Identification of DNA-binding proteins by Kernel Sparse Representation via L 2,1 -matrix norm
Computers in Biology and Medicine(2023)
摘要
An understanding of DNA-binding proteins is helpful in exploring the role that proteins play in cell biology. Furthermore, the prediction of DNA-binding proteins is essential for the chemical modification and structural composition of DNA, and is of great importance in protein functional analysis and drug design. In recent years, DNA-binding protein prediction has typically used machine learning-based methods. The prediction accuracy of various classifiers has improved considerably, but researchers continue to spend time and effort on improving prediction performance. In this paper, we combine protein sequence evolutionary information with a classification method based on kernel sparse representation for the prediction of DNA-binding proteins, and based on the field of machine learning, a model for the identification of DNA-binding proteins by sequence information was finally proposed. Based on the confirmation of the final experimental results, we achieved good prediction accuracy on both the PDB1075 and PDB186 datasets. Our training result for cross-validation on PDB1075 was 81.37%, and our independent test result on PDB186 was 83.9%, both of which outperformed the other methods to some extent. Therefore, the proposed method in this paper is proven to be effective and feasible for predicting DNA-binding proteins.
更多查看译文
关键词
kernel sparse representation,proteins,dna-binding
AI 理解论文
溯源树
样例
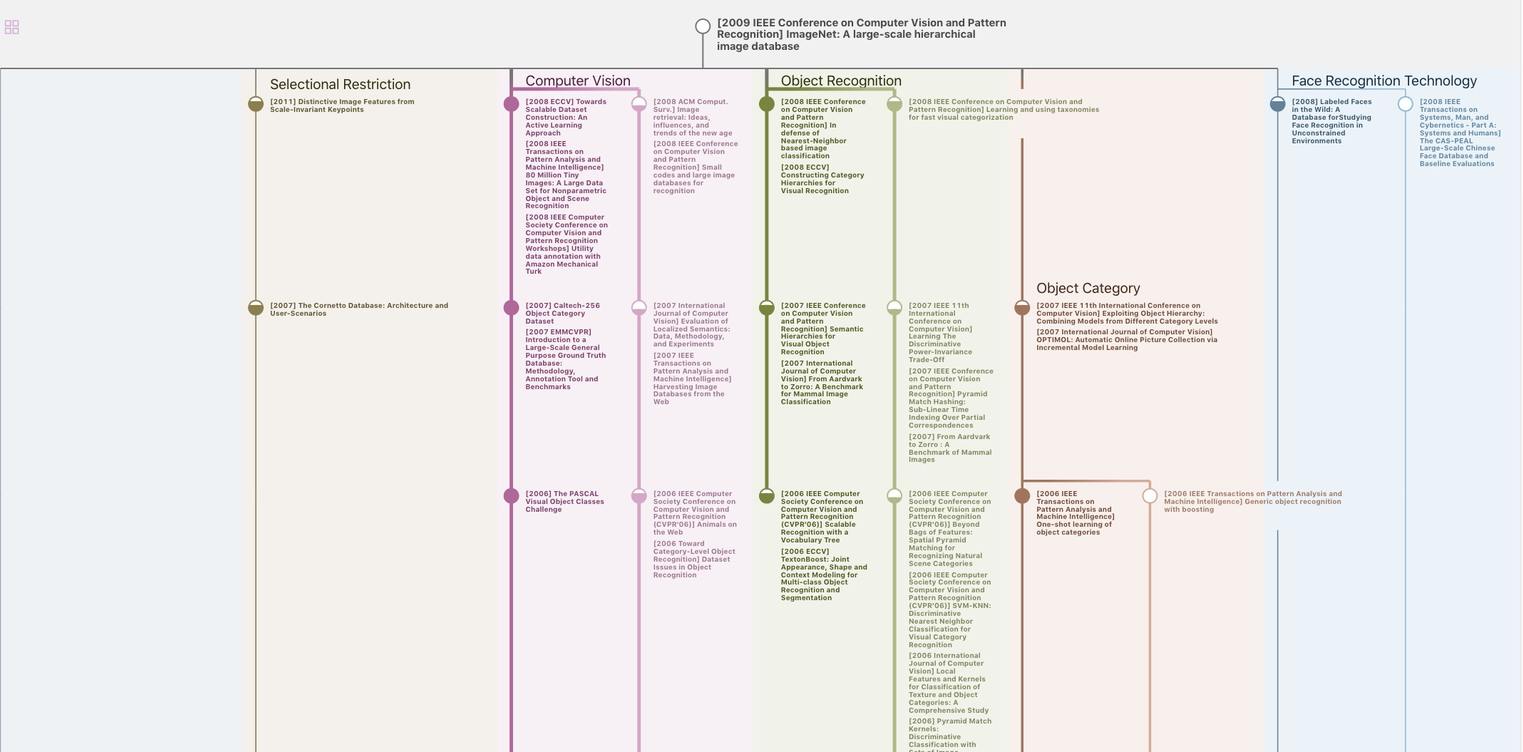
生成溯源树,研究论文发展脉络
Chat Paper
正在生成论文摘要