Research on Improved Method based on YOLOV5s Target Detection Model
Frontiers in Computing and Intelligent Systems(2023)
摘要
Aiming at the problem of low detection accuracy of small targets, an object detection method based on average pooling improved YOLOV5s model is proposed. The algorithm introduces the Squeeze Excitation attention module and the Efficient Intersection Over Union loss function to comprehensively improve the detection calculation efficiency and accurate deployment ability. With the development of deep learning technology, which is of great significance to improve the detection accuracy and detection rate. YOLO greatly improves detection performance, three times faster than retinanet and 2 times faster than faster-rcnn. YOLO has strong generalization ability, can be applied to different application scenarios, and is also easy to deploy. The steel surface defect public dataset was selected for verification. The results show that the improved YOLOV5s model is better than the original YOLOV5s model, the test average accuracy mAP can reach 81.8%, the average accuracy mAP of the model is increased by 7.4%, and the overall performance of the improved model is better than other conventional models.
更多查看译文
关键词
detection,target
AI 理解论文
溯源树
样例
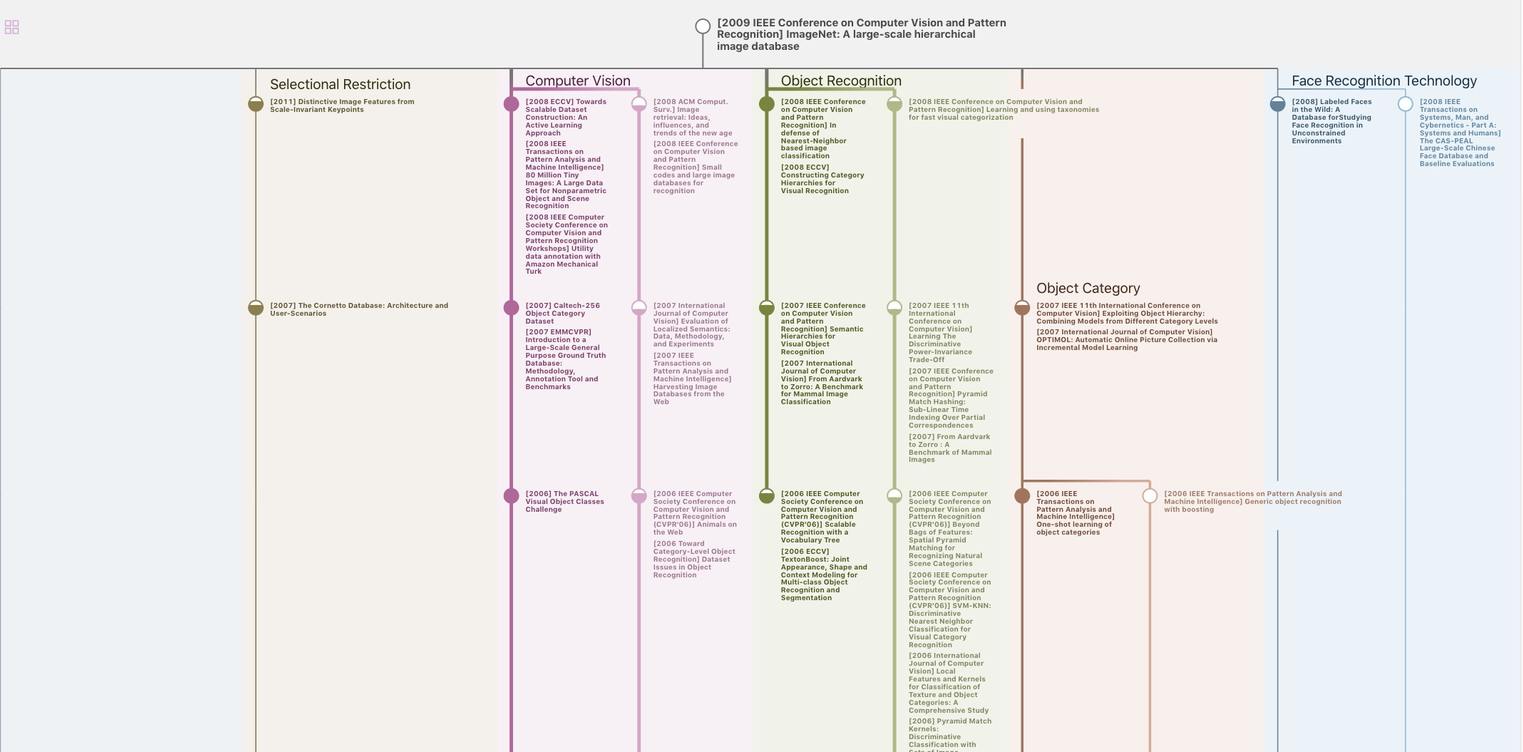
生成溯源树,研究论文发展脉络
Chat Paper
正在生成论文摘要