Abstract 5434: Machine-learning-based epigenetic detection of early-stage lung cancers using the EpiCheck liquid biopsy platform
Cancer Research(2023)
摘要
Abstract Alterations in DNA methylation are one of the earliest, most common signatures of cancer development, making them ideal biomarkers for early detection. Methylation profiling of plasma cell-free DNA (cfDNA) has significant potential to expand the use of liquid biopsies to cancer screening. The EpiCheck platform combines methylation-sensitive restriction endonuclease (MSRE) digestion with whole genome sequencing to comprehensively map genome-wide DNA methylation changes with high fidelity. In this proof-of-concept study, MSRE-NGS was used to interrogate a liquid biopsy atlas focused on early-stage lung cancers to i) identify informative NGS methylation biomarkers in plasma, and ii) develop a machine learning method to discriminate between cancer and high-risk, non-cancer controls. The EpiCheck lung cancer atlas was constructed using biospecimens from academic (UBC, Vanderbilt, Cleveland Clinic) and commercial biobanks, and from a prospective multi-center study (NCT04968548). Plasma samples were collected from 93 high-risk primary lung cancer patients (62% stage I) and 90 high-risk individuals without cancer. Extracted DNA was digested with methylation-sensitive endonucleases and sequenced at an average depth of 600x. Methylation levels of ~6 million genomic loci were rank ordered using Student's t-test. Gene set enrichment analysis (GSEA) was performed on the top ranking 1000 differentially methylated loci. A logistic regression classifier with Lasso regularization was trained on 100,000 loci, and performance was examined by mean AUC using 5-fold cross-validation. A total of 23,130 loci exhibited significant differential methylation patterns between cancers and controls (p<0.01, FDR corrected). Of these, 20,159 and 2,971 lung cancer loci were hypermethylated and hypomethylated, respectively. Biological characterization using GSEA identified enrichments in transcriptional regulation and developmental control. In particular, loci were enriched for Polycomb Repressive Complex regulated genes, suggesting a possible connection to abnormal epigenetic regulation via histone modification in lung cancer. Construction of a machine learning logistic regression model based on the five training folds utilized 224 loci on average, and achieved a mean cross-validation AUC of 0.93 when distinguishing plasma cancer cases vs controls. Our findings demonstrate that the MSRE-NGS EpiCheck platform identified putative biomarkers within the plasma methylome for detecting early-stage lung cancer. A machine learning model trained on methylation targets performed with high accuracy in discriminating lung cancer patients from high-risk healthy individuals. Additional studies are required for defining the strength of the approach and for validating its use in non-invasive lung cancer screening. Citation Format: Dvir Netanely, Stephen Lam, Anna McGuire, Stephen Deppen, Eric Grogan, Fabien Maldonado, Michael Gieske, Joseph Seaman, Kimberly Rieger-Christ, Satish Kalanjeri, Luis Herrera, Nichole Tanner, Garrett B. Sherwood, Orna Savin, Shacade Danan, Sarah Zaouch, Nimrod Axelrad, Revital Knirsh, Ofir Shliefer, Keren Manor, Radha Duttagupta, Aharona Shuali, Peter J. Mazzone, Gerard A. Silvestri, Catherine A. Schnabel, Danny Frumkin, Adam Wasserstrom. Machine-learning-based epigenetic detection of early-stage lung cancers using the EpiCheck liquid biopsy platform. [abstract]. In: Proceedings of the American Association for Cancer Research Annual Meeting 2023; Part 1 (Regular and Invited Abstracts); 2023 Apr 14-19; Orlando, FL. Philadelphia (PA): AACR; Cancer Res 2023;83(7_Suppl):Abstract nr 5434.
更多查看译文
关键词
epigenetic detection,machine-learning-based machine-learning-based,early-stage
AI 理解论文
溯源树
样例
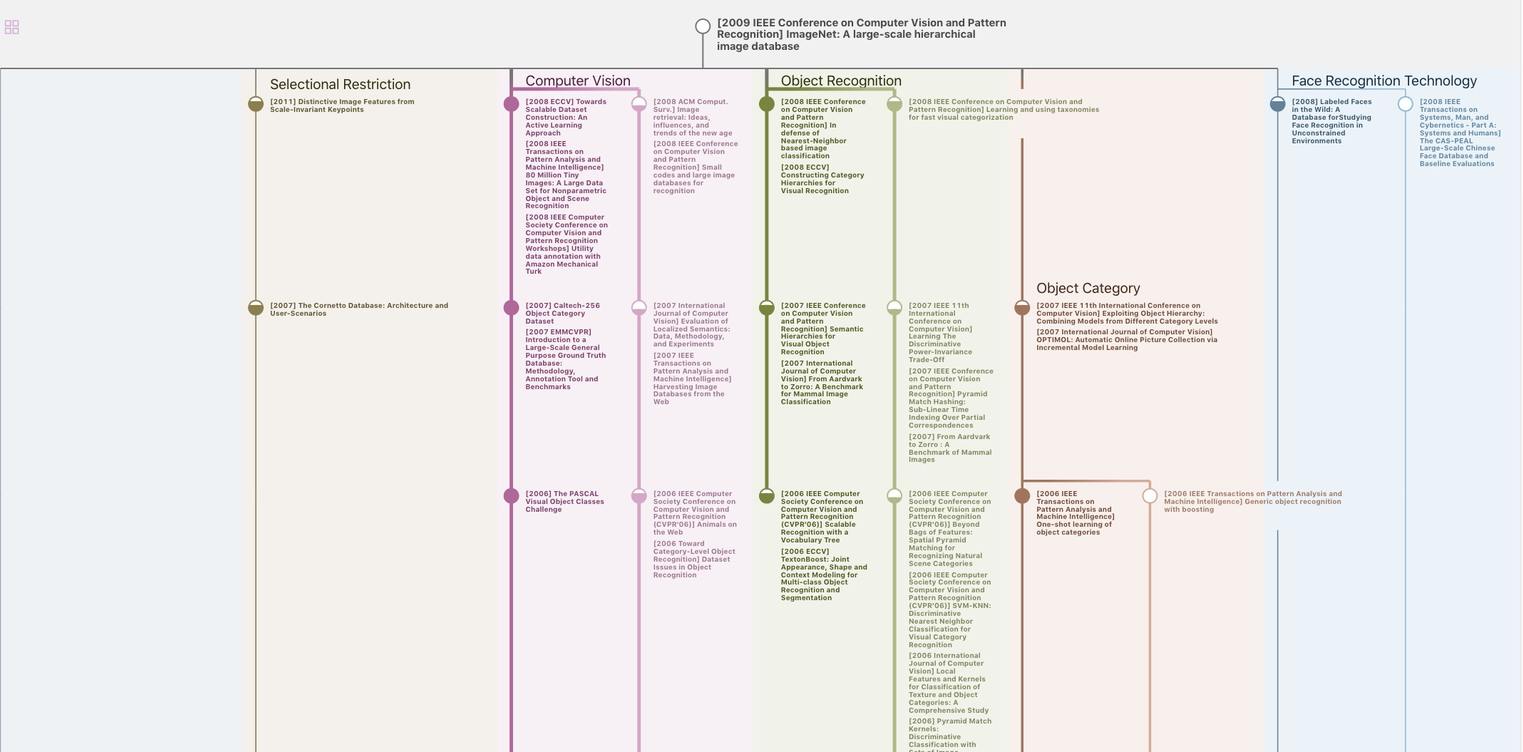
生成溯源树,研究论文发展脉络
Chat Paper
正在生成论文摘要