Language-Guided Transformer for Federated Multi-Label Classification
AAAI 2024(2024)
摘要
Federated Learning (FL) is an emerging paradigm that enables multiple users to collaboratively train a robust model in a privacy-preserving manner without sharing their private data. Most existing approaches of FL only consider traditional single-label image classification, ignoring the impact when transferring the task to multi-label image classification. Nevertheless, it is still challenging for FL to deal with user heterogeneity in their local data distribution in the real-world FL scenario, and this issue becomes even more severe in multi-label image classification. Inspired by the recent success of Transformers in centralized settings, we propose a novel FL framework for multi-label classification. Since partial label correlation may be observed by local clients during training, direct aggregation of locally updated models would not produce satisfactory performances. Thus, we propose a novel FL framework of Language-Guided Transformer (FedLGT) to tackle this challenging task, which aims to exploit and transfer knowledge across different clients for learning a robust global model. Through extensive experiments on various multi-label datasets (e.g., FLAIR, MS-COCO, etc.), we show that our FedLGT is able to achieve satisfactory performance and outperforms standard FL techniques under multi-label FL scenarios. Code is available at https://github.com/Jack24658735/FedLGT.
更多查看译文
关键词
ML: Distributed Machine Learning & Federated Learning,CV: Language and Vision,ML: Multi-class/Multi-label Learning & Extreme Classification
AI 理解论文
溯源树
样例
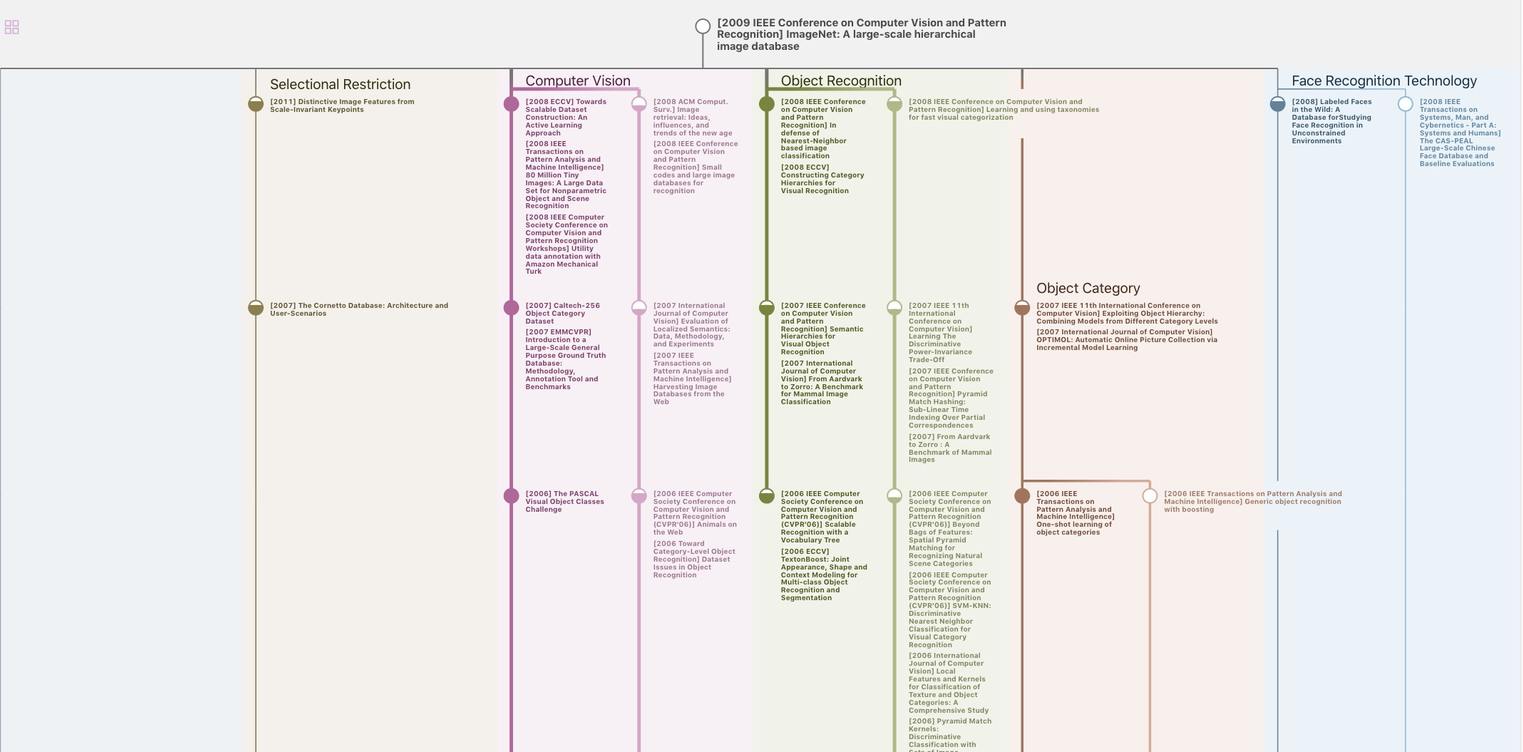
生成溯源树,研究论文发展脉络
Chat Paper
正在生成论文摘要