Predicting Wearing-Off Episodes in Parkinson’s with Multimodal Machine Learning
SpringerBriefs in computer science(2023)
摘要
This chapter addresses the significant concern of wearing-off episodes in patients with Parkinson. These episodes occur when the effects of medication, like levodopa, wear off, resulting in the recurrence of PD symptoms. The methodology harnesses features derived from heart rate, stress levels, sleep patterns, and step counts, combined with machine learning techniques, to forecast wearing-off in patients. Relevant features encompass mean condition, sleep-related factors, and time-related aspects. The study conducts experiments employing five different machine learning algorithms for all participants, seeking a personalized approach. Among the classifiers tested, the XGBoost classifier excels, achieving a 0.43 F1-score and 0.72 balanced accuracy. This methodology presents a promising avenue for automated, sensor-based detection of medication wearing off in PD patients, offering valuable insights into timely intervention and enhanced management strategies.
更多查看译文
关键词
parkinsons,predicting,machine learning,wearing-off
AI 理解论文
溯源树
样例
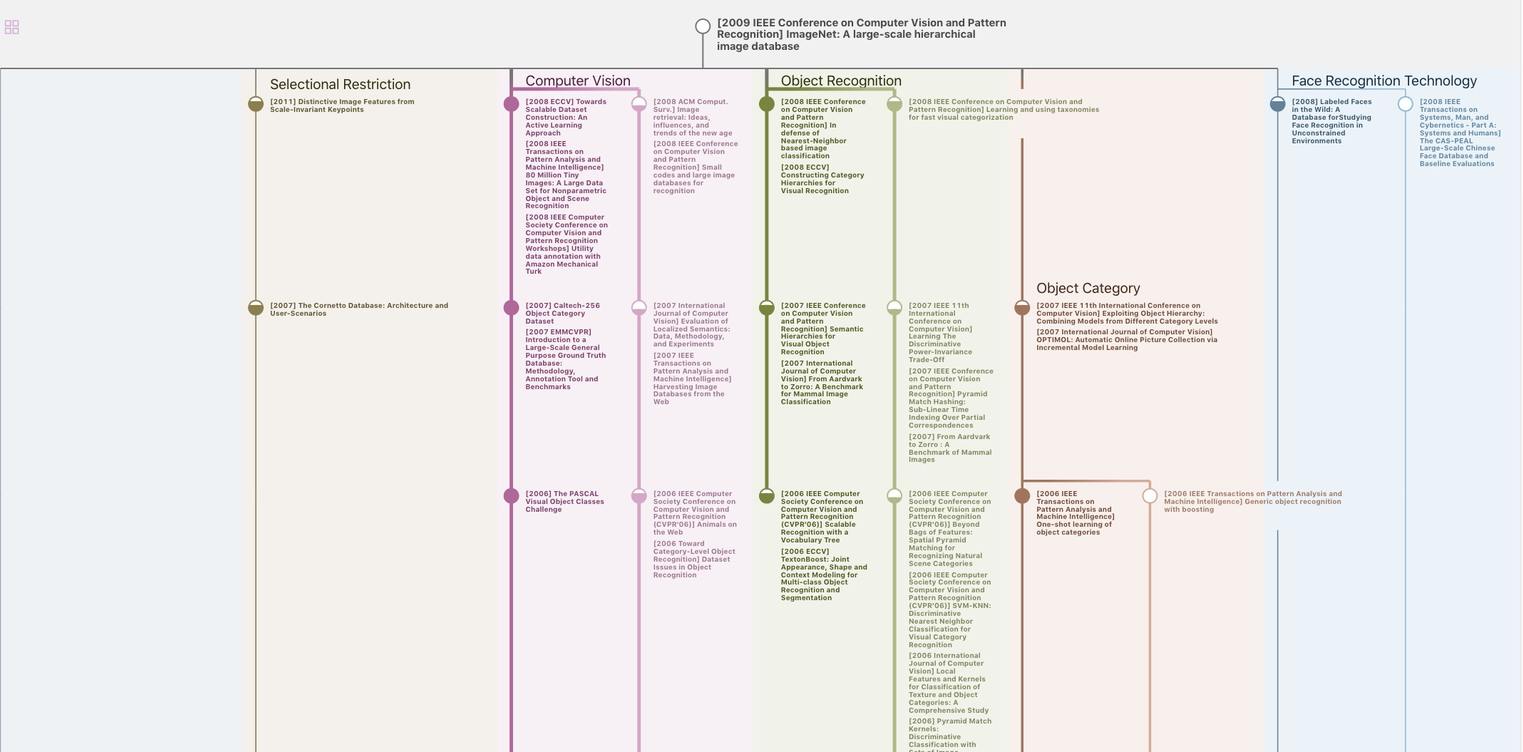
生成溯源树,研究论文发展脉络
Chat Paper
正在生成论文摘要