GNN-Based Meta-Learning Approach for Adaptive Power Control in Dynamic D2D Communications.
IEEE Trans. Veh. Technol.(2024)
摘要
This paper proposes a deep learning-based power control method for maximizing the sum rate subject to rate requirements in the interference-limited device-to-device (D2D) communications. Based on the dynamic nature of D2D communications, we consider the environment where system parameters, such as the number of devices, rate requirements, and deployment area, unpredictably change over time. To deal with the low adaptability and scalability issues of the conventional deep learning-based approaches in dynamic environments, we develop an environment-adaptive power control method by leveraging graph neural network (GNN) architecture and meta-learning approach. In the developed method, we design the node feature and state update rule for GNN by taking into account the characteristics of power optimization problem and meta-train model by treating some past environments as meta-tasks. Simulation results demonstrate that the developed method outperforms the conventional GNN-based power control methods in terms of the average sum-throughput achievement ratio and adaptation speed to test environments.
更多查看译文
关键词
Interference management,power control,meta learning,graph neural network,D2D communications
AI 理解论文
溯源树
样例
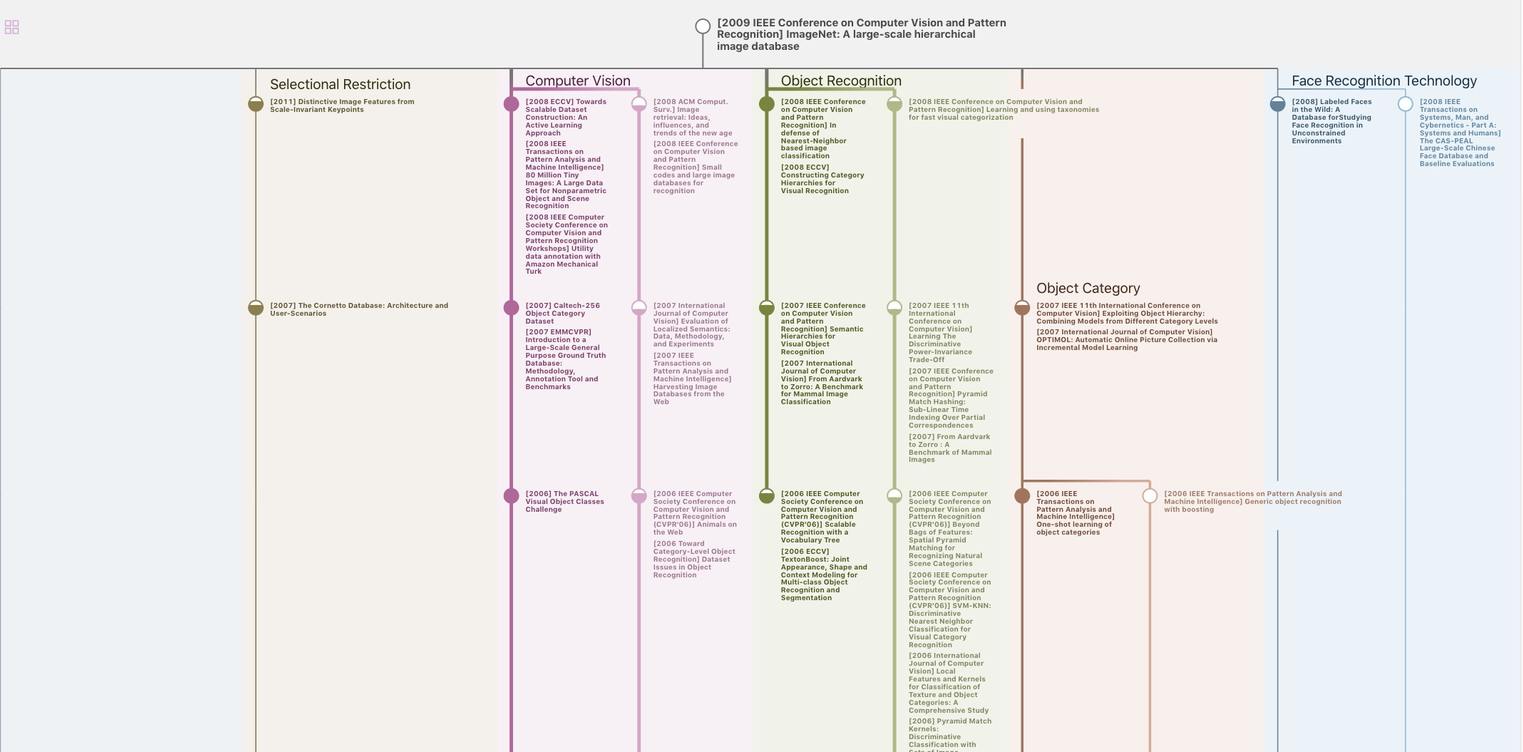
生成溯源树,研究论文发展脉络
Chat Paper
正在生成论文摘要