On Calibration of Scene-Text Recognition Models
Lecture Notes in Computer Science(2023)
摘要
The topics of confidence and trust in modern scene-text recognition (STR) models have been rarely investigated in spite of their prevalent use within critical user-facing applications. We analyze confidence estimation for STR models and find that they tend towards overconfidence thus leading to overestimation of trust in the predicted outcome by users. To overcome this phenomenon we propose a word-level confidence calibration approach. Initially, we adapt existing single-output T-scaling calibration methodologies to suit the case of sequential decoding. Interestingly, extensive experimentation reveals that character-level calibration underperforms word-level calibration and it may even be harmful when employing conditional decoding. In addition, we propose a novel calibration metric better suited for sequential outputs as well as a variant of T-scaling specifically designed for sequential prediction. Finally, we demonstrate that our calibration approach consistently improves prediction accuracy relative to the non-calibrated baseline when employing a beam-search strategy.
更多查看译文
关键词
calibration,recognition,models,scene-text
AI 理解论文
溯源树
样例
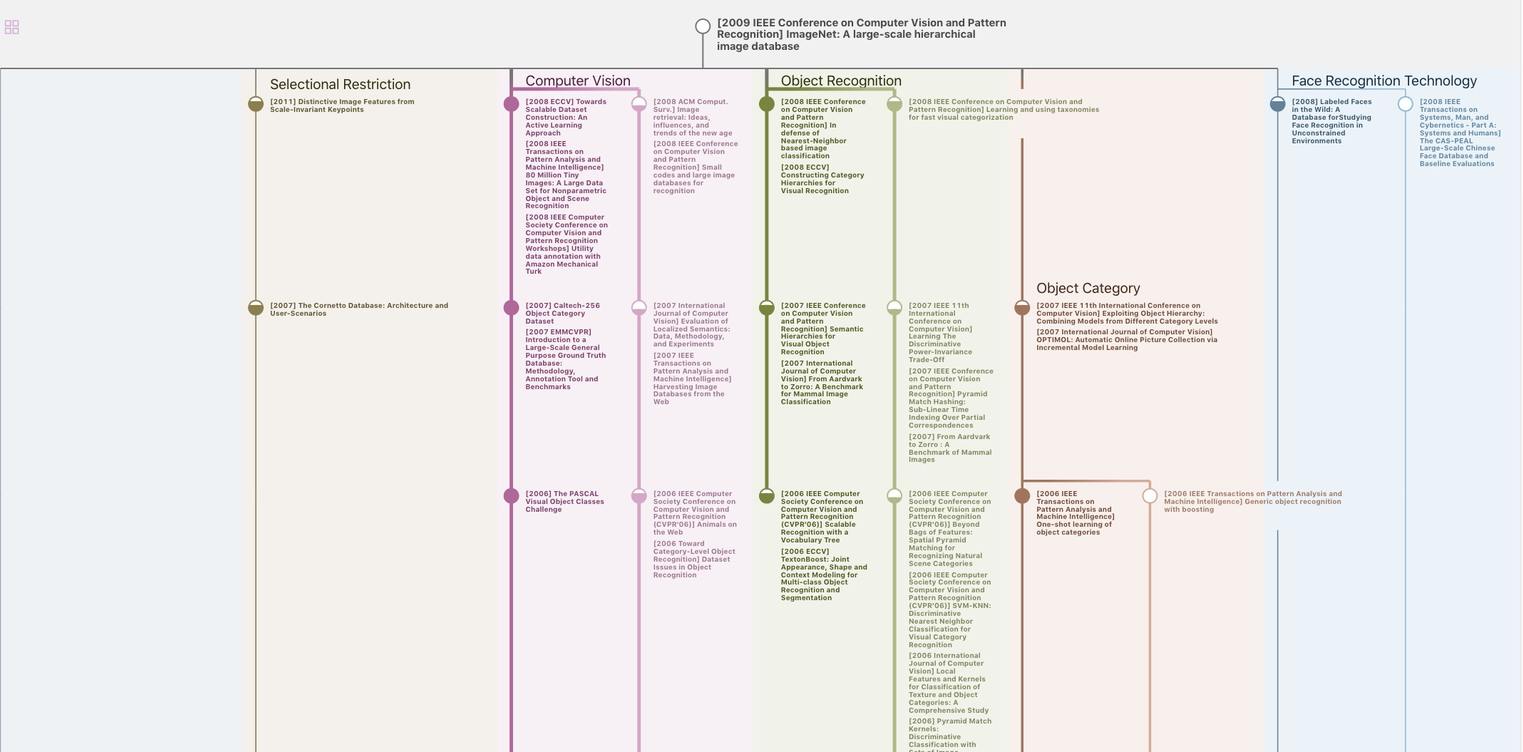
生成溯源树,研究论文发展脉络
Chat Paper
正在生成论文摘要