Steerable Graph Neural Network on Point Clouds via Second-Order Random Walks
IEEE Transactions on Multimedia(2023)
摘要
Point cloud analysis, arising from computer graphics, remains a fundamental but challenging problem, mainly due to the non-Euclidean property of point cloud data modality. With the snap increase in the amount and breadth of related research in deep learning for graphs, many important works come in the form of graphs representing the point clouds. In this paper, we present a sampling adaptive graph convolutional network that combines the powerful representation ability of random walk subgraph searching and the essential success of the Fisher vector. Extending from those existing graph representation learning or embedding methods with multi-hop neighbor random searching, we sample multi-scale walk fields by using a
steerable
exploration-exploitation
second order random walk
, which endows our model with the most flexibility compared with the original first order random walk. To encode each-scale walk field consisting of several walk paths, specifically, we characterize these paths of walk field by Gaussian mixture models (GMMs) so as to better analogize the standard CNNs on Euclidean modality. Each Gaussian component implicitly defines a direction and all of them properly encode the
spatial layout
of walk fields after the gradient projecting to the space of Gaussian parameters, i.e. the Fisher vectors. Thereby, we introduce and name our deep graph convolutional network as
PointFisher
. Comprehensive evaluations on several public datasets well demonstrate the superiority of our proposed learning method over other state-of-the-arts for point cloud classification and segmentation.
更多查看译文
关键词
Second order random walk,steerable graph neural network,Gaussian mixture models,Fisher vectors
AI 理解论文
溯源树
样例
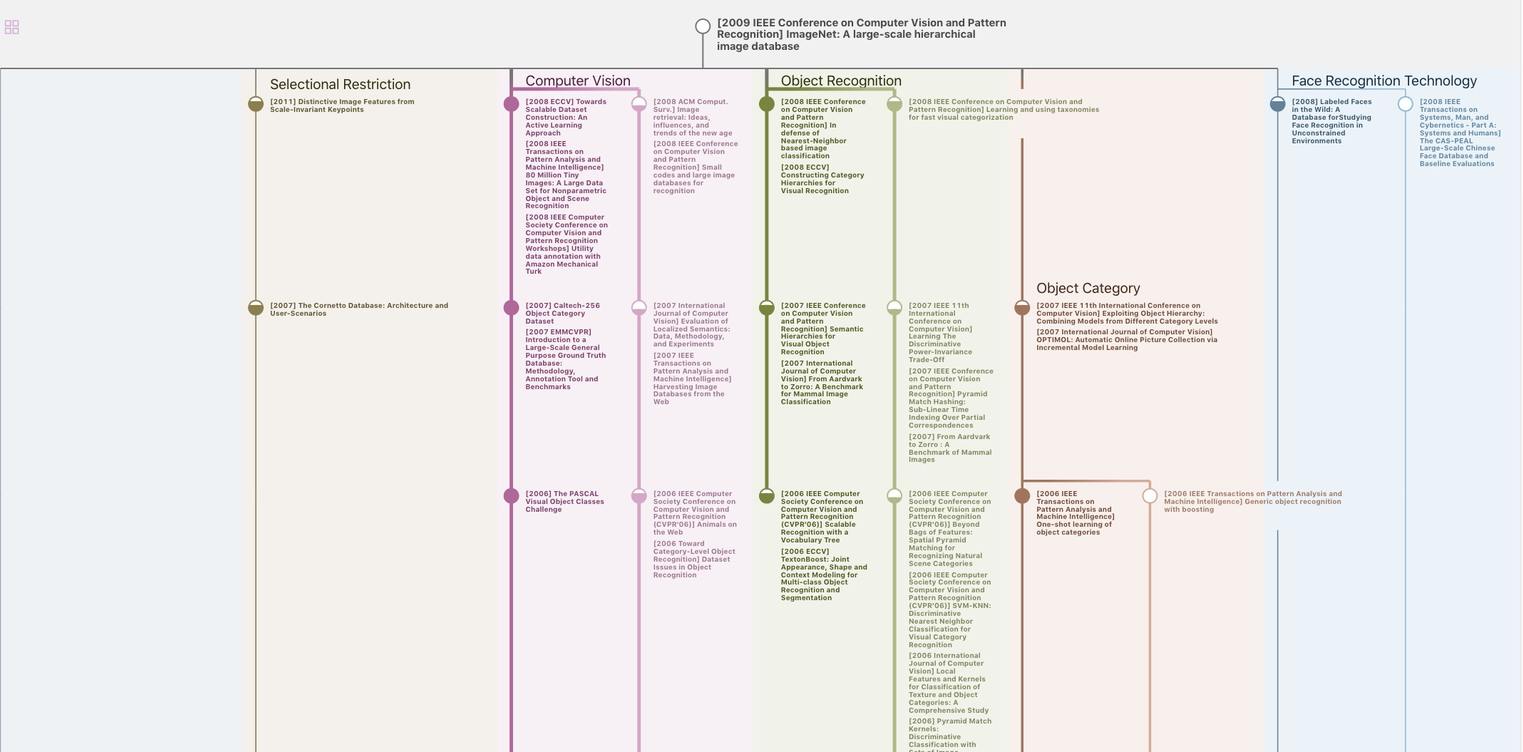
生成溯源树,研究论文发展脉络
Chat Paper
正在生成论文摘要