Knowledge-informed neuro-integrators for aggregation kinetics
COMMUNICATIONS IN NONLINEAR SCIENCE AND NUMERICAL SIMULATION(2024)
摘要
We report a novel approach for the efficient computation of solutions of a broad class of largescale systems of non-linear ordinary differential equations, describing aggregation kinetics. The method is based on a new take on the dimensionality reduction for this class of equations which can be naturally implemented by a cascade of small feed -forward artificial neural networks. We show that this cascade, of otherwise static models, is capable of predicting solutions of the original large-scale system over large intervals of time, using the information about the solution computed over much smaller intervals. The computational cost of the method depends very mildly on the temporal horizon, which is a major improvement over the current state-of-the-art methods, whose complexity increases super -linearly with the system's size and proportionally to the simulation time. In cases when prior information about the values of solutions over a relatively small interval of time is already available, the method's computational complexity does not depend explicitly on the system's size. The successful application of the new method is illustrated for spatially -homogeneous systems, with a source of monomers, for a number of the most representative reaction rates kernels.
更多查看译文
关键词
Aggregation kinetics,Model reduction,Neural networks,Machine leaning
AI 理解论文
溯源树
样例
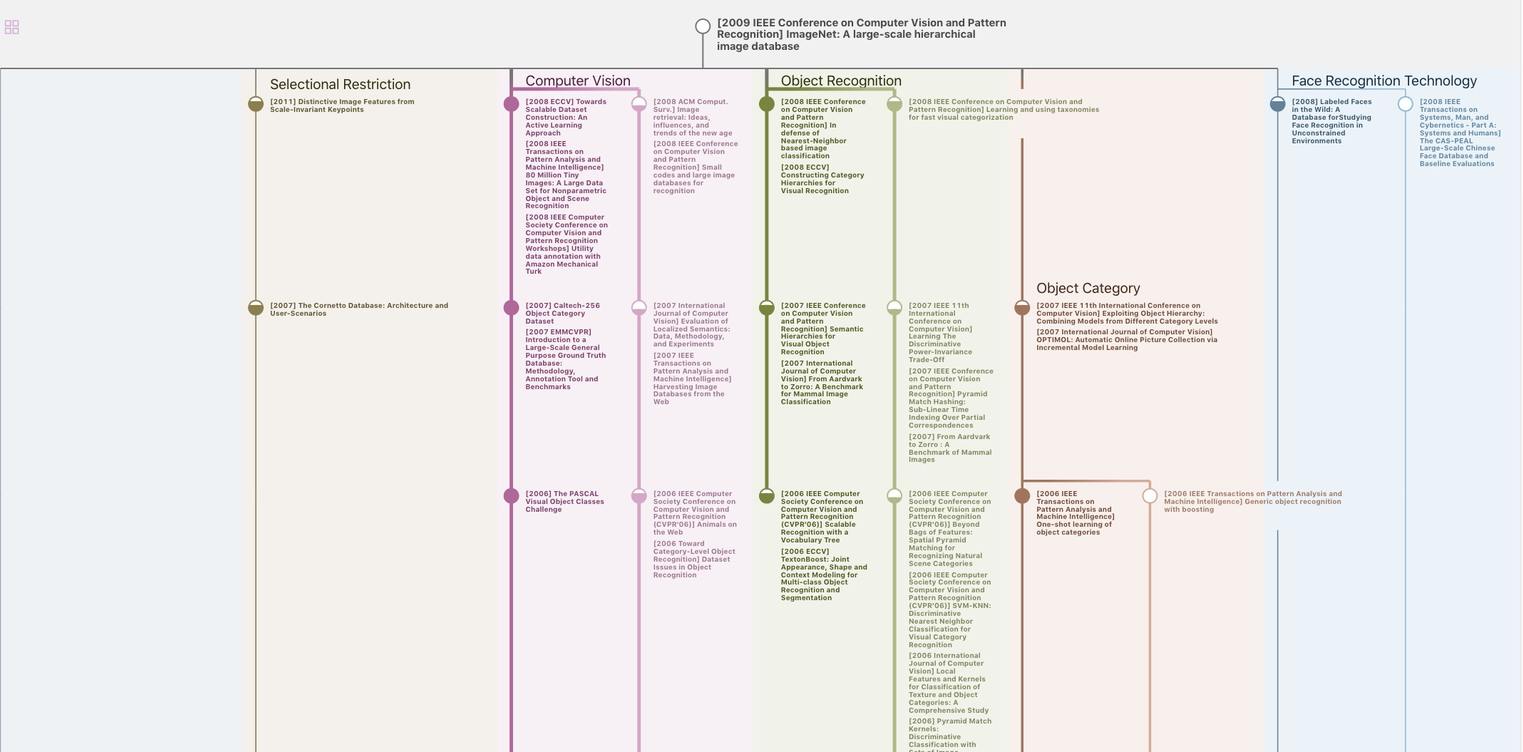
生成溯源树,研究论文发展脉络
Chat Paper
正在生成论文摘要