One-Stage Methods of Computer Vision Object Detection to Classify Carious Lesions from Smartphone Imaging
Oral(2023)
摘要
The current study aimed to implement and validate an automation system to detect carious lesions from smartphone images using different one-stage deep learning techniques. 233 images of carious lesions were captured using a smartphone camera system at 1432 × 1375 pixels, then classified and screened according to a visual caries classification index. Following data augmentation, the YOLO v5 model for object detection was used. After training the model with 1452 images at 640 × 588 pixel resolution, which included the ones that were created via image augmentation, a discrimination experiment was performed. Diagnostic indicators such as true positive, true negative, false positive, false negative, and mean average precision were used to analyze object detection performance and segmentation of systems. YOLO v5X and YOLO v5M models achieved superior performance over the other models on the same dataset. YOLO v5X’s mAP was 0.727, precision was 0.731, and recall was 0.729, which was higher than other models of YOLO v5, which generated 64% accuracy, with YOLO v5M producing slightly inferior results. Overall mAPs of 0.70, precision of 0.712, and recall of 0.708 were achieved. Object detection through the current YOLO models was able to successfully extract and classify regions of carious lesions from smartphone photographs of in vitro tooth specimens with reasonable accuracy. YOLO v5M was better fit to detect carious microcavitations while YOLO v5X was able to detect carious changes without cavitation. No single model was capable of adequately diagnosing all classifications of carious lesions.
更多查看译文
关键词
caries,smartphone,clinical photography,YOLO,object detection
AI 理解论文
溯源树
样例
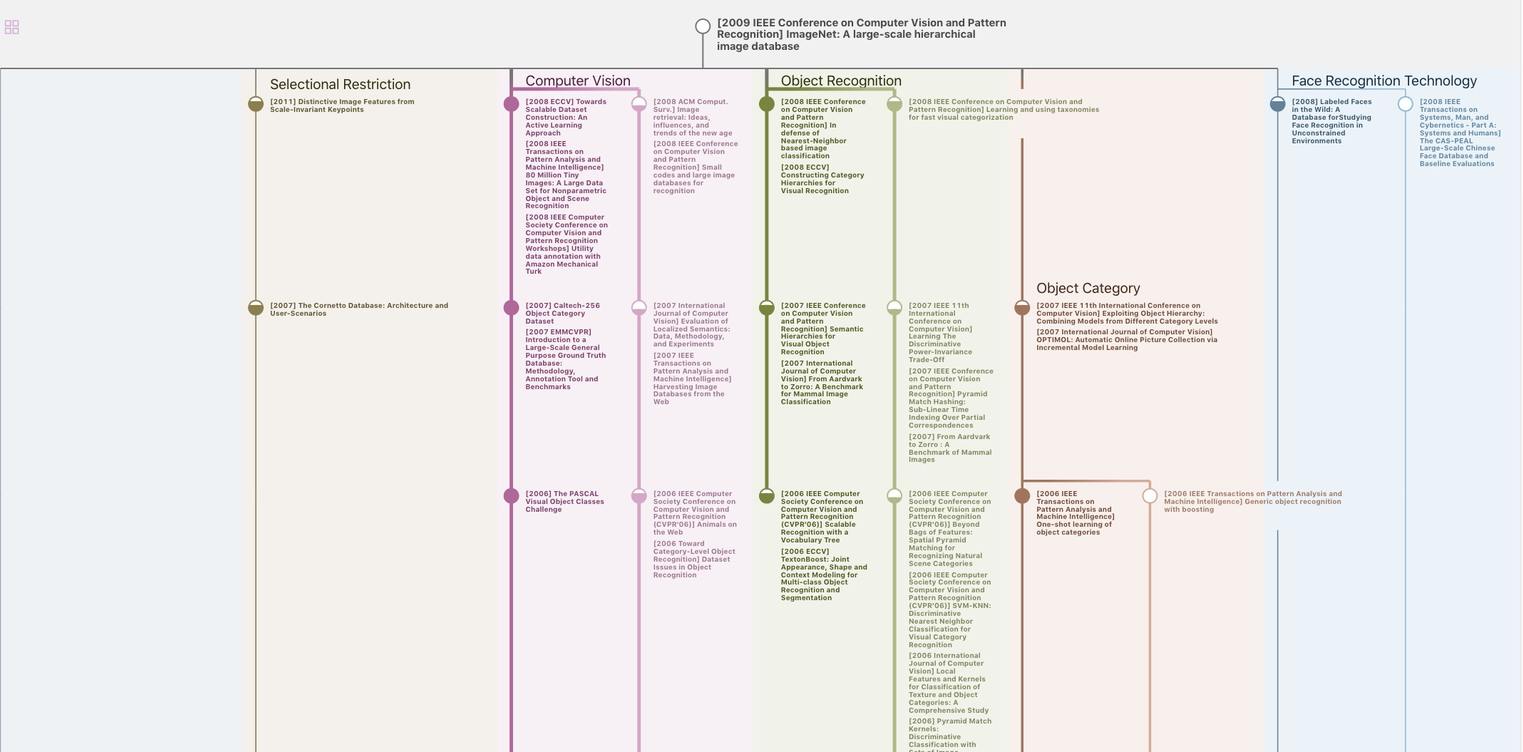
生成溯源树,研究论文发展脉络
Chat Paper
正在生成论文摘要