Single image dehazing using frequency-guided filtering and progressive physics learning
JOURNAL OF ELECTRONIC IMAGING(2023)
摘要
Current learning-based dehazing methods simply rely on the paired synthetic datasets and physical models, which can hardly describe the complicated degradation for practical applications. These methods still struggle to achieve haze removal, color distortion, and detail restoration instantly, and they ignore the frequency characteristic differences and prior knowledge importance. To address these problems, we propose an unpaired stage-wise framework integrating frequency-guided filtering and progressive physics learning in an adversarial dehazing network, called FPD-Net. To be specific, a guided filter based on frequency information is employed to decompose the high and low frequency components for better feature extraction. We further merge the prior and physical knowledge to form progressive physics learning, which produces pleasing haze-free outputs with high visibility and reality. For better atmospheric light estimation, the variational auto-encoder and Kullback-Leibler loss are included to represent the illumination message. Extensive experiments on both synthetic and real datasets prove that our designed FPD-Net achieves better performance visually and quantitatively than the comparison dehazing models.(c) 2023 SPIE and IS&T
更多查看译文
关键词
image dehazing,unpaired learning,frequency filtering,progressive physics learning,variational auto-encoder
AI 理解论文
溯源树
样例
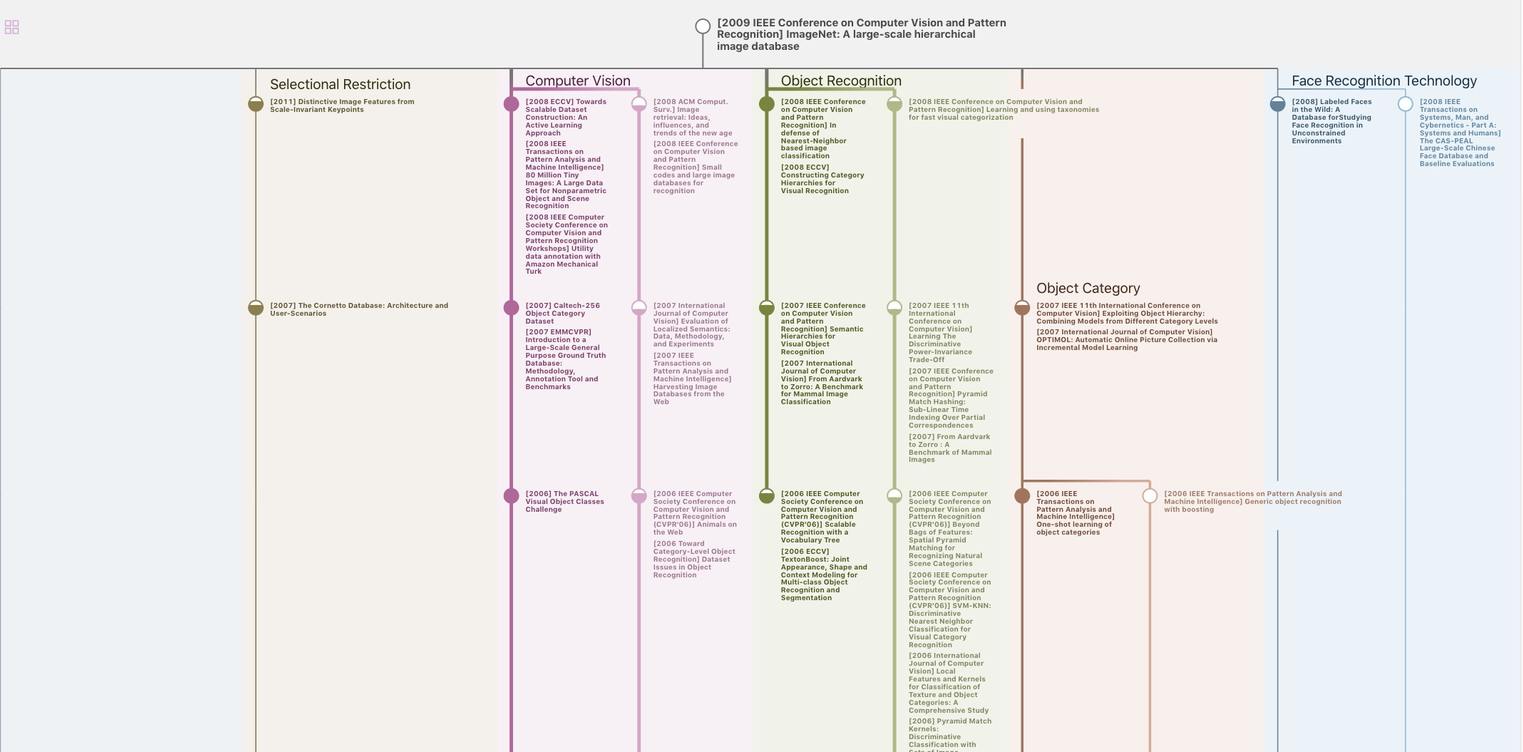
生成溯源树,研究论文发展脉络
Chat Paper
正在生成论文摘要