Water-tolerant and anti-dust CeCo-MnO2 membrane catalysts for low temperature selective catalytic reduction of nitrogen oxides
JOURNAL OF ENVIRONMENTAL CHEMICAL ENGINEERING(2023)
摘要
The present work successfully proposes a domain knowledge-guided Machine Learning (ML) strategy, which successes the development of a water-tolerant anti-dust catalyst for Low-Temperature (LT) Selective Catalytic Reduction (SCR) of nitrogen oxides (NOx) from catalyst discovery to industrial deployment. The discovered catalyst is able to convert 99 % of NOx at 150 degrees C in the standard waste gas, even in the waste gas containing 7 vol % of water vapors, the efficiency is still retained at 97 %. The superior LT activity and water-tolerance are attributed to abundant surface-active oxygen, Bronsted acid and microcellular structure. The SCR reaction mainly follows the Eley-Rideal (E-R) pathway driven by Bronsted acid and the Langmuir-Hinshelwood (L-H) pathway maintained by abundant reactive oxygen species in moisture waste gases. And then, catalyst is synthesized on a polyphenylene sulfide filter to render the membrane configuration in order to have the anti-dust ability. The final membrane catalyst has the capacity of converting 95 % NOx in the waste gas containing 7 vol% of moisture at 150 degrees C and the dedusting, and denitrification ability. The domain knowledge-guided Machine Learning (ML) strategy paves a wide avenue for the whole chain development from the data-driven discovery to the industrial deployment associated with mechanism exploration.
更多查看译文
关键词
Machine learning,Anti-dust membrane catalyst,Selective catalytic reduction,Low-temperature activity,Water tolerance
AI 理解论文
溯源树
样例
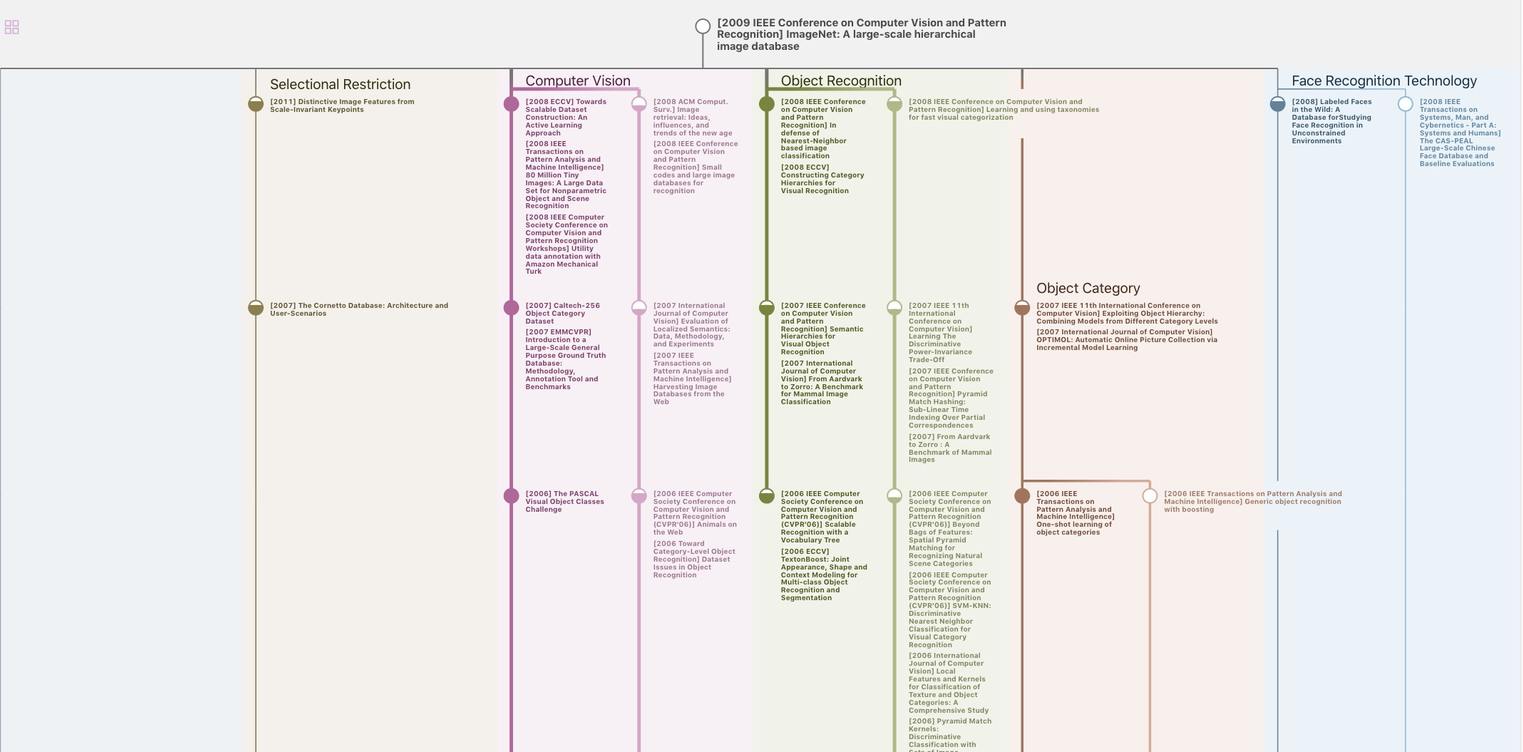
生成溯源树,研究论文发展脉络
Chat Paper
正在生成论文摘要