Estimating Counterfactual Matrix Means with Short Panel Data
arxiv(2023)
摘要
We develop a more flexible approach for identifying and estimating average
counterfactual outcomes when several but not all possible outcomes are observed
for each unit in a large cross section. Such settings include event studies and
studies of outcomes of "matches" between agents of two types, e.g. workers and
firms or people and places. When outcomes are generated by a factor model that
allows for low-dimensional unobserved confounders, our method yields
consistent, asymptotically normal estimates of counterfactual outcome means
under asymptotics that fix the number of outcomes as the cross section grows
and general outcome missingness patterns, including those not accommodated by
existing methods. Our method is also computationally efficient, requiring only
a single eigendecomposition of a particular aggregation of any factor estimates
constructed using subsets of units with the same observed outcomes. In a
semi-synthetic simulation study based on matched employer-employee data, our
method performs favorably compared to a Two-Way-Fixed-Effects-model-based
estimator.
更多查看译文
AI 理解论文
溯源树
样例
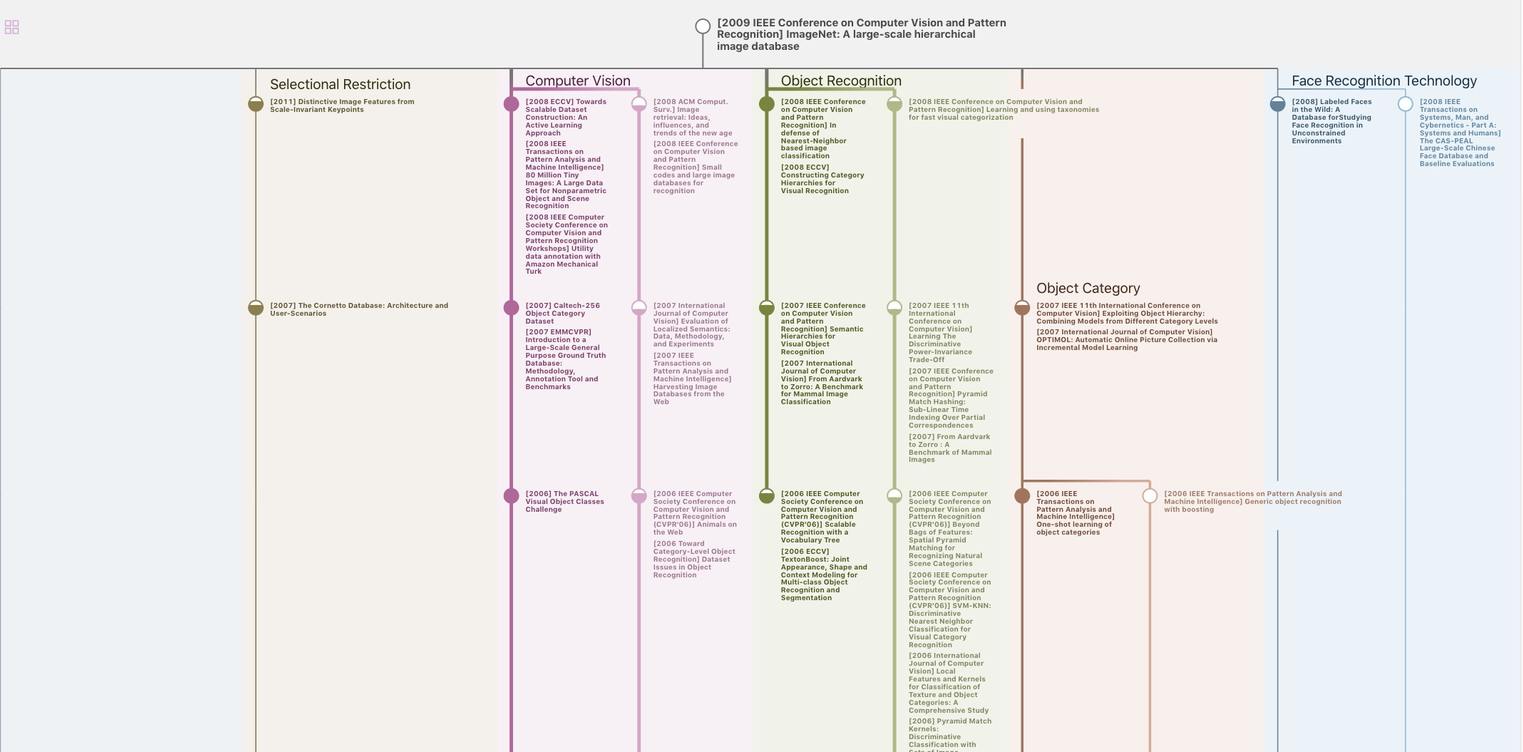
生成溯源树,研究论文发展脉络
Chat Paper
正在生成论文摘要