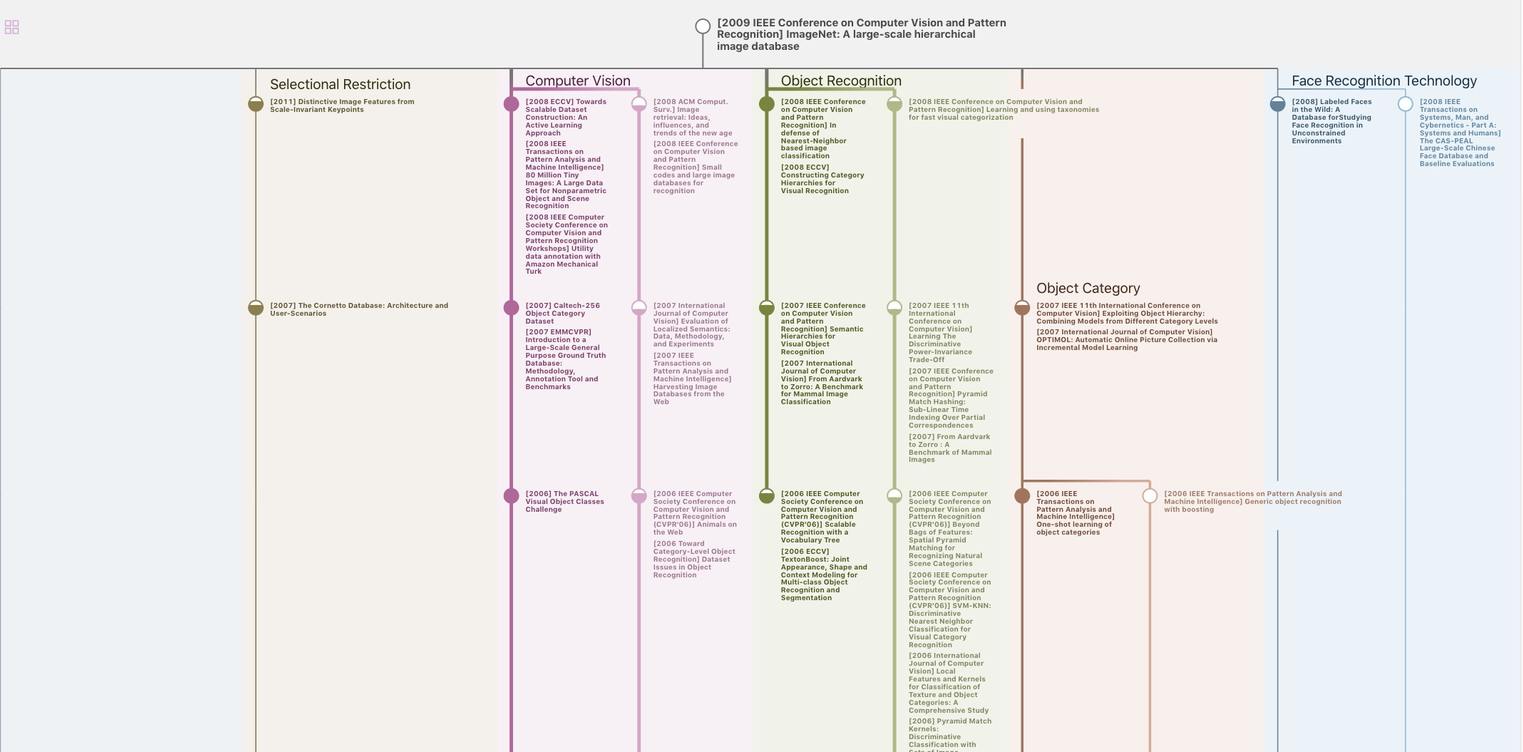
生成溯源树,研究论文发展脉络
Chat Paper
正在生成论文摘要