Instrumental Variable Estimation for Causal Inference in Longitudinal Data with Time-Dependent Latent Confounders
AAAI 2024(2024)
摘要
Causal inference from longitudinal observational data is a challenging problem due to the difficulty in correctly identifying the time-dependent confounders, especially in the presence of latent time-dependent confounders. Instrumental variable (IV) is a powerful tool for addressing the latent confounders issue, but the traditional IV technique cannot deal with latent time-dependent confounders in longitudinal studies. In this work, we propose a novel Time-dependent Instrumental Factor Model (TIFM) for time-varying causal effect estimation from data with latent time-dependent confounders. At each time-step, the proposed TIFM method employs the Recurrent Neural Network (RNN) architecture to infer latent IV, and then uses the inferred latent IV factor for addressing the confounding bias caused by the latent time-dependent confounders. We provide a theoretical analysis for the proposed TIFM method regarding causal effect estimation in longitudinal data. Extensive evaluation with synthetic datasets demonstrates the effectiveness of TIFM in addressing causal effect estimation over time. We further apply TIFM to a climate dataset to showcase the potential of the proposed method in tackling real-world problems.
更多查看译文
关键词
ML: Time-Series/Data Streams,ML: Causal Learning
AI 理解论文
溯源树
样例
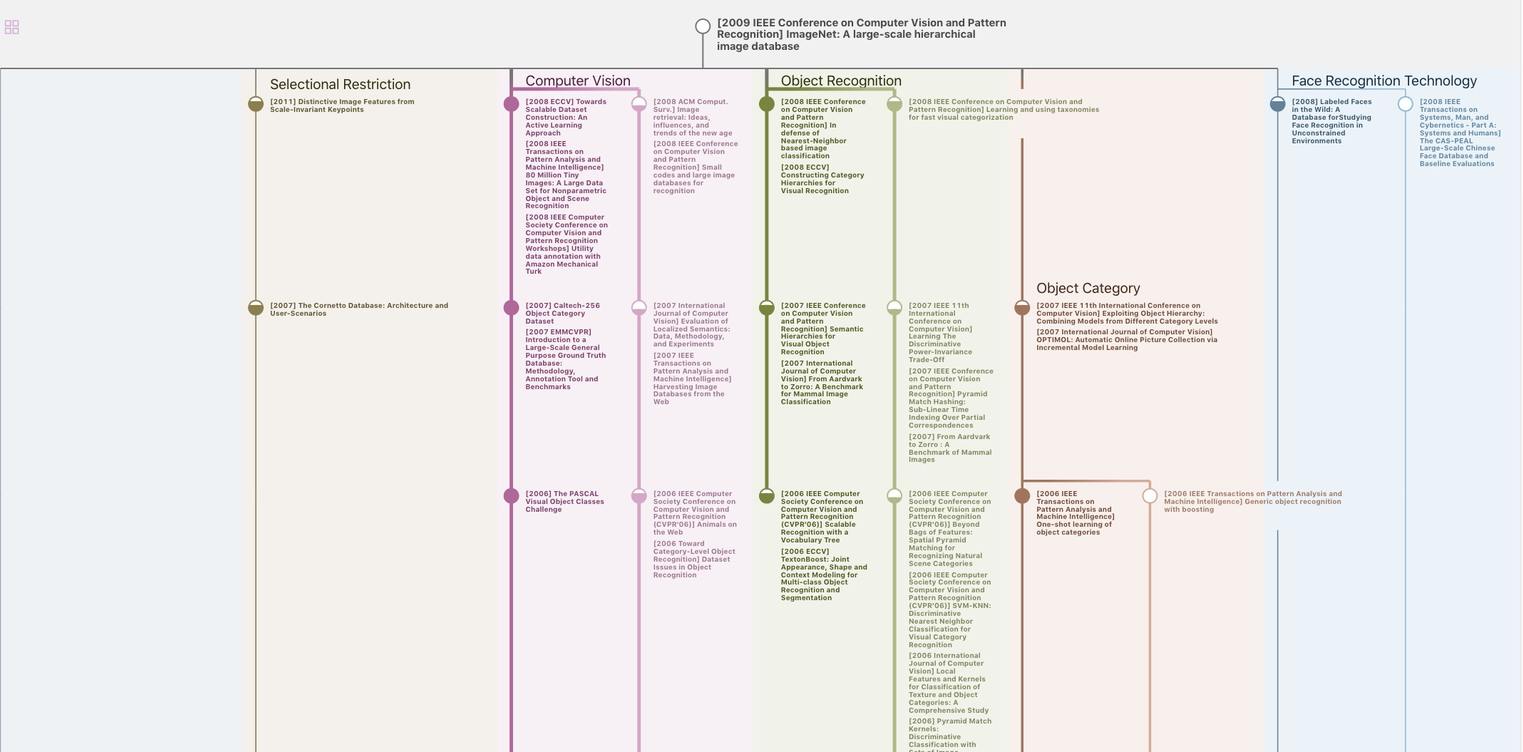
生成溯源树,研究论文发展脉络
Chat Paper
正在生成论文摘要