Swarm Learning and Knowledge Distillation Empowered Self-Driving Detection against Threat Behavior for Intelligent IoT
IEEE Transactions on Mobile Computing(2023)
摘要
The combination of mobile communication and the Internet of Things (IoT) has made physical devices more intelligent, bringing great convenience to our lives. However, the deep integration of personal information and the Internet increases the risk of data leakage and is easily exploited maliciously. In addition, due to limited system resources, smart devices with lightweight design are required. Therefore, it is necessary to realize low-energy and effective abnormal behavior detection of IoT devices, but the existing detection methods have disadvantages such as leakage of user privacy, low accuracy, and difficulty in dynamically improving the effect. To address these issues, this paper proposes a dynamic interactive minor anomaly detection scheme called ADONIS based on Swarm Learning (SL). The scheme combines the concept of swarm defense and utilizes SL to achieve local data fusion, which improves the detection effect and protects user privacy. Moreover, the decentralized structure of SL can cope with the impact of single node damage to enhance the robustness of IoT services. Furthermore, we propose training and detection decoupling framework to achieve high accuracy, low energy consumption, and low latency. It improves the performance by fitting the training model with full data, and simplifies the complexity of the detection model using knowledge distillation. We also design a self-enhancing dynamic strategy based on the decoupling framework to maintain powerful detection capability through human-computer interaction (HCI) and continuous learning. The framework relies on traffic data to keep the model sensitive to new behavior through iterative training without disturbing the user. Finally, simulation experiments show that our proposed scheme can achieve 82.2% accuracy, reduce the average detection time to 8.22
$ ms$
, and simplify the model complexity by 15.9%. Compared with existing methods, ADONIS can provide lighter, safer and more accurate anomaly detection.
更多查看译文
关键词
IoT,network abnormal detection,human-computer interaction,swarm defense,training-detection decoupling
AI 理解论文
溯源树
样例
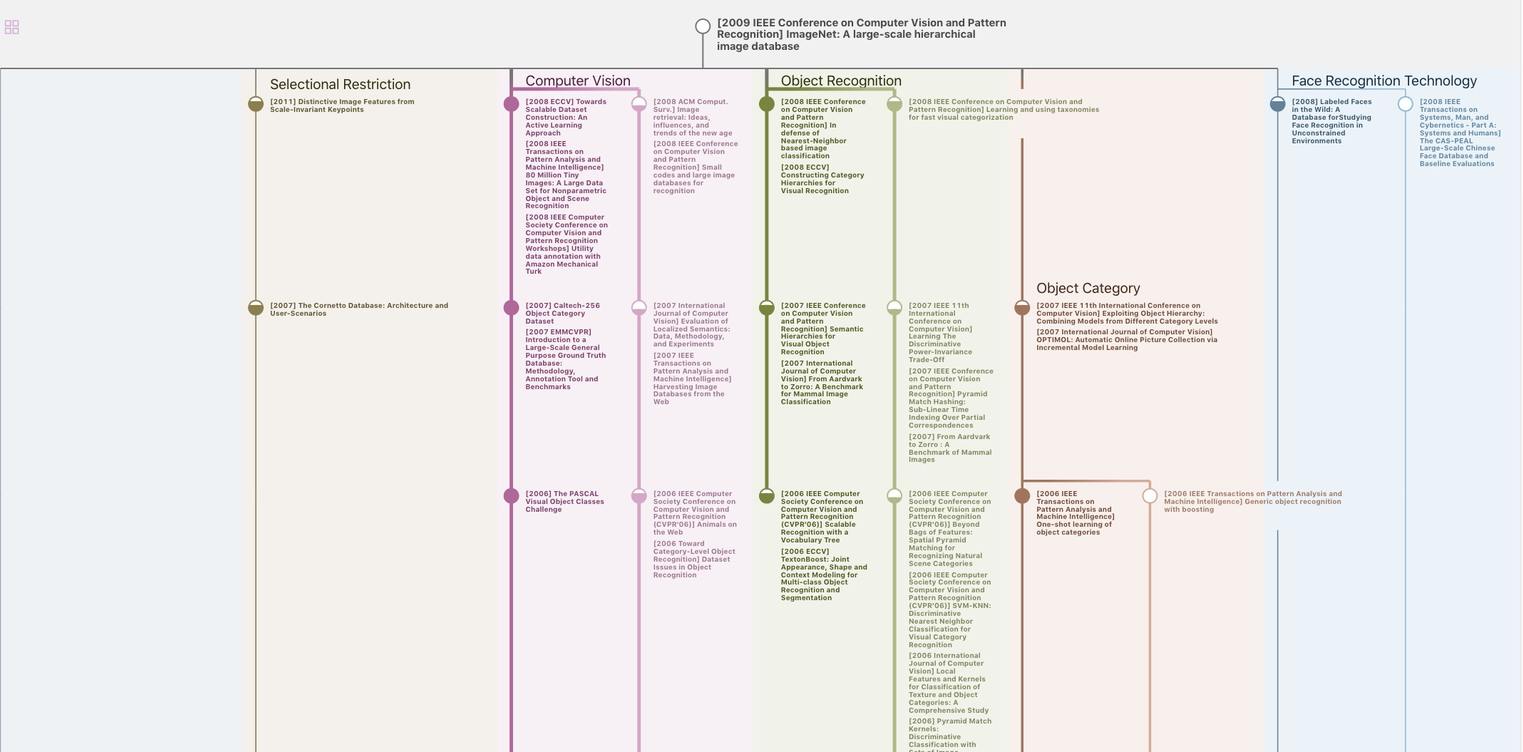
生成溯源树,研究论文发展脉络
Chat Paper
正在生成论文摘要