Deep Semantic-Aware Proxy Hashing for Multi-Label Cross-Modal Retrieval
IEEE TRANSACTIONS ON CIRCUITS AND SYSTEMS FOR VIDEO TECHNOLOGY(2024)
摘要
Deep hashing has attracted broad interest in cross-modal retrieval because of its low cost and efficient retrieval benefits. To capture the semantic information of raw samples and alleviate the semantic gap, supervised cross-modal hashing methods that utilize label information which could map raw samples from different modalities into a unified common space, are proposed. Although making great progress, existing deep cross-modal hashing methods are suffering from some problems, such as: 1) considering multi-label cross-modal retrieval, proxy-based methods ignore the data-to-data relations and fail to explore the combination of the different categories profoundly, which could lead to some samples without common categories being embedded in the vicinity; 2) for feature representation, image feature extractors containing multiple convolutional layers cannot fully obtain global information of images, which results in the generation of sub-optimal binary hash codes. In this paper, by extending the proxy-based mechanism to multi-label cross-modal retrieval, we propose a novel Deep Semantic-aware Proxy Hashing (DSPH) framework, which could embed multi-modal multi-label data into a uniform discrete space and capture fine-grained semantic relations between raw samples. Specifically, by learning multi-modal multi-label proxy terms and multi-modal irrelevant terms jointly, the semantic-aware proxy loss is designed to capture multi-label correlations and preserve the correct fine-grained similarity ranking among samples, alleviating inter-modal semantic gaps. In addition, for feature representation, two transformer encoders are proposed as backbone networks for images and text, respectively, in which the image transformer encoder is introduced to obtain global information of the input image by modeling long-range visual dependencies. We have conducted extensive experiments on three baseline multi-label datasets, and the experimental results show that our DSPH framework achieves better performance than state-of-the-art cross-modal hashing methods. The code for the implementation of our DSPH framework is available at https://github.com/QinLab-WFU/DSPH.
更多查看译文
关键词
Semantics,Codes,Transformers,Feature extraction,Correlation,Hash functions,Visualization,Deep hashing,semantic-aware proxy,cross-modal retrieval,semantic relationships,transformer encoder
AI 理解论文
溯源树
样例
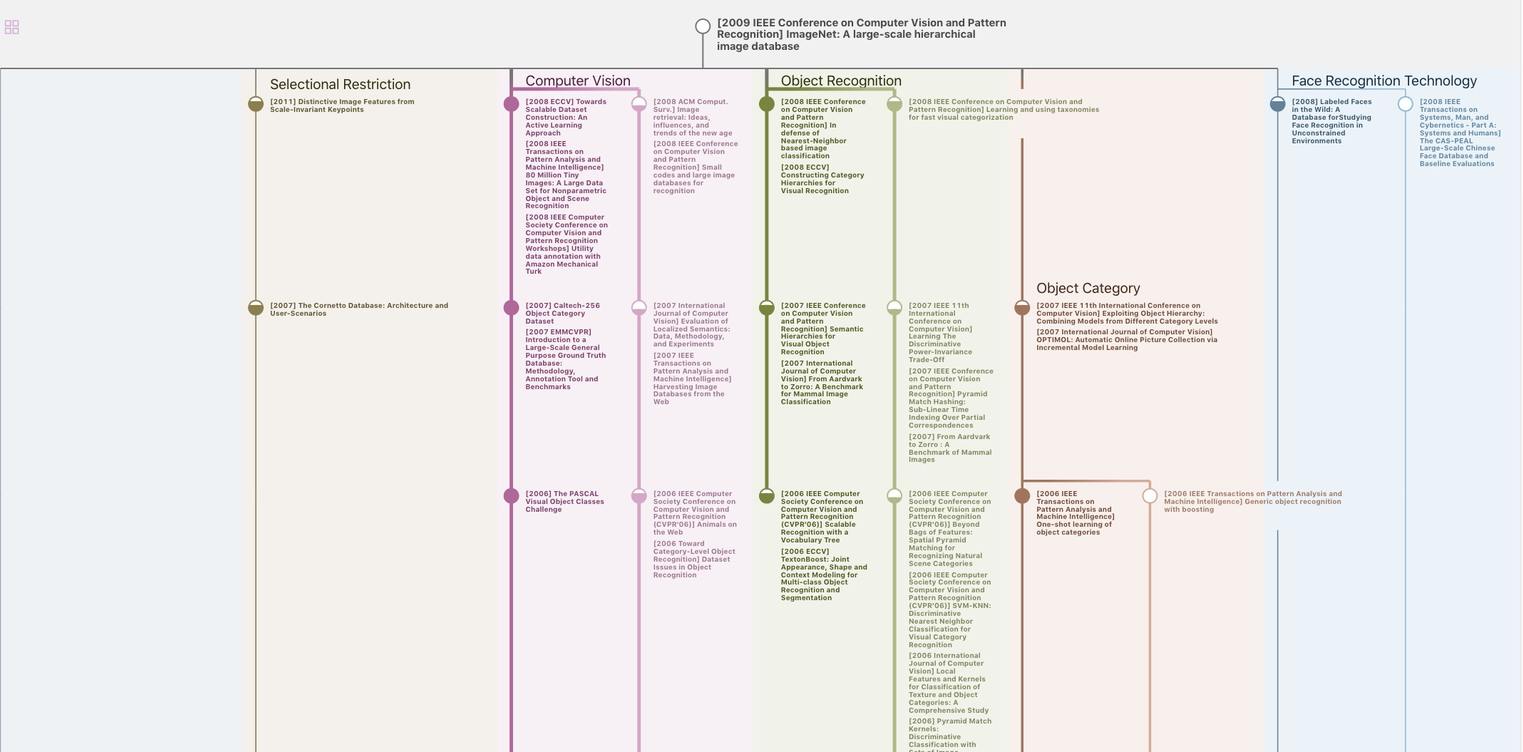
生成溯源树,研究论文发展脉络
Chat Paper
正在生成论文摘要