Testing General Linear Hypotheses Under a High-Dimensional Multivariate Regression Model with Spiked Noise Covariance
JOURNAL OF THE AMERICAN STATISTICAL ASSOCIATION(2024)
摘要
We consider the problem of testing linear hypotheses under a multivariate regression model with a high-dimensional response and spiked noise covariance. The proposed family of tests consists of test statistics based on a weighted sum of projections of the data onto the estimated latent factor directions, with the weights acting as the regularization parameters. We establish asymptotic normality of the test statistics under the null hypothesis. We also establish the power characteristics of the tests and propose a data-driven choice of the regularization parameters under a family of local alternatives. The performance of the proposed tests is evaluated through a simulation study. Finally, the proposed tests are applied to the Human Connectome Project data to test for the presence of associations between volumetric measurements of human brain and behavioral variables. Supplementary materials for this article are available online including a standardized description of the materials available for reproducing the work.
更多查看译文
关键词
Factor models,Local alternatives,Random matrix theory,Regularized tests
AI 理解论文
溯源树
样例
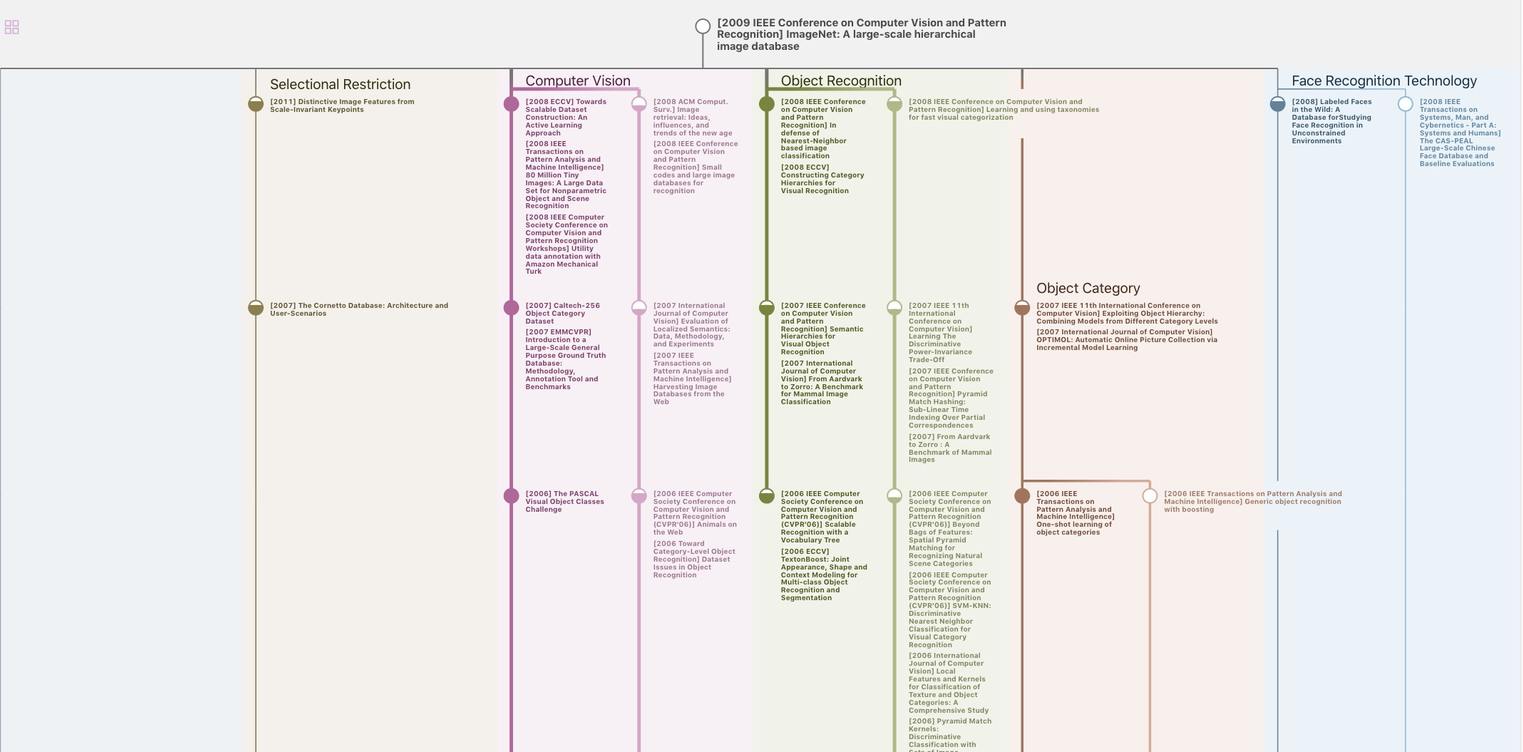
生成溯源树,研究论文发展脉络
Chat Paper
正在生成论文摘要