DSS-Net: Dynamic Self-Supervised Network for Video Anomaly Detection
IEEE TRANSACTIONS ON MULTIMEDIA(2024)
摘要
Video Anomaly detection, aiming to detect the abnormal behaviors in surveillance videos, is a challenging task since the anomalous events are diversified and complicated in different situations. And this makes it difficult to use one single static network architecture to extract useful information from diverse abnormal patterns. Therefore, in this article, we propose a novel Dynamic Self-Supervised Network (DSS-Net) to explore both spatial and temporal anomalous information. In our DSS-Net, we design a dynamic network to adaptively select suitable network architecture to extract latent features from different anomalous patterns and normal patterns. Specifically, we generate spatial and temporal pseudo-abnormal data as the input of the dynamic network to conduct self-supervised learning. And we have a specific design on Hybrid Anomaly Dynamic Convolution (HAD-Conv) to extract features for diversified anomalous events adaptively. We utilize both normal and pseudo-abnormal data to encourage the dynamic network to mine the discriminative information. Furthermore, we design a feature separation loss to maximize the difference between the anomalous and normal videos. We evaluate our proposed method on four public anomaly detection datasets and achieve competitive results compared with the state-of-the-art approaches.
更多查看译文
关键词
Anomaly detection,dynamic networks,pseudo-abnormal data,self-supervised learning
AI 理解论文
溯源树
样例
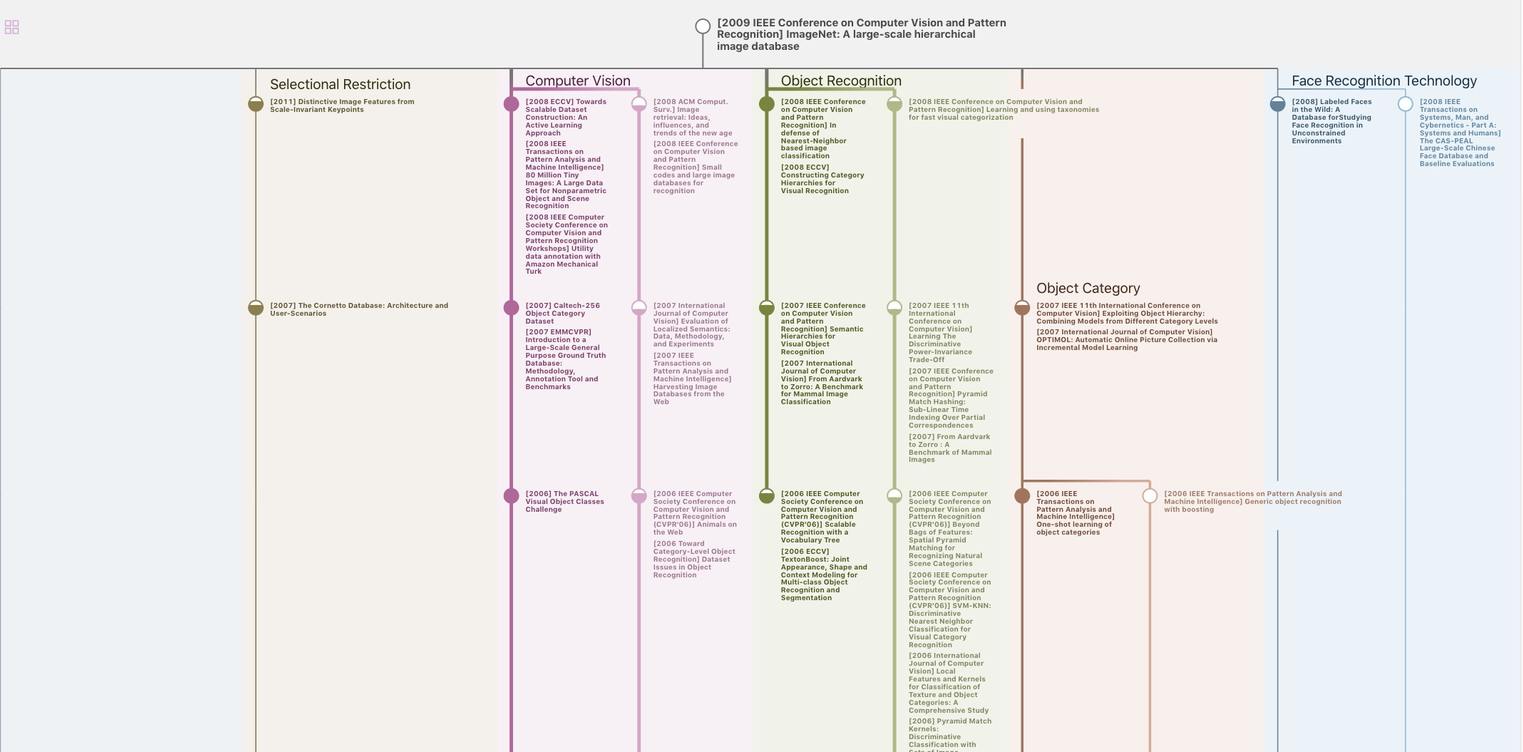
生成溯源树,研究论文发展脉络
Chat Paper
正在生成论文摘要