Orthogonal Subspace Learning for Language Model Continual Learning
arXiv (Cornell University)(2023)
摘要
Benefiting from massive corpora and advanced hardware, large language models (LLMs) exhibit remarkable capabilities in language understanding and generation. However, their performance degrades in scenarios where multiple tasks are encountered sequentially, also known as catastrophic forgetting. In this paper, we propose orthogonal low-rank adaptation (O-LoRA), a simple and efficient approach for continual learning in language models, effectively mitigating catastrophic forgetting while learning new tasks. Specifically, O-LoRA learns tasks in different (low-rank) vector subspaces that are kept orthogonal to each other in order to minimize interference. Our method induces only marginal additional parameter costs and requires no user data storage for replay. Experimental results on continual learning benchmarks show that our method outperforms state-of-the-art methods. Furthermore, compared to previous approaches, our method excels in preserving the generalization ability of LLMs on unseen tasks.
更多查看译文
关键词
language model continual learning,orthogonal subspace learning,continual learning
AI 理解论文
溯源树
样例
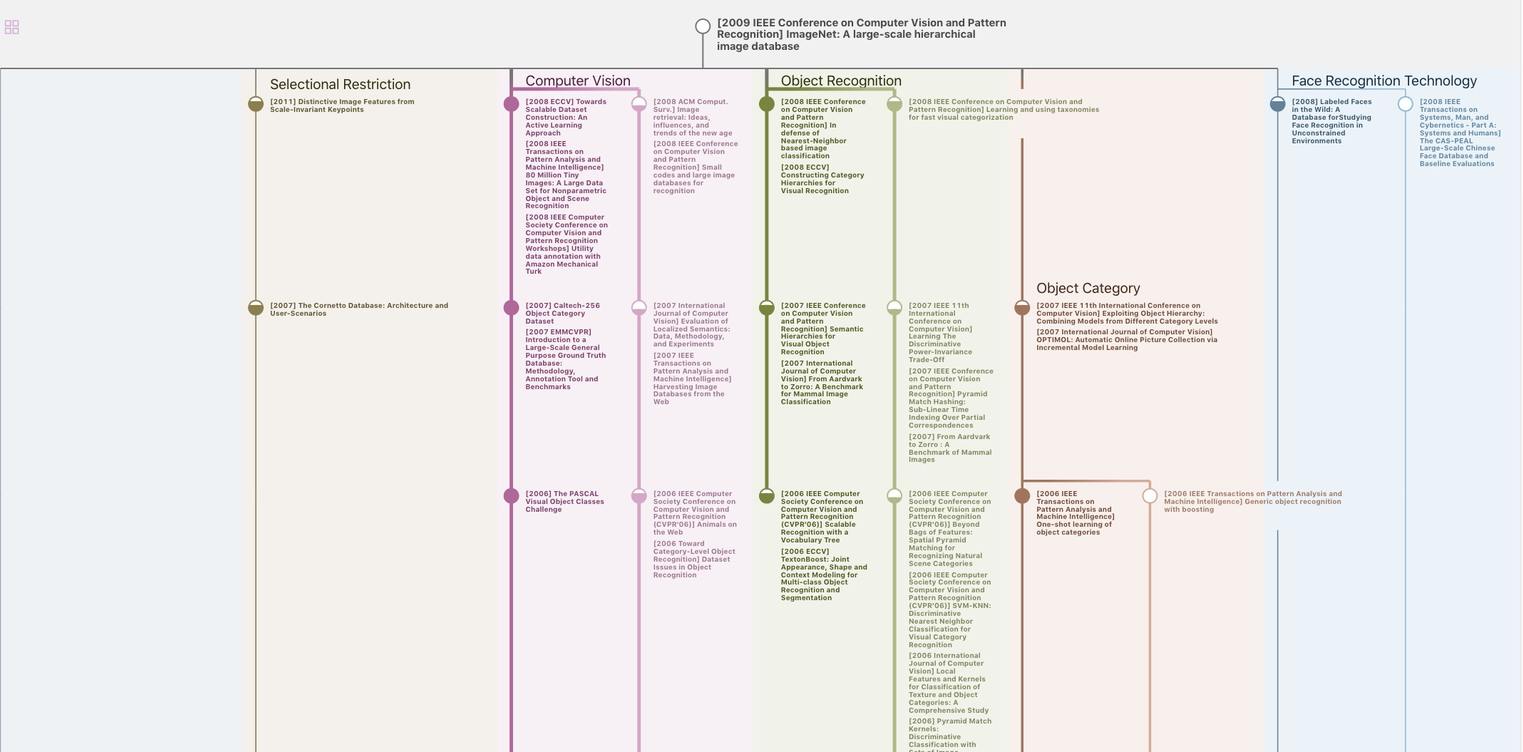
生成溯源树,研究论文发展脉络
Chat Paper
正在生成论文摘要