Automated LC-MS analysis and data extraction for high-throughput chemistry
DIGITAL DISCOVERY(2023)
摘要
High-throughput experimentation for chemistry and chemical biology has emerged as a highly impactful technology, particularly when applied to Direct-to-Biology. Analysis of the rich datasets which come from this mode of experimentation continues to be the rate-limiting step to reaction optimisation and the submission of compounds for biological assay. We present PyParse, an automated, accurate and accessible program for data extraction from high-throughput chemistry and provide real-life examples of situations in which PyParse can provide dramatic improvements in the speed and accuracy of analysing plate data. This software package has been made available through GitHub repository under an open-source Apache 2.0 licence, to facilitate the widespread adoption of high-throughput chemistry and enable the creation of standardised chemistry datasets for reaction prediction. We demonstrate that PyParse, an open-access tool, improves upon existing methods for analysis of LC-MS data obtained for high-throughput experimentation.
更多查看译文
关键词
data extraction,chemistry,high-throughput
AI 理解论文
溯源树
样例
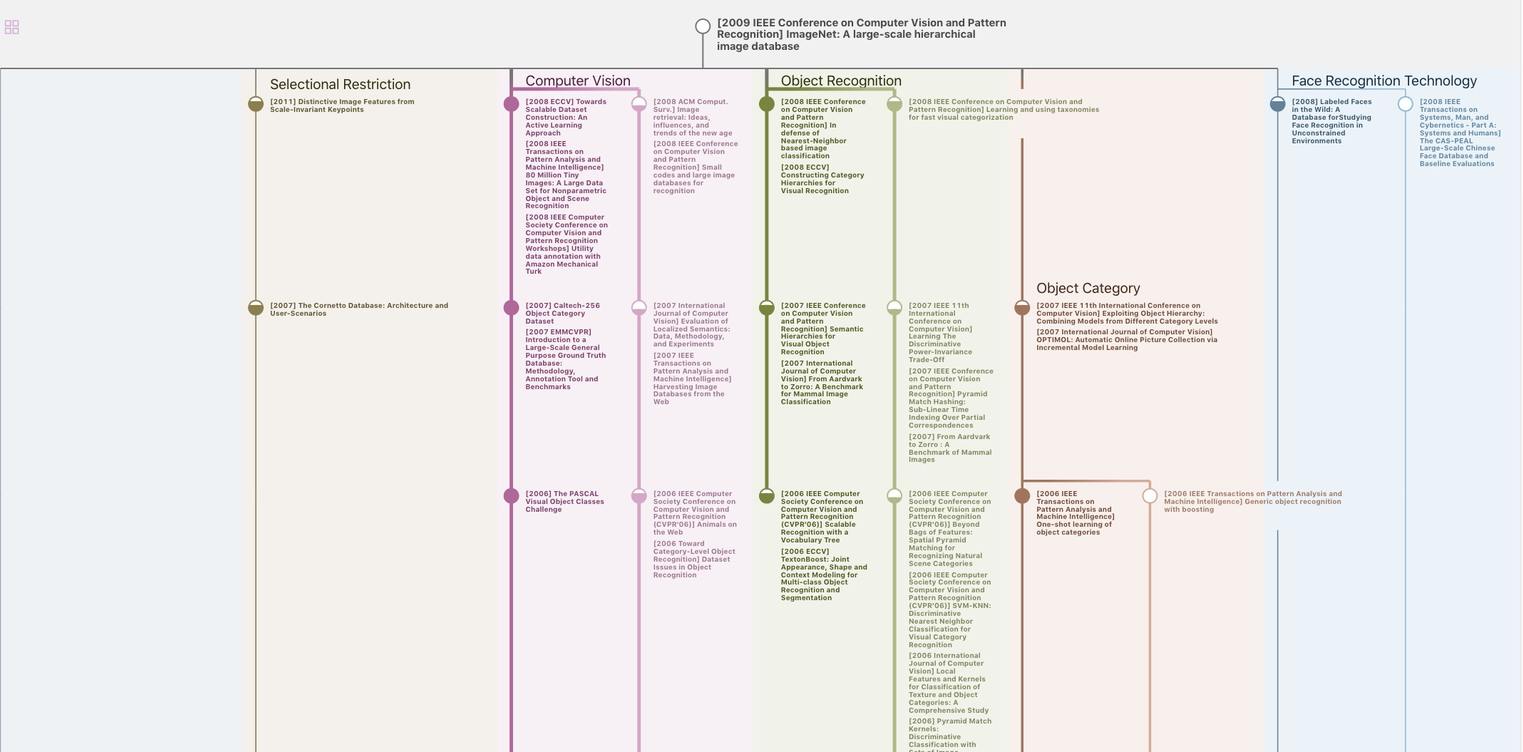
生成溯源树,研究论文发展脉络
Chat Paper
正在生成论文摘要