Memory Efficient Neural Processes via Constant Memory Attention Block
arXiv (Cornell University)(2023)
摘要
Neural Processes (NPs) are popular meta-learning methods for efficiently modelling predictive uncertainty. Recent state-of-the-art methods, however, leverage expensive attention mechanisms, limiting their applications, particularly in low-resource settings. In this work, we propose Constant Memory Attention Block (CMAB), a novel general-purpose attention block that (1) is permutation invariant, (2) computes its output in constant memory, and (3) performs updates in constant computation. Building on CMAB, we propose Constant Memory Attentive Neural Processes (CMANPs), an NP variant which only requires \textbf{constant} memory. Empirically, we show CMANPs achieve state-of-the-art results on popular NP benchmarks (meta-regression and image completion) while being significantly more memory efficient than prior methods.
更多查看译文
关键词
memory,attention,processes
AI 理解论文
溯源树
样例
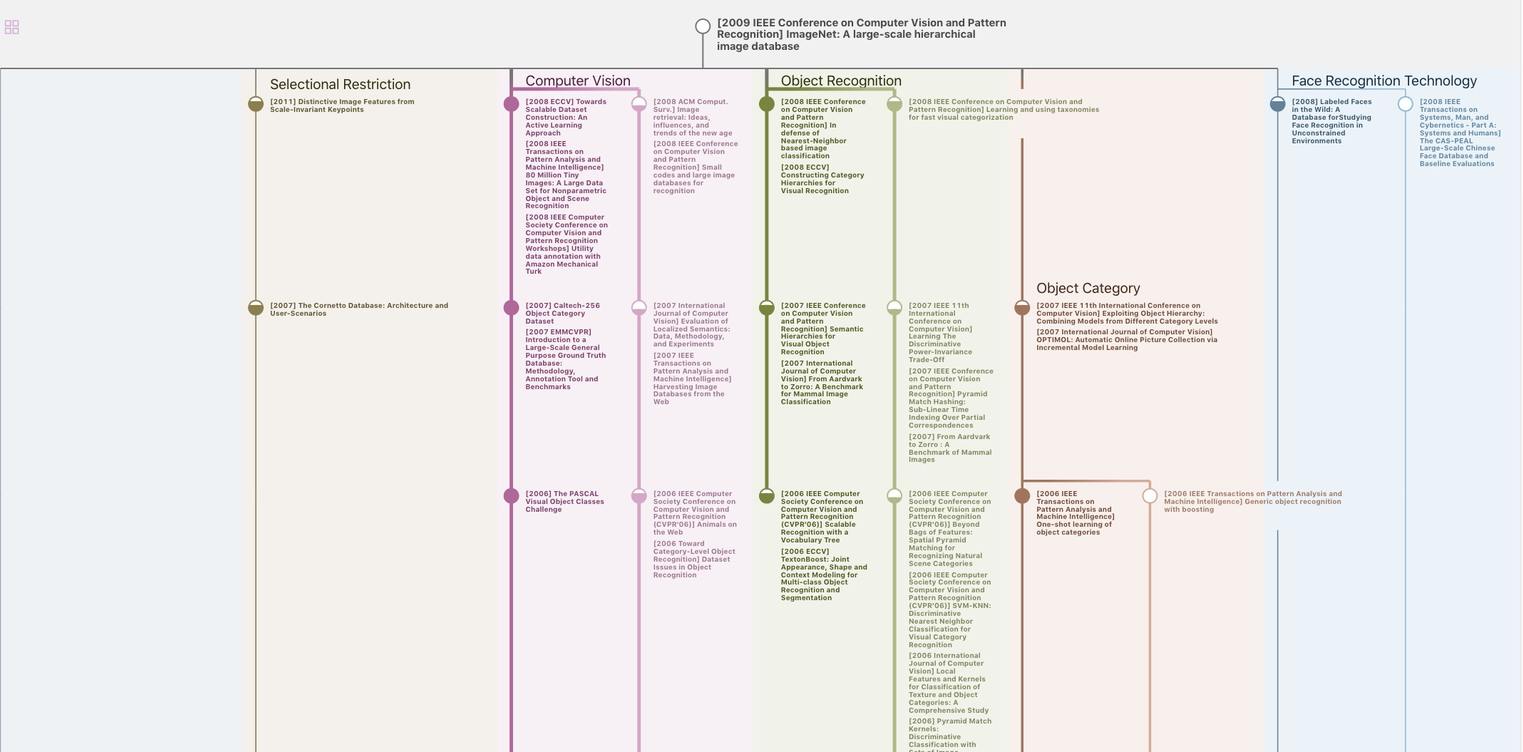
生成溯源树,研究论文发展脉络
Chat Paper
正在生成论文摘要