An analytic hierarchy process method weighted by an improved Random Forest model considering sample optimization selection for the evaluation of landslide susceptibility
Research Square (Research Square)(2023)
摘要
Abstract Landslides often cause great losses to people, so it is important to know the extent of landslide damage to reduce the impact of disasters. Although many scholars have conducted on landslide disaster susceptibility, there are still some issues, such as an unreasonable negative sample selection strategy and an absence of subjective environmental information of the study area in a single machine learning evaluation model. Therefore, an analytic hierarchy process (AHP) method weighted by an improved Random Forest (RF) model is proposed for evaluating landslide susceptibility based on sample optimization. On the basis of density analysis of landslide data, this method employs the specific factor method (CF) to generate negative sample data. The improved RF model based on adaptive boosting (ADB_RF) is proposed to obtain objective weights, which are then combined with the subjective weights obtained by AHP. Meanwhile, a case study of the evaluation of landslide disasters is conducted in the Chuxiong Autonomous Prefecture of Yunnan Province in China. The results show: (1) The landslide susceptibility evaluation method proposed in this study can objectively reflect the area prone to landslides with high accuracy and effectiveness. (2) The area under the line of the CF-combination model reached 96.1%, indicating a high degree of accuracy. (3) In the northwest of Chuxiong Prefecture, there are a greater number of extremely high-risk areas than in the southeast, the possibility of another landslide disaster is high, which needs to be focused. The research findings have significant reference value for preventing disasters and mitigating losses.
更多查看译文
关键词
analytic hierarchy process method,improved random forest model,sample optimization selection
AI 理解论文
溯源树
样例
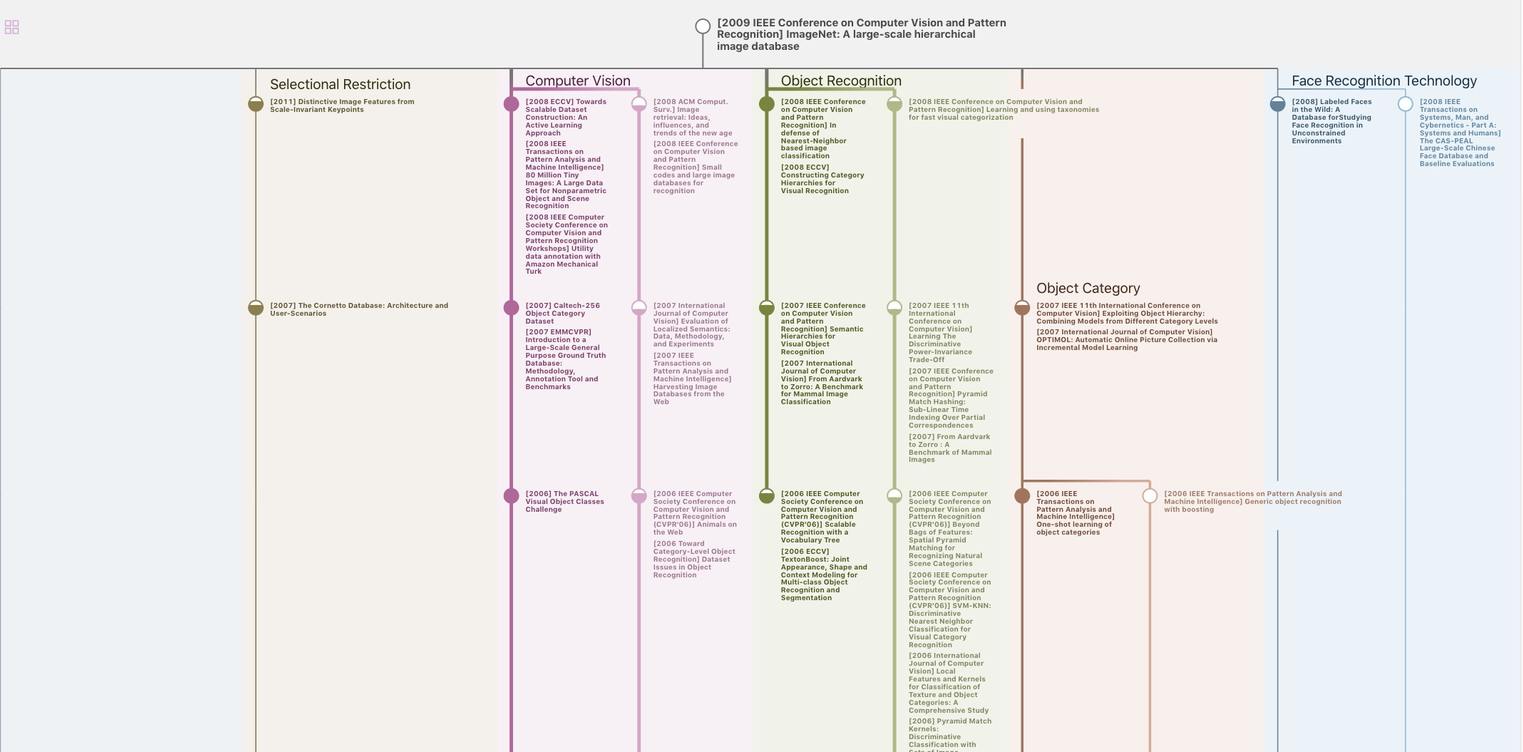
生成溯源树,研究论文发展脉络
Chat Paper
正在生成论文摘要