A Probabilistic Framework for Online Analysis of Alarm Floods Using Convolutional Neural Networks
IEEE TRANSACTIONS ON INSTRUMENTATION AND MEASUREMENT(2024)
摘要
Safe and efficient operation of complex industrial processes is highly dependent on alarm systems. There is a phenomenon called alarm flood, which significantly impairs the performance of alarm systems. An alarm flood refers to a situation when a plant operator is overloaded with many alarms triggered sequentially within a short time period. As a result, the operator can be distracted from taking the required safety actions. It is, therefore, imperative to deal with the problem of alarm floods, particularly for online applications. By taking advantage of historical alarm and event (A&E) logs, efficient alarm flood monitoring methods based on similarity analysis can be developed in the area of machine learning (ML). In this article, we develop a probabilistic framework for transforming alarm flood data into suitable inputs for convolutional neural networks (CNNs). The proposed alarm flood representation is used to establish an ML-based alarm flood analysis framework, where a CNN is trained to predict upcoming fault scenarios by observing online alarm floods. This method is capable of incorporating the activated alarm tags and their triggering times, as well as tolerating the effect of irrelevant alarms and alarm order ambiguities. Two alarm datasets from the Tennessee Eastman (TE) benchmark and a Vinyl Acetate Monomer (VAM) process are used to evaluate the proposed approach.
更多查看译文
关键词
Alarm floods,convolutional neural networks (CNNs),early operator assistance,machine learning (ML),online classification,root cause diagnosis
AI 理解论文
溯源树
样例
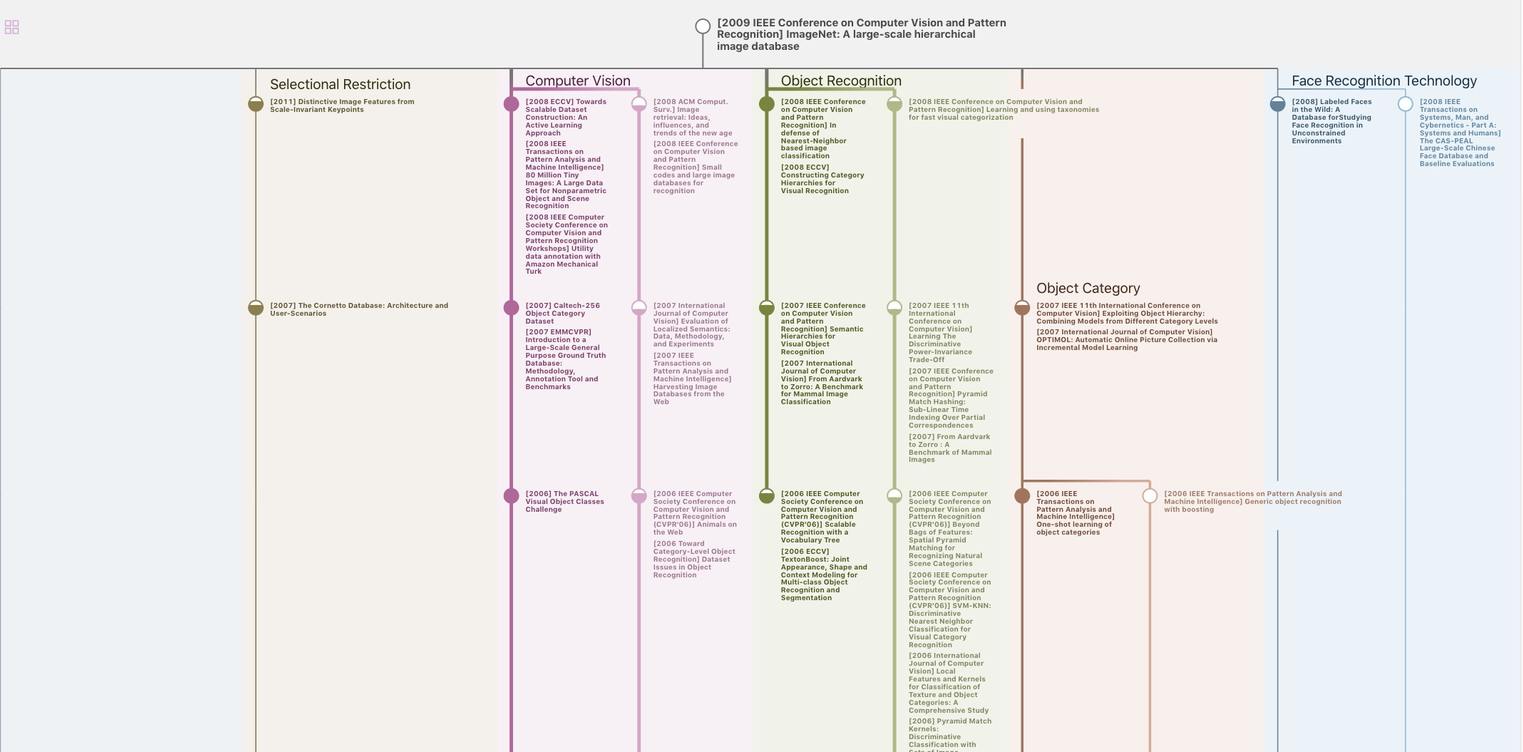
生成溯源树,研究论文发展脉络
Chat Paper
正在生成论文摘要