INDM: Chiplet-Based Interconnect Network and Dataflow Mapping for DNN Accelerators
IEEE TRANSACTIONS ON COMPUTER-AIDED DESIGN OF INTEGRATED CIRCUITS AND SYSTEMS(2024)
摘要
Chiplet-based deep neural network (DNN) accelerator is a promising solution to balance the performance and manufacturing cost. However, different from monolithic chips, interconnect network design and architectural partitioning for multiple chiplets would result in a huge design space and make it difficult to keep scalability and high hardware utilization. Moreover, how to efficiently map DNN workloads onto multiple DRAM dies and compute dies is another major challenge. To alleviate the above issues, in this work, we propose INDM, a chiplet-based interconnect network and dataflow mapping co-optimization for DNN accelerators. First, we propose an efficient hierarchical interconnect network composed of a multiring on-die network and a cluster-based interdie network, to facilitate the data reuse and traffic pattern in DNN workloads. Second, architectural partitioning and topology exploration for chiplet-based DNN accelerators are proposed to find the optimal architecture configurations. Third, an interdie communication-aware dataflow mapping is proposed to minimize traffic congestion during DNN layer switching. We implement the proposed chiplet-based interconnect network design and dataflow mapping algorithm for a set of popular DNN models, including VGG-16, ResNet-18, DarkNet-19, ResNet-50, and ResNet-101. Experimental results show that as compared with the state-of-the-art related work, such as NN-Baton and SIMBA, our work achieves 26.00%-73.81% energy-delay-product (EDP) reduction and 26.93%-79.78% latency reduction.
更多查看译文
关键词
Chiplet,dataflow mapping,deep neural network (DNN) accelerator,interconnect
AI 理解论文
溯源树
样例
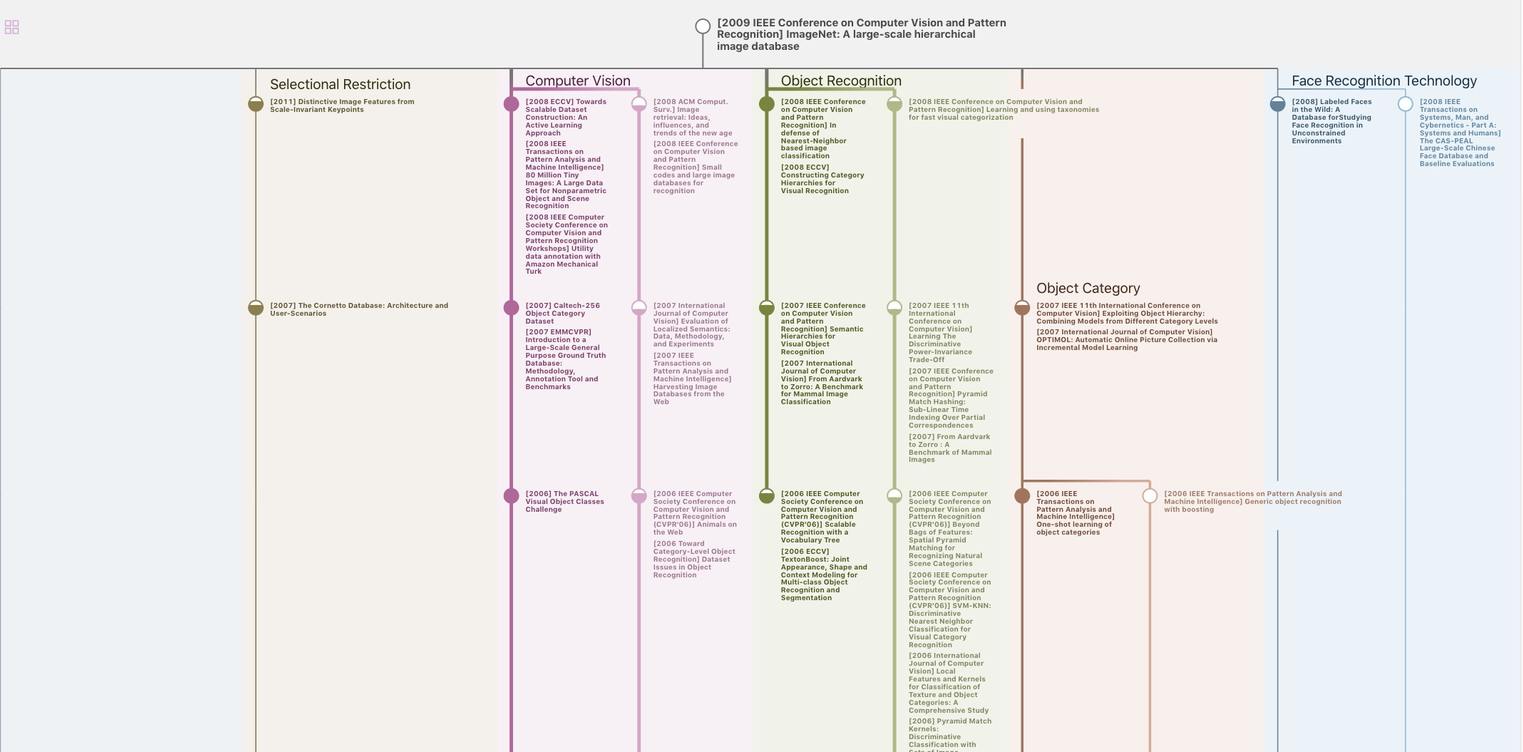
生成溯源树,研究论文发展脉络
Chat Paper
正在生成论文摘要