Deep learning in hydrology and water resources disciplines: concepts, methods, applications, and research directions
JOURNAL OF HYDROLOGY(2024)
摘要
Over the past few years, Deep Learning (DL) methods have garnered substantial recognition within the field of hydrology and water resources applications. Beginning with a discussion on fundamental concepts of DL, we discussed the state-of-the-art DL architectures such as Long -Short -Term -Memory (LSTM), Convolutional Neural Networks (CNN), Generative Adversarial Networks (GAN), and Encoder -Decoder models that have gained much attention over the recent years. The recent advancements in the DL model, such as the Attention model and Transformer Neural Network, that are designed to handle sequential time series data, are also discussed. An overview of integrating physics -based hydrological models with state-of-the-art DL models, known as PhysicsGuided Deep Learning (PGDL), and its potential for improving the accuracy and interpretability of hydrological predictions are discussed. We emphasized that PGDL has the potential to enhance the physical consistency and robustness of the hydrologic predictions. We further delve into Explainable Artificial Intelligence (XAI), examining various techniques for constructing interpretable models. The objective is to empower users to comprehend and confidently trust machine learning algorithms' results (model outputs). Furthermore, we delved into the diverse applications of Deep Learning (DL) in hydrology and water resources sectors, encompassing areas such as drought and flood forecasting, remote sensing applications, water quality assessments, subsurface flow inversion problems, groundwater level prediction, and hydro -climate variable downscaling.
更多查看译文
关键词
Deep learning,Hydrology,Water resources,Physics guided deep learning,Explainable Artificial Intelligence (XAI)
AI 理解论文
溯源树
样例
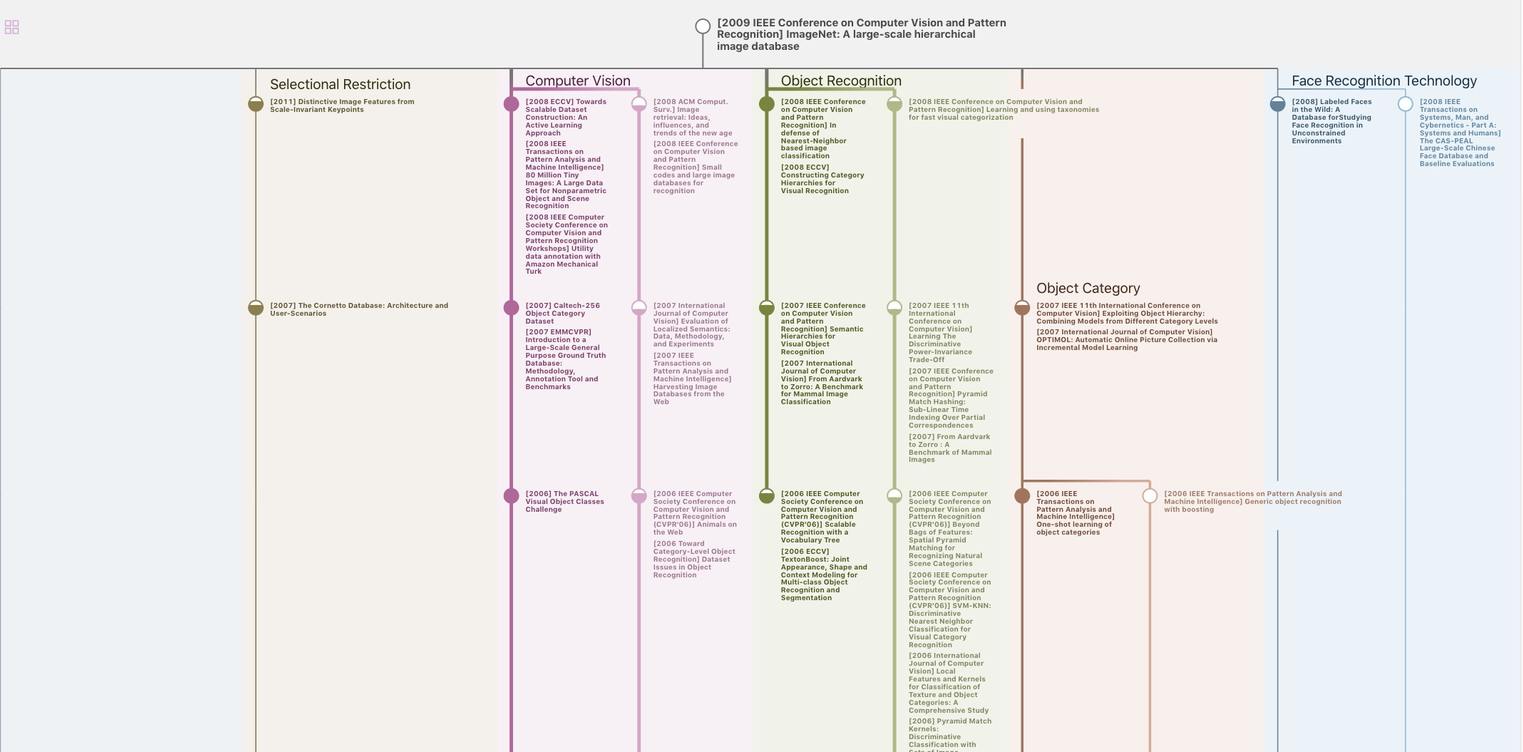
生成溯源树,研究论文发展脉络
Chat Paper
正在生成论文摘要