Polysomnographic Assessment of Sleep Disturbances in Cancer Development
Chest(2023)
摘要
Background Many cellular processes are controlled by sleep. Therefore, alterations in sleep might be expected to stress biological systems that could influence malignancy risk. Research Question What is the association between polysomnographic measures of sleep disturbances and incident cancer, and what is the validity of cluster analysis in identifying polysomnography phenotypes? Study Design and Methods We conducted a retrospective multicenter cohort study using linked clinical and provincial health administrative data on consecutive adults free of cancer at baseline with polysomnography data collected between 1994 and 2017 in four academic hospitals in Ontario, Canada. Cancer status was derived from registry records. Polysomnography phenotypes were identified by k-means cluster analysis. A combination of validation statistics and distinguishing polysomnographic features was used to select clusters. Cox cause-specific regressions were used to assess the relationship between identified clusters and incident cancer. Results Among 29,907 individuals, 2,514 (8.4%) received a diagnosis of cancer over a median of 8.0 years (interquartile range, 4.2-13.5 years). Five clusters were identified: mild (mildly abnormal polysomnography findings), poor sleep, severe OSA or sleep fragmentation, severe desaturations, and periodic limb movements of sleep (PLMS). The associations between cancer and all clusters compared with the mild cluster were significant while controlling for clinic and year of polysomnography. When additionally controlling for age and sex, the effect remained significant only for PLMS (adjusted hazard ratio [aHR], 1.26; 95% CI, 1.06-1.50) and severe desaturations (aHR, 1.32; 95% CI, 1.04-1.66). Further controlling for confounders, the effect remained significant for PLMS, but was attenuated for severe desaturations. Interpretation In a large cohort, we confirmed the importance of polysomnographic phenotypes and highlighted the role that PLMS and oxygenation desaturation may play in cancer. Using this study’s findings, we also developed an Excel (Microsoft) spreadsheet (polysomnography cluster classifier) that can be used to validate the identified clusters on new data or to identify which cluster a patient belongs to. Trial Registry ClinicalTrials.gov; Nos.: NCT03383354 and NCT03834792; URL: www.clinicaltrials.gov Many cellular processes are controlled by sleep. Therefore, alterations in sleep might be expected to stress biological systems that could influence malignancy risk. What is the association between polysomnographic measures of sleep disturbances and incident cancer, and what is the validity of cluster