Optimal Transport-Based One-Shot Federated Learning for Artificial Intelligence of Things
IEEE INTERNET OF THINGS JOURNAL(2024)
摘要
Federated learning (FL) is an emerging distributed machine learning (ML) paradigm in the Artificial Intelligence of Things (AIoT). FL enables AIoT devices to collaboratively train an ML model on the network edge, while protecting data privacy and solving the problem of isolated data islands. Contemporary FL is typically realized through the model aggregation of locally trained models and the model dissemination of a globally averaged model; such a procedure iteratively proceeds until a predefined convergence criterion is met. However, FL necessitates frequent information exchanges between AIoT devices and a parameter server, which inevitably induces tremendous communication costs. Therefore, this article proposes a new design for efficient one-shot FL for AIoT systems, so that the model aggregation and dissemination can be completed within a single communication round. To this end, we leverage optimal transport (OT) theory to design the coupled model averaging (CODE) algorithm to fuse the model weights of the neural networks (NNs) on AIoT devices. The CODE algorithm initially performs OT-based layer-by-layer model averaging (MA) over two NNs to form a fused NN, which will then be averaged with another NN. The CODE algorithm progressively determines a pair of NNs, and continues until all NNs have been examined to achieve one-shot FL. In addition, we provide a detailed convergence analysis for the proposed solution. Our simulation results show that the proposed solution outperforms other one-shot MA mechanisms under various parameter settings.
更多查看译文
关键词
Artificial Intelligence of Things (AIoT),federated learning (FL),one-shot learning,optimal transport (OT)
AI 理解论文
溯源树
样例
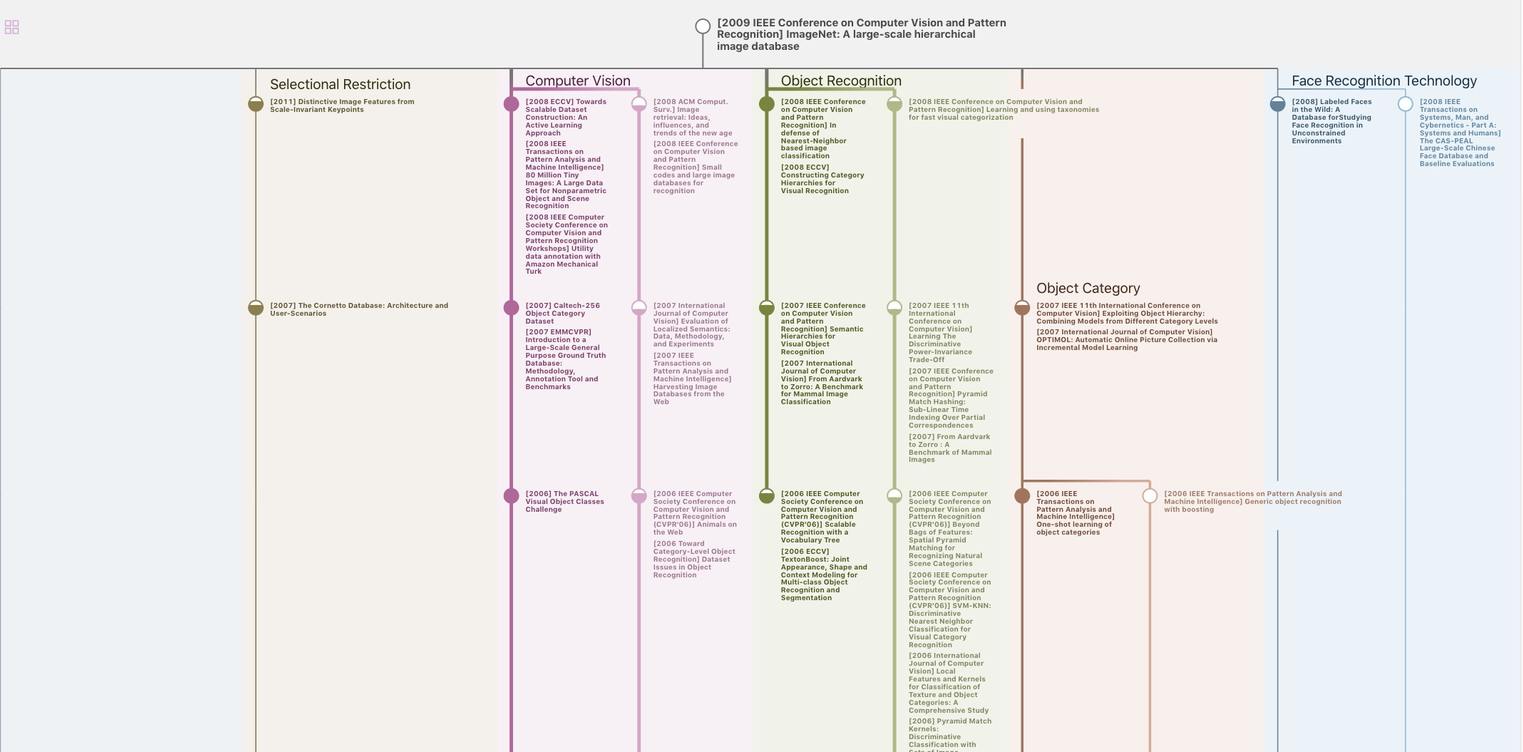
生成溯源树,研究论文发展脉络
Chat Paper
正在生成论文摘要