3D Molecular Geometry Analysis with 2D Graphs
arXiv (Cornell University)(2023)
摘要
Ground-state 3D geometries of molecules are essential for many molecular analysis tasks. Modern quantum mechanical methods can compute accurate 3D geometries but are computationally prohibitive. Currently, an efficient alternative to computing ground-state 3D molecular geometries from 2D graphs is lacking. Here, we propose a novel deep learning framework to predict 3D geometries from molecular graphs. To this end, we develop an equilibrium message passing neural network (EMPNN) to better capture ground-state geometries from molecular graphs. To provide a testbed for 3D molecular geometry analysis, we develop a benchmark that includes a large-scale molecular geometry dataset, data splits, and evaluation protocols. Experimental results show that EMPNN can efficiently predict more accurate ground-state 3D geometries than RDKit and other deep learning methods. Results also show that the proposed framework outperforms self-supervised learning methods on property prediction tasks.
更多查看译文
关键词
3d molecular geometry analysis,molecular geometry,2d graphs
AI 理解论文
溯源树
样例
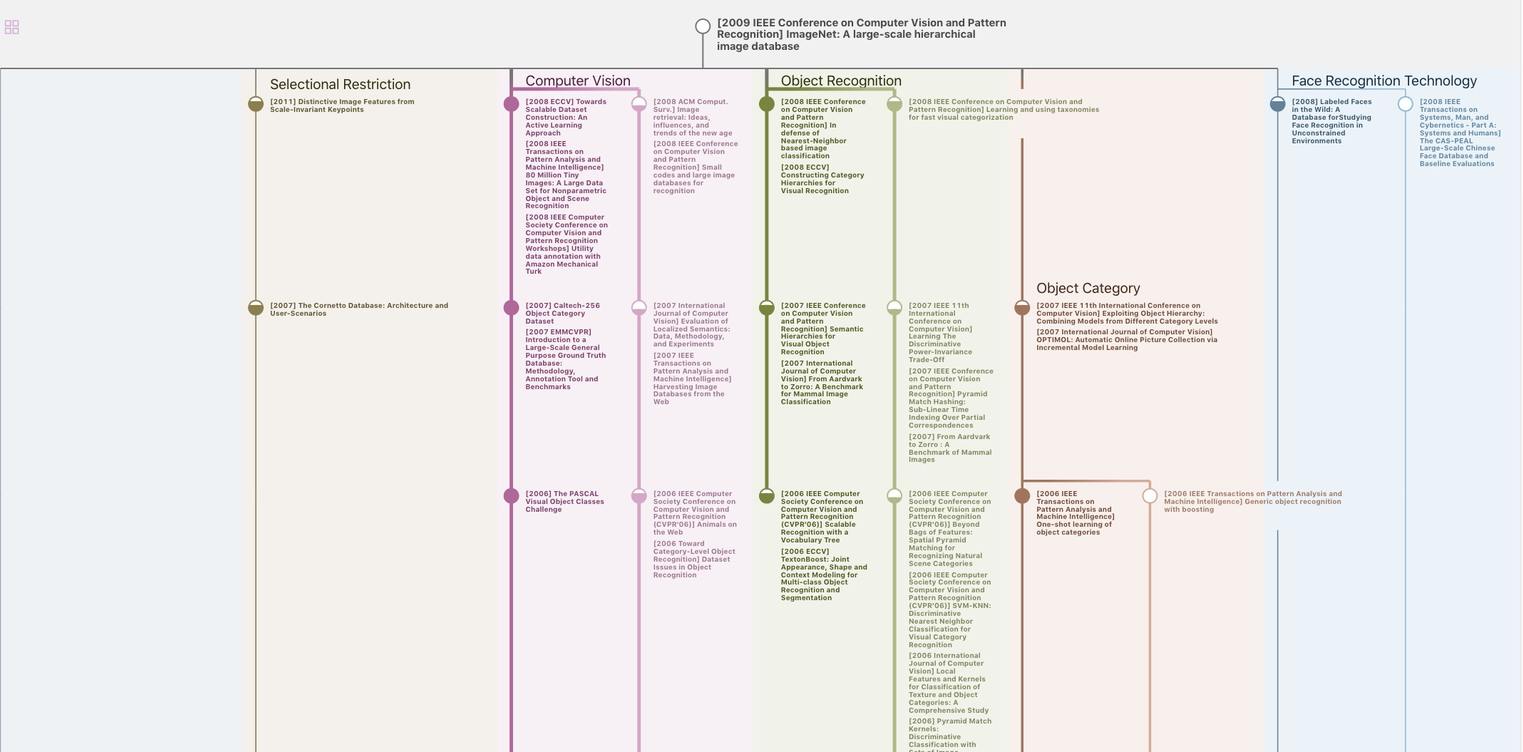
生成溯源树,研究论文发展脉络
Chat Paper
正在生成论文摘要