analysis in identifying polysomnography phenotypes? We conducted a retrospective multicenter cohort study using linked clinical and provincial health administrative data on consecutive adults free of cancer at baseline with polysomnography data collected between 1994 and 2017 in four academic hospitals in Ontario, Canada. Cancer status was derived from registry records. Polysomnography phenotypes were identified by k-means cluster analysis. A combination of validation statistics and distinguishing polysomnographic features was used to select clusters. Cox cause-specific regressions were used to assess the relationship between identified clusters and incident cancer. Among 29,907 individuals, 2,514 (8.4%) received a diagnosis of cancer over a median of 8.0 years (interquartile range, 4.2-13.5 years). Five clusters were identified: mild (mildly abnormal polysomnography findings), poor sleep, severe OSA or sleep fragmentation, severe desaturations, and periodic limb movements of sleep (PLMS). The associations between cancer and all clusters compared with the mild cluster were significant while controlling for clinic and year of polysomnography. When additionally controlling for age and sex, the effect remained significant only for PLMS (adjusted hazard ratio [aHR], 1.26; 95% CI, 1.06-1.50) and severe desaturations (aHR, 1.32; 95% CI, 1.04-1.66). Further controlling for confounders, the effect remained significant for PLMS, but was attenuated for severe desaturations. In a large cohort, we confirmed the importance of polysomnographic phenotypes and highlighted the role that PLMS and oxygenation desaturation may play in cancer. Using this study’s findings, we also developed an Excel (Microsoft) spreadsheet (polysomnography cluster classifier) that can be used to validate the identified clusters on new data or to identify which cluster a patient belongs to. ClinicalTrials.gov; Nos.: NCT03383354 and NCT03834792; URL: www.clinicaltrials.gov Polysomnographic Subtypes and Incident Cancer: Another Adverse Outcome Related to Period Limb MovementsCHESTVol. 164Issue 2PreviewFor several years, there has been an increased focus in sleep research on identifying patient subtypes defined by characteristics ranging from self-reported symptoms to objective data from polysomnography (PSG) and associating these subtypes with clinically important outcomes.1-5 In this issue of CHEST, Kendzerska et al6 use a large retrospective dataset and routinely available information from PSG to validate previously suggested physiologic subtypes and to examine relationships with cancer incidence. Full-Text PDF
更多查看译文
关键词
sleep disturbances,cancer
AI 理解论文
溯源树
样例
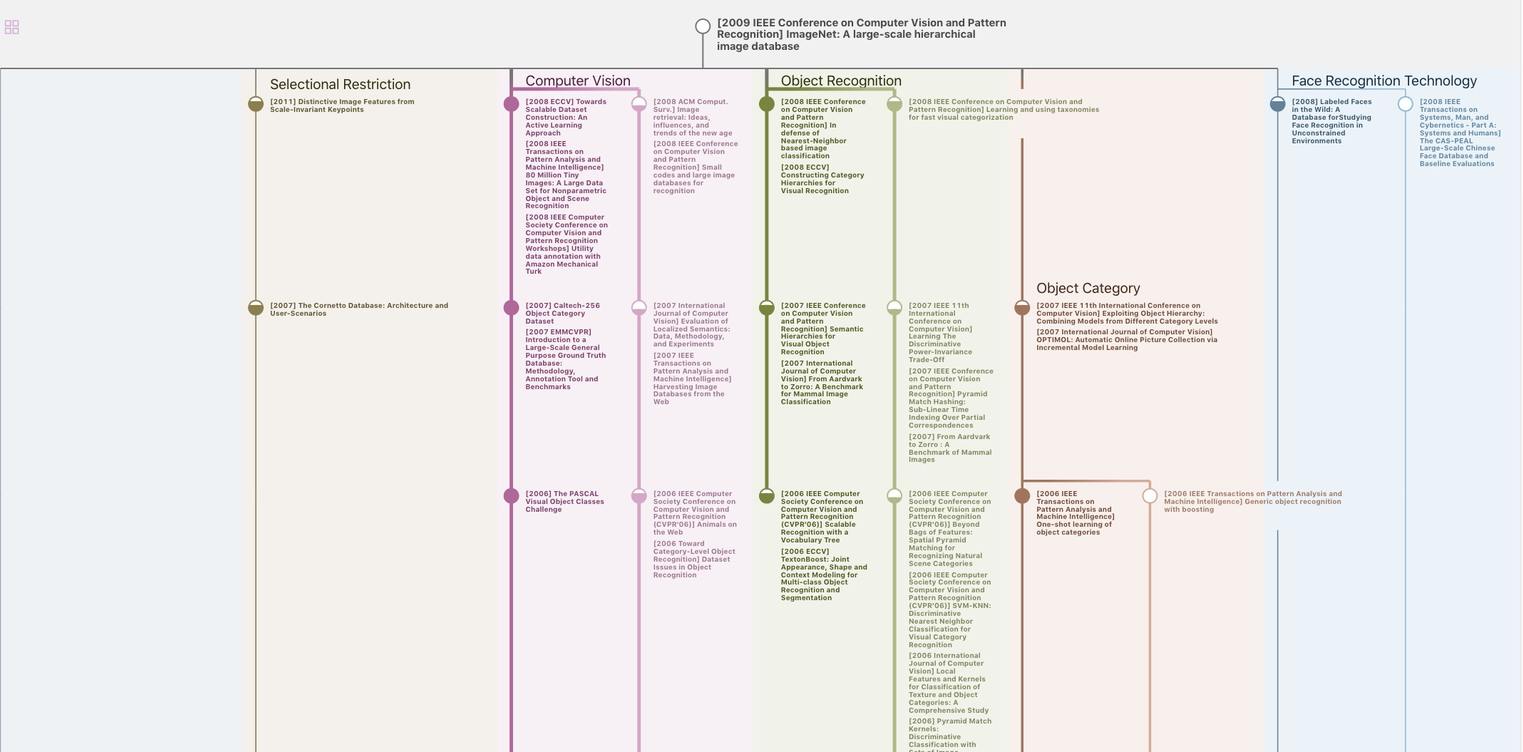
生成溯源树,研究论文发展脉络
Chat Paper
正在生成论文摘